Artificial intelligence (AI) is used to describe the creation of smart machines and computer systems capable of doing work previously reserved for humans. Machine learning, NLP, CV, and robots are just a few of the many technologies included. Healthcare, banking, transportation, education, and entertainment are just a few of the many fields that benefits from artificial intelligence. Autonomous vehicles, medical diagnosis, virtual assistants, recommendation systems, fraud detection, and more are just a few examples of how AI is changing the world. It revolutionizes people’s daily lives and workplaces by fostering productivity, creativity, and fresh opportunities.
Artificial intelligence (AI) is changing entire industries and opening up previously unimaginable opportunities. Autonomous vehicles, voice-activated assistants, tailored suggestions, and prescriptive analytics are just some of the technologies made possible by such a breakthrough. Artificial intelligence (AI)-enabled systems are revolutionizing efficiency, productivity, and accuracy by processing massive volumes of data, identifying patterns, and making complicated choices in real-time.
AI raises important moral questions. Privacy, security, bias, and the economy and workforce are all raised as concerns despite this promising promise. Finding a happy medium between exploration and accountability is essential. AI has many advantages, however, some problems still occur, such as poor data quality, a lack of interpretability, and the requirement for constant learning and adaptability. Overcoming these hurdles and addressing ethical considerations is crucial to ensuring AI’s responsible and positive incorporation into society.
1. Virtual agent
A virtual agent, commonly known as a chatbot or conversational agent, refers to a computer program or AI system designed to simulate human-like conversations and interact with users in natural language. These virtual agents are typically deployed on various platforms, such as websites, messaging applications, or virtual assistants, to provide automated customer support, answer user inquiries, perform tasks, or offer personalized recommendations.
The latest news surrounding virtual agents showcases their increasing adoption and advancements in their capabilities. One notable trend is the integration of virtual agents into customer service and support channels. Many businesses are leveraging virtual agents to manage routine customer inquiries, provide instant responses, and offer 24/7 support. Companies improve efficiency, reduce response times, and enhance customer satisfaction by automating these interactions.
Advancements in natural language processing and machine learning techniques have contributed to the improved intelligence and conversational capabilities of virtual agents. These agents now understand and interpret complex user queries, recognize intent, and provide more accurate and relevant responses. They even learn from past interactions and user feedback to continuously enhance their performance.
Another significant development in virtual agents is their integration with voice-based assistants or smart home devices. Virtual agents are now accessed through voice commands, allowing users to interact with them hands-free and receive instant assistance. Such an integration further expands the convenience and accessibility of virtual agents, enabling users to access information or perform tasks through voice interactions.
Virtual agents are being employed in sectors beyond customer support. They are finding applications in fields like healthcare, education, and finance. Virtual agents in the healthcare industry assist with patient triage, provide health-related information, or offer support for mental health. Virtual agents serve as tutors or provide personalized learning experiences in education. They assist with financial planning or offer investment advice in finance.
The ongoing advancements in virtual agent technologies aim to enhance their natural language understanding, context awareness, and emotional intelligence. Researchers are exploring techniques like sentiment analysis and emotional recognition to enable virtual agents to understand and respond appropriately to user emotions and provide more empathetic interactions.
2. Biometrics
The field of study known as biometrics focuses on developing methods for accurately and reliably determining and classifying individuals based on their distinct physical or behavioral traits. Biometric systems enable the identification and authentication of individuals through traits such as fingerprints, facial features, iris patterns, voiceprints, and even behavioral attributes like typing rhythm, or motion by leveraging cutting-edge technology. Biometric systems offer superior security and convenience, as they capture and analyze these distinct traits to verify or recognize individuals with greater accuracy compared to traditional identification methods such as passwords or ID cards.
The latest advancements in biometrics highlight their remarkable potential and wide-ranging applications. A notable trend is the integration of biometric technology into mobile devices, enabling secure authentication. Many smartphones now incorporate fingerprint sensors or facial recognition capabilities, empowering users to unlock their devices or authorize transactions using their unique biometric traits. The widespread adoption emphasizes the increasing significance of biometrics in enhancing security and the user experience.
Biometrics are making notable contributions to border control and airport security. Biometric systems are being deployed at airports and border checkpoints to verify the identities of travelers and strengthen security measures. Automated facial recognition and iris scanning technologies enable faster and more accurate identity verification, facilitating the prevention of identity fraud and smoother travel processes.
Financial services, medical care, and security are just some of the fields that benefit from biometrics. Implementing biometric authentication technologies in the financial sector helps reduce the chances of fraud and illegal access to online banking and payment systems. Accurate patient identification, protected access to medical records, and the elimination of medical identity theft are all made possible by biometrics in the healthcare industry. Biometric systems are used by law enforcement to aid in the identification and arrest of criminals by matching biometric data, such as fingerprints or facial features, with databases of known individuals.
The continuous advancements in biometric technologies focus on improving accuracy, robustness, and usability. Innovations such as contactless biometrics, multimodal biometrics (combining multiple traits), and liveness detection (to prevent spoofing) are actively being researched and developed to address security concerns and enhance the reliability of biometric systems.
3. Computer Vision
Computer vision refers to the field of artificial intelligence and computer science that focuses on enabling computers to gain a high-level understanding of visual information from images or videos. It involves developing algorithms and systems that interpret and analyze visual data to extract meaningful insights, recognize objects, detect patterns, and make decisions based on visual inputs. Computer vision algorithms aim to replicate human visual perception and enable machines to “see” and understand the visual world.
The latest news in computer vision showcases several exciting advancements and applications. One prominent trend is the use of computer vision in autonomous vehicles and robotics. Computer vision algorithms play a crucial role in enabling vehicles and robots to perceive and interpret their surroundings, detect obstacles, recognize traffic signs, and navigate autonomously. The technology has the potential to revolutionize transportation systems, enhance safety, and enable the deployment of self-driving cars and advanced robotic systems.
Another noteworthy development in computer vision is its application in healthcare. Computer vision algorithms analyze medical images, such as X-rays, CT scans, and pathology slides, to assist in disease diagnosis, tumor detection, and medical imaging analysis. Such technology has the potential to improve the accuracy and efficiency of diagnostics, aid in the early detection of diseases, and enhance patient care in the healthcare industry.
Computer vision is being utilized in various fields, such as retail, agriculture, security, and entertainment. In retail, computer vision systems enable cashier-less checkout, automated inventory management, and personalized shopping experiences. In agriculture, computer vision assists in crop monitoring, pest detection, and yield estimation. In security, computer vision algorithms enhance surveillance systems, facial recognition, and object tracking. Computer vision is used for virtual reality, augmented reality, and gesture recognition, enhancing user experiences in gaming and multimedia applications in entertainment.
The continuous advancements in deep learning, neural networks, and hardware technologies have significantly contributed to the progress in computer vision. These advancements have led to improved accuracy, speed, and scalability of computer vision algorithms, enabling their widespread adoption and opening up new possibilities for applications across various industries.
4. AI in Healthcare
The application of artificial intelligence technologies and algorithms in the healthcare business to improve patient care, diagnostics, treatment planning, and overall healthcare administration is referred to as AI in healthcare. It entails the use of machine learning, deep learning, natural language processing, and computer vision on enormous volumes of medical data to discover patterns, generate predictions, and aid healthcare professionals in decision-making processes.
The most recent AI news in healthcare highlights a number of key achievements and efforts. The application of AI for medical imaging analysis is a key trend. Medical pictures such as X-rays, MRIs, and CT scans are analyzed by AI algorithms, which aid radiologists in detecting abnormalities and offering correct diagnoses. Such a type of technology has the potential to increase illness diagnosis, eliminate diagnostic errors, and improve patient outcomes.
Another major advancement is the use of AI in medication research and development. AI systems scan massive volumes of biomedical data, including genomic information and chemical structures, to find prospective medication candidates and accelerate the drug development process. It offers the potential to speed up the discovery of new medicines, improve personalized care, and more effectively address unmet medical needs.
AI is revolutionizing healthcare by creating virtual assistants and chatbots that offer patient support, answer health-related questions, and deliver critical medical advice. These artificial intelligence-powered technologies improve access to healthcare information, enable remote patient monitoring, and boost patient participation and self-management.
AI is being used to forecast disease outcomes and aid in clinical decision-making. Machine learning models trained on patient data aid in disease progression prediction, identifying high-risk patients, and recommending effective treatment regimens. The technology has the potential to provide more tailored and accurate healthcare interventions, which could result in better patient outcomes and resource management.
The use of AI in healthcare creates ethical, privacy, and security problems. Ensuring the responsible and ethical use of patient data, safeguarding data privacy, and correcting biases in AI systems are critical considerations in such a domain.
5. AI in Education
The utilization of artificial intelligence (AI) in the field of education encompasses the application of advanced technologies and analytical techniques to elevate and revolutionize the learning process. The process involves the integration of intelligent systems, sophisticated algorithms, and data analysis to facilitate teaching, personalized learning, assessment, and educational administration. The primary objective of AI in education is to enhance educational outcomes, improve efficiency, and provide learners with tailored and adaptive learning experiences that cater to their unique needs.
The latest developments in AI for education are both promising and captivating. One notable trend is the incorporation of AI-powered virtual assistants and chatbots within educational platforms. These intelligent companions serve as valuable resources for students by offering prompt answers to inquiries, delivering timely feedback, and providing personalized recommendations. These virtual assistants foster increased student engagement, provide immediate support, and enable educators to identify areas where students require additional guidance or intervention by acting as interactive and accessible aids.
The integration of machine learning algorithms has paved the way for personalized learning paths based on individual student data analysis. Adaptive learning systems, leveraging AI capabilities, dynamically adjust instructional content and strategies to suit each student’s unique requirements, optimizing their learning journey by addressing their specific strengths and weaknesses. Its adaptive approach ensures that students receive tailored instruction and support, thereby maximizing their educational growth and progress.
AI is being utilized to automate administrative tasks, such as grading and scheduling, freeing up teachers’ time to focus on instruction and student support. Natural language processing and speech recognition technologies are being employed to improve language learning and assist students with reading and writing skills.
The incorporation of AI in education is prompting discussions around ethics, privacy, and the responsible use of data. Educational institutions and policymakers are addressing these concerns to ensure the ethical deployment of AI technologies while safeguarding student privacy.
Overall, AI in education holds great promise to revolutionize traditional teaching approaches, personalize learning experiences, and improve educational outcomes. It empowers educators with valuable insights, enables personalized and adaptive learning experiences for students, and streamlines administrative processes, ultimately reshaping the future of education.
6. Reinforcement Learning
Reinforcement learning is a scope of machine learning in which agents learn to create sequential decisions by interacting with their surroundings. Reinforcement learning is established on the notion that an agent receives feedback in the form of rewards or penalties for its actions, unlike supervised learning, which relies on labeled examples, and unsupervised learning, which focuses on finding patterns in data. The agent explores the environment, takes action, and learns from the consequences to maximize its cumulative reward over time.
The latest news in reinforcement learning highlights remarkable advancements and applications of such a technique. One notable development is the use of reinforcement learning in the field of robotics, which enables robots to learn complex tasks through trial and error. It has led to advancements in areas such as autonomous navigation, object manipulation, and robot-human collaboration.
Reinforcement learning has gained attention in the field of healthcare, where it is being explored to optimize treatment strategies and personalize patient care. Researchers are continually refining algorithms and techniques in reinforcement learning, such as deep reinforcement learning, which combines deep neural networks with reinforcement learning to handle complex tasks and large state spaces more efficiently.
The integration of reinforcement learning with other areas, such as natural language processing and computer vision, is an area of ongoing research, unlocking new possibilities for intelligent systems and autonomous agents. Reinforcement learning continues to push the boundaries of AI and holds great potential for solving complex decision-making problems in numerous domains.
7. Explainable AI
Explainable AI, commonly known as interpretable AI or transparent AI, encompasses the advancement of artificial intelligence systems that aim to provide comprehensive and comprehensible explanations for their decision-making processes and outputs. It tries to bridge the “black box” nature of complicated AI models and the need for transparency and confidence in AI-driven systems.
The goal of explainable AI is to provide insights into how AI algorithms arrive at their conclusions, enabling humans to understand the underlying reasoning and factors influencing the decisions. It is particularly crucial in critical domains where the stakes are high, such as healthcare, finance, and autonomous vehicles, where decisions need to be explainable and accountable.
The latest trends in explainable AI involve the development of techniques and methodologies to enhance the interpretability of AI models. Researchers are exploring methods such as rule-based explanations, model distillation, feature importance analysis, and attention mechanisms to provide intuitive explanations for AI decisions. There is even a focus on integrating human-centered design principles into explainable AI systems, ensuring that explanations are not only accurate but easily understandable and useful for end-users.
Efforts are being made to establish ethical guidelines and regulatory frameworks that promote the responsible use of AI and ensure transparency in decision-making processes. The advancement of explainable AI is aimed at fostering trust, accountability, and ethical considerations in the deployment and adoption of AI systems across various domains.
8. Transfer Learning
Transfer learning refers to a machine learning technique in which the results of one task are used to enhance the results of a related task. Transfer learning uses pre-trained models that have been built on large datasets for a separate but related job instead of beginning from scratch. The technique allows learned characteristics, representations, and patterns to be transferred from the source job to the target task, eliminating the need for vast amounts of data and computing resources.
Transfer learning is widely used in various domains, such as computer vision and natural language processing, where pre-trained models like convolutional neural networks (CNNs) and transformers are commonly employed. These models capture general features and knowledge from the source task, which is fine-tuned and adapted to the target task with relatively few labeled examples. Transfer learning facilitates faster training, better generalization, and improved performance on the target task.
The most recent trends in transfer learning emphasize expanding its capabilities and applicability. Researchers are investigating approaches for transferring knowledge across domains that enable models to transfer information from one domain to another with diverse data distributions. There is current research on unsupervised transfer learning, which tries to use unlabeled data from the source task to improve performance on the target task.
Transfer learning is being extended to more complicated tasks requiring multimodal input, such as mixing vision and language, to enable models to learn from several modalities simultaneously. One example of an extension is the combination of image recognition with natural language processing. These developments in transfer learning approaches are broadening their potential and enabling the construction of machine learning models that are more efficient and accurate in a variety of applications.
9. AI-assisted creativity
AI-assisted creativity refers to the application of artificial intelligence (AI) techniques to enhance and augment human creativity in various domains such as art, music, design, and writing. It involves the use of machine learning algorithms and generative models to assist and inspire creative processes, allowing individuals to explore new ideas, generate novel content, and push the boundaries of traditional creativity. AI-assisted creativity systems analyze and learn from vast amounts of existing creative work, enabling them to generate new and unique outputs based on learned patterns and styles.
The goal of AI-assisted creativity is not to replace human creativity but to act as a collaborator, providing suggestions, insights, and novel perspectives that spark inspiration and enhance the creative process. AI models generate artwork, compose music, design graphics, and even write text in a way that aligns with human preferences and styles. These AI systems serve as creative companions, helping artists, musicians, and designers explore new possibilities, overcome creative blocks, and discover innovative solutions.
The latest trends in AI-assisted creativity include the development of advanced generative models such as generative adversarial networks (GANs), variational autoencoders (VAEs), and transformer models. These models have shown impressive capabilities in generating highly realistic and creative outputs, pushing the boundaries of what AI is able to achieve in the realm of creativity.
There is a growing focus on interactive and co-creative AI systems that allow users to actively collaborate and interact with AI models to co-create artworks, music compositions, or designs. Additionally, efforts are being made to incorporate ethical considerations, such as diversity, fairness, and responsible use, into AI-assisted creativity systems to ensure that they contribute positively to the creative process and respect human values.
10. Machine learning
Machine learning is a subfield of AI that seeks to automate the process of learning and decision-making by computers. It is accomplished through the creation of algorithms and statistical models. It entails building mathematical models and analyzing massive datasets to spot trends, draw conclusions, and arrive at sound judgments and conclusions. Algorithms that use machine learning enhance their efficiency with each new round of training by applying what they’ve learned.
Machine learning’s primary focus is on creating computer systems that are capable of learning new skills and knowledge without human intervention. Different types of machine learning algorithms, such as those for supervised learning, unsupervised learning, and reinforcement learning, are best suited for specific tasks.
The forefront of machine learning is currently witnessing a surge of interest and progress in the realm of deep learning, a subfield that centers on the application of artificial neural networks. These deep learning models possess the ability to learn intricate hierarchical representations of data, leading to notable advancements in domains including computer vision, natural language processing, and speech recognition. Deep learning has led to breakthroughs in a wide range of tasks by using advanced methods like convolutional neural networks (CNNs) and recurrent neural networks (RNNs). These include accurate image classification, strong object detection, smooth language translation, and nuanced sentiment analysis. The boundaries of what is yet to be achieved with machine learning are being pushed to new frontiers as researchers continue to refine and expand the capabilities of deep learning models.
An emerging and notable trend in the field of artificial intelligence is the convergence of machine learning with cutting-edge technologies like edge computing and the Internet of Things (IoT). Such a trend entails the processing and analysis of data at the edge of the network, enabling real-time insights and driving efficiency gains. Organizations reduce latency and enhance responsiveness by deploying machine learning models directly on edge devices or within IoT ecosystems. Such integration paves the way for transformative applications, such as predictive maintenance in industrial settings, anomaly detection in smart infrastructure, and real-time decision-making in critical domains. The potential for immediate, data-driven actions and intelligent automation across diverse industries continues to expand.
Transfer learning is another remarkable trend in machine learning, where pre-trained models are utilized as a starting point for training new models on different tasks or datasets. Transfer learning permits leveraging the knowledge and representations learned from one task to improve performance on another related task with limited training data. The approach accelerates model development and reduces the requirement for extensive labeled datasets.
Additionally, there is a growing emphasis on the ethical considerations and interpretability of machine learning models. There is a pressing need for openness and equity in the use of machine learning algorithms in important decision-making processes. Concerns about bias, explainability, and potential hazards are motivating researchers and practitioners to focus on methods to assure fairness, interpretability, and accountability in machine learning models.
11. Robotic process automation
Robotic Process Automation (RPA) entails the deployment of software robots or bots to streamline and automate repetitive, rule-based tasks within business processes. Such cutting-edge technology empowers organizations to automate labor-intensive and error-prone activities by simulating human interactions with digital systems. Software robots adeptly undertake activities such as data entry, data extraction, form completion, report generation, and other mundane tasks, liberating human workers to dedicate their skills and expertise to more intricate and high-value endeavors through RPA. RPA exemplifies the relentless pursuit of operational excellence by driving efficiency gains and enhancing productivity across a wide range of industries and sectors.
The latest trends in robotic process automation focus on expanding its capabilities and integrating it with other emerging technologies. One significant trend is the integration of RPA with artificial intelligence (AI) and machine learning (ML) algorithms. Organizations enable intelligent automation by combining RPA with AI and ML, where software robots learn from data, make decisions, and adapt to changing scenarios. The integration enhances the capabilities of RPA systems, allowing them to handle more complex tasks that require cognitive abilities, such as natural language processing and image recognition.
Another emerging trend is the adoption of supervised RPA. Attended RPA involves human-robot collaboration, unlike traditional unattended RPA, where software robots work autonomously. Attended RPA allows software robots to assist human workers in real time, providing them with suggestions, automating repetitive steps, and streamlining their workflows. The collaborative approach increases efficiency, reduces errors, and improves the overall productivity of human workers.
There is a growing emphasis on scalability in RPA implementations. Organizations are looking for RPA platforms that scale easily to handle large volumes of transactions and processes across different departments and business units. The latest RPA tools provide centralized control and management capabilities, allowing organizations to scale their RPA initiatives effectively and manage multiple robots on a single platform.
The security and governance aspects of Robotic Process Automation (RPA) have garnered heightened attention as its adoption continues to proliferate. Organizations now place paramount importance on upholding the integrity, confidentiality, and compliance of their automated processes. RPA platforms are increasingly incorporating advanced security features to address these concerns, such as robust encryption mechanisms, granular access controls, and comprehensive audit trails. These measures serve to safeguard sensitive data and mitigate potential risks, ensuring that RPA implementations adhere to stringent security standards and regulations. Organizations confidently leverage the transformative potential of RPA while upholding the trust and privacy of their data assets by prioritizing security and governance.
The latest trends in Robotic Process Automation involve its integration with other technologies such as process mining, intelligent document processing, and workflow management systems. These integrations enable end-to-end automation by combining the capabilities of different technologies and streamlining entire business processes.
12. AI optimized hardware
AI optimized hardware refers to dedicated hardware components or systems that are intricately engineered to optimize the performance and efficiency of artificial intelligence (AI) workloads. These hardware solutions are meticulously crafted to cater to the distinctive computational demands of AI algorithms, enabling accelerated processing capabilities, enhanced precision, and energy-efficient operations. Organizations harness the full potential of AI technologies, achieving faster and more efficient execution of complex AI tasks, and unlocking new possibilities for advanced machine learning models and applications by leveraging purpose-built hardware. The development and integration of AI optimized hardware exemplifies the continuous drive towards refining and fine-tuning the underlying infrastructure supporting AI advancements.
AI optimized hardware often incorporates specialized processors, such as graphics processing units (GPUs), field-programmable gate arrays (FPGAs), tensor processing units (TPUs), or application-specific integrated circuits (ASICs). These processors are designed to handle the parallel processing demands of AI tasks, such as deep learning, by efficiently executing matrix operations and neural network computations.
The most recent reports on AI-optimized hardware highlight the continual breakthroughs and innovations being made in this area of study. The development of artificial intelligence processors that are both more powerful and more efficient is a trend worth noting. Companies are pouring money into research and development in order to produce specialized processors that are tailor-made to handle the workloads associated with AI. These chips make use of cutting-edge technology to achieve considerable performance increases and energy savings. Some examples of these technologies include sophisticated semiconductor processes and unique architectural designs.
There is a focus on specialized hardware solutions for specific AI applications. For example, hardware accelerators optimized for natural language processing (NLP) tasks, computer vision, or recommendation systems are being developed to provide targeted performance boosts for these domains. These specialized hardware solutions enable more efficient and cost-effective AI deployments across a wide range of industries and use cases.
Another notable trend is the integration of AI-optimized hardware into edge devices and Internet of Things (IoT) devices. It enables AI processing to be performed locally on the device itself, reducing reliance on cloud-based infrastructure and enabling real-time AI inference in edge computing scenarios. The integration of AI-optimized hardware at the edge enhances privacy, reduces latency, and enables AI applications in resource-constrained environments.
There is a growing emphasis on energy-efficient AI-optimized hardware. Research and development efforts are focused on creating power-efficient processors and architectures that handle demanding AI workloads while minimizing energy consumption. It is crucial for sustainable AI deployments and enabling AI applications on mobile devices, autonomous vehicles, and other battery-powered devices.
13. Natural language generation
Natural language generation (NLG) is an artificial intelligence subfield that focuses on the synthesis of human-like, coherent, and contextually relevant text from input data. NLG systems examine structured data, such as statistics, facts, or data points, and translate it into natural language text using algorithms and language models. The goal is to create written content that is indistinguishable from human-authored text. NLG is used in a variety of disciplines, including data reporting, content enhancement, virtual assistants, chatbots, and more.
The most recent news about natural language generation highlights advances in the quality and sophistication of generated text. Researchers and developers are improving NLG systems’ fluency, coherence, and inventiveness. It entails training big language models with massive volumes of text data to represent the nuances and complexities of human language. Modern NLG models are capable of producing highly realistic and contextually relevant text, displaying outstanding language knowledge and expressive abilities.
There is a rising emphasis on customized and adaptive natural language generation. Developers are looking into methods for adjusting generated text to particular user preferences, situations, or target audiences. It enables more personalized communication and content creation, thereby increasing user engagement and happiness. Personalized NLG is used in marketing, customer service, and content suggestions.
The incorporation of multimodal capabilities is another key trend in natural language creation. Natural Language Generation systems are being coupled with computer vision and other modalities to generate text that describes visual information or other sensory inputs. Such connections allow for applications like image captioning, video summarization, and interactive storytelling, in which generated text complements and improves multimedia information.
Ethical considerations in natural language production are becoming increasingly essential. Researchers are investigating methods to ensure ethical and fair text generation and avoid concerns such as disinformation, bias amplification, or unsuitable content. The emphasis in the created language is on defining ethical principles, increasing openness, and encouraging justice and diversity.
14. Speech recognition
Automatic speech recognition (ASR), more generally known as speech recognition, is a technology capable of converting spoken language into written text. Speech recognition is a subset of ASR. It is an interdisciplinary field that combines knowledge from areas such as linguistics, signal processing, and machine learning. Speech recognition systems analyze audio recordings or real-time speech input and utilize algorithms to identify and transcribe spoken words into textual form. The technology has applications in various domains, including voice assistants, dictation software, call center automation, transcription services, and more.
The recent news in speech recognition showcases advancements in accuracy, performance, and application diversity. Researchers and developers are continuously striving to improve the accuracy of speech recognition systems, aiming to reduce errors and enhance the overall user experience. It is achieved through advancements in machine learning algorithms, neural network architectures, and large-scale training data. State-of-the-art speech recognition models are achieving impressive levels of accuracy, rivaling human transcription capabilities in certain contexts.
The integration of speech recognition with other technologies is expanding its range of applications. Speech recognition is being combined with natural language processing (NLP) techniques to enable more sophisticated voice assistants capable of understanding and responding to complex queries and commands. Speech recognition is being utilized in multilingual and cross-lingual applications, facilitating communication and information access across different languages and cultures.
There is growing interest in developing speech recognition systems that are robust in challenging acoustic conditions. It entails addressing issues such as background noise, accents, and speech variations, making speech recognition more reliable in real-world environments. Researchers are exploring methods like data augmentation, domain adaptation, and transfer learning to improve system performance in diverse acoustic settings.
Another significant trend in speech recognition is the integration of privacy and security measures. Concerns regarding data privacy and security have emerged with the increasing use of voice-activated devices and voice-controlled applications. Efforts are being made to develop privacy-preserving speech recognition techniques that ensure user data is protected and processed securely.
15. Deep learning platforms
Deep learning platforms refer to software frameworks and tools that are specifically developed to facilitate the process of building, training, and deploying deep learning models. Deep learning is a branch of machine learning in which artificial neural networks are used to learn and extract patterns from massive volumes of data. Deep learning platforms offer a comprehensive collection of capabilities and libraries that allow researchers and data scientists to create and train large neural networks for applications like image identification, natural language processing, and speech recognition.
Recent developments in deep learning platforms have centered around substantial progress in their capabilities, user-friendliness, and seamless integration with complementary technologies. A notable trend is the growing availability of intuitive platforms that facilitate the construction and implementation of deep learning models. These platforms offer user-friendly interfaces, pre-existing models, and streamlined workflows, democratizing the field of deep learning and widening its accessibility to people with varying levels of programming proficiency or machine learning knowledge. Such advancements contribute to accelerating the adoption of deep learning procedures and empowering a broader user base to leverage the transformative potential of this cutting-edge technology.
Another notable trend is the integration of deep learning platforms with cloud computing and edge devices. Cloud-based deep learning platforms allow users to leverage the scalability and computational power of the cloud to train and deploy large-scale deep learning models. On the other hand, edge-based platforms enable running deep learning models directly on devices with limited computing resources, such as smartphones, IoT devices, and autonomous vehicles. Such integration opens up opportunities for real-time inference and decision-making at the edge, enabling applications like object recognition, voice assistants, and autonomous systems.
The latest progress in deep learning platforms encompasses significant advancements in the domains of model interpretability and explainability. There is a rising demand for comprehending the rationale behind their predictions and decisions as deep learning models become increasingly intricate and find applications in critical domains. Researchers and developers, in response, are actively exploring novel techniques and tools to unravel and articulate the inner workings of deep learning models. Such pursuit aims to facilitate a deeper understanding of the models, foster trust and confidence in their outcomes, and instill a sense of accountability in their deployment. The pursuit of interpretability and explainability enhances transparency and empowers users to make informed decisions based on a clearer comprehension of the underlying mechanisms at play by shedding light on the black-box nature of deep learning.
Deep learning platforms are embracing collaboration and knowledge sharing. Open-source platforms and communities foster collaboration among researchers and practitioners, enabling the exchange of ideas, algorithms, and best practices. The collaborative approach accelerates innovation and drives the development of state-of-the-art deep learning platforms and techniques.
16. Decision management
Decision management is a strategic practice that aims to enhance and integrate decision-making activity within organizations through the intelligent utilization of technology, data analysis, and decision modeling techniques. Decision management empowers businesses to come up with more informed, consistent, and efficient decisions across a wide range of areas by leveraging advanced tools and approaches. It involves identifying critical decisions, establishing clear rules and criteria for making those decisions, integrating relevant data and analytics, and implementing automated systems to support the decision-making process. Organizations optimize their decision-making capabilities, drive better outcomes, and gain a competitive edge in today’s fast-paced business landscape through the adoption of modern decision management practices.
Several trends and advancements are shaping the field. One significant development is the increasing integration of artificial intelligence (AI) and machine learning (ML) into decision-management processes, according to the latest news about decision management. Organizations are leveraging AI and ML algorithms to analyze large volumes of data, detect patterns, and generate insights that inform decision-making. It enables organizations to make more data-driven and predictive decisions, leading to improved outcomes and a competitive advantage.
Another emerging trend in decision management is the adoption of real-time decision-making capabilities. Organizations now make decisions in near real-time, leveraging streaming data and sophisticated algorithms with advancements in data processing and analytics technologies. It allows for faster responses to changing conditions, dynamic adjustments to business processes, and improved customer experiences.
Decision management is increasingly focusing on explainability and transparency.
As AI-driven decision-making becomes more prevalent, there is a growing need to understand and interpret the rationale behind automated decisions. Organizations are investing in technologies and approaches that provide insights into decision logic, allowing for better understanding, auditing, and compliance with regulatory requirements.
The application of decision management is expanding across diverse industries and domains. Organizations are recognizing the value of optimized decision-making processes from healthcare to finance, manufacturing to retail. They are implementing decision management systems and platforms that enable them to seize and manage decision logic, align decision-making with business objectives, and continuously improve decision performance.
What is Artificial Intelligence?
The development of computer systems that accomplish tasks that normally require human intelligence is referred to as artificial intelligence (AI). It encompasses a vast array of elaborate techniques and approaches, meticulously crafted to enable machines to exquisitely emulate the cognitive prowess of humanity. These include the art of reasoning, the refinement of learning, the mastery of problem solving, and the discernment of decision making. Artificial intelligence, at its foundation, tries to create algorithms and models that analyze and interpret data, discover patterns, and make intelligent predictions or judgments.
Machine learning, deep learning, natural language processing, and computer vision are just some of the cutting-edge technologies that AI is utilizing to bring about a revolution in a number of different industries. Algorithms in machine learning enable computers to learn from data and gradually improve their performance, all without human intervention. Researchers have turned to deep learning, a subfield of machine learning that makes use of artificial neural networks to extract complex features and hierarchical representations from data to create complex models for applications like picture and speech recognition.
The goal of natural language processing is to give computers the ability to comprehend, interpret, and produce human language. Such interactions are expected to enhance human-machine communication and interaction. Applications like object identification, picture categorization, and facial recognition are all made possible through the use of computer vision, which entails teaching machines to interpret and analyze visual information from photos or videos.
The exponential growth of data, the accessibility of powerful computing resources, and advancements in algorithms and models are what are driving the modern AI landscape. AI applications are prevalent in various domains, including healthcare, finance, manufacturing, transportation, and entertainment. Examples include personalized medicine, autonomous vehicles, smart virtual assistants, recommendation systems, and fraud detection.
AI has enormous promise and potential, but it poses significant ethical and societal concerns. Issues such as algorithmic bias, privacy concerns, job displacement, and the influence of technology on human decision-making are ongoing topics of discussion and investigation. It is essential to develop AI technologies ethically and responsibly, assuring transparency, fairness, and accountability.
The profound influence of AI on society and the economy is going to be nothing short of revolutionary as it relentlessly progresses. The following exquisite technological advances elevate productivity, refine decision-making processes, and propel innovation throughout various industries.
How is AI pushing the boundaries of innovation?
The use of AI is enabling breakthroughs in many different industries that were previously thought impossible.AI has the ability to completely transform industries by allowing machines to accomplish jobs that previously required human intelligence. One key aspect where AI is pushing boundaries is data analysis and decision-making. AI algorithms uncover patterns, insights, and correlations that humans miss with their ability to process vast amounts of data quickly and efficiently. It opens up new avenues for predictive analytics, optimization, and personalized recommendations, enhancing the efficiency and effectiveness of businesses and organizations.
Artificial intelligence is driving innovation in a variety of industries, including healthcare and medicine. Medical professionals get to evaluate medical images, spot trends, and aid in the diagnosis of diseases with greater precision thanks to the use of artificial intelligence (AI)-driven tools. The processing of patient data by AI algorithms, the identification of abnormalities, and the prediction of disease consequences all lead to more individualized and accurate therapies.
AI is causing a revolution in the transportation, robotics, and manufacturing industries within the field of autonomous systems. The use of artificial intelligence algorithms to guide vehicles and enable them to make judgments in real time while they are out on the road is quickly becoming a reality. Robots armed with artificial intelligence are capable of completing difficult jobs, working in tandem with humans, and adjusting to ever-evolving situations. Systems in manufacturing that are AI-powered streamline production processes, enhance quality control, and enable predictive maintenance, all of which help to lower costs and boost productivity.
The use of AI is changing how businesses interact with their customers. Natural language processing and machine learning algorithms enable virtual assistants, chatbots, and recommendation systems, allowing them to offer distinctive and enjoyable experiences for each user. Companies are able to learn their customers’ preferences, foresee their requirements, and provide them with more personalized products and services with the help of AI.
AI’s ability to learn and improve over time is expanding the bounds of what’s possible in terms of technological progress. Reinforcement learning techniques allow AI systems to learn through trial and error, enabling them to adapt and optimize their performance in dynamic environments. The adaptive capability opens up possibilities for autonomous agents, intelligent systems, and adaptive algorithms that continuously evolve and enhance their capabilities.
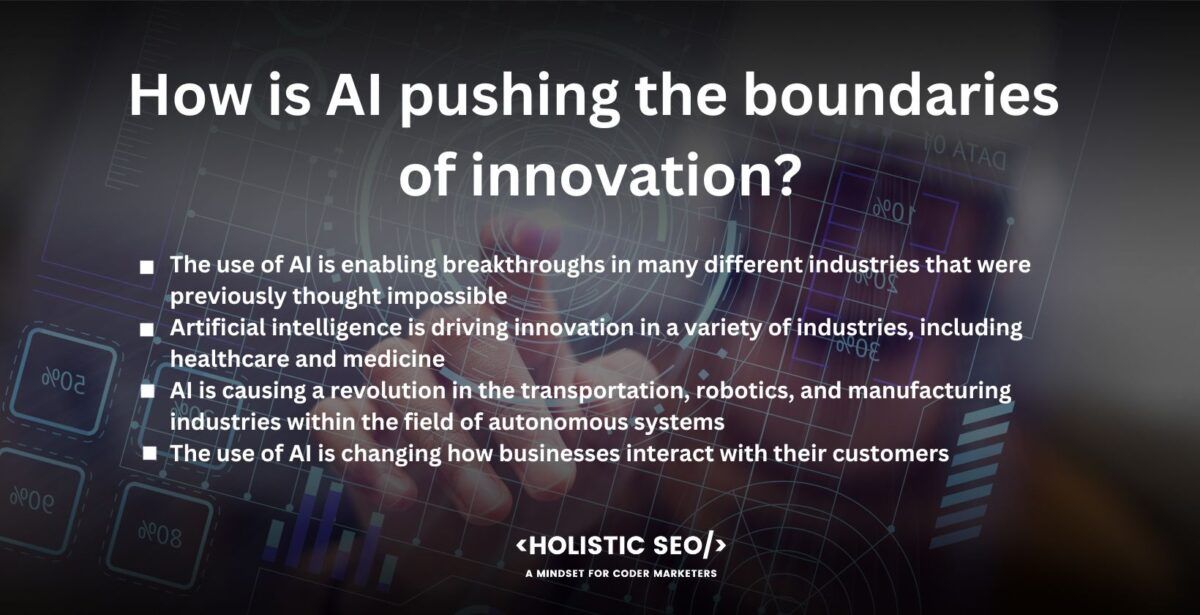
What are the breakthroughs in machine learning algorithm?
Advancements in AI have paved the way for significant breakthroughs in machine learning algorithms, revolutionizing the capabilities and applications of such a field. One major breakthrough is the development of deep learning algorithms, which are neural networks with multiple layers that learn hierarchical representations of data. Deep learning has demonstrated remarkable success in various domains, such as computer vision, natural language processing, and speech recognition.
Another breakthrough is the emergence of reinforcement learning, where an agent learns to make decisions based on feedback from its environment. It has enabled AI systems to achieve impressive feats, such as defeating world champions in complex games like Go and achieving superhuman performance in video games. There have been advancements in transfer learning, which allows models to leverage knowledge from one domain to excel in another, even with limited data. It has accelerated progress in areas where labeled datasets are scarce.
There have been breakthroughs in generative models, such as generative adversarial networks (GANs) and variational autoencoders (VAEs), enabling the generation of realistic images, videos, and other types of synthetic data. These breakthroughs in machine learning algorithms have opened up new possibilities in areas like healthcare, autonomous systems, robotics, and personalized recommendations, among others. They have propelled the field of AI forward, fostering innovation and pushing the boundaries of what is possible in artificial intelligence.
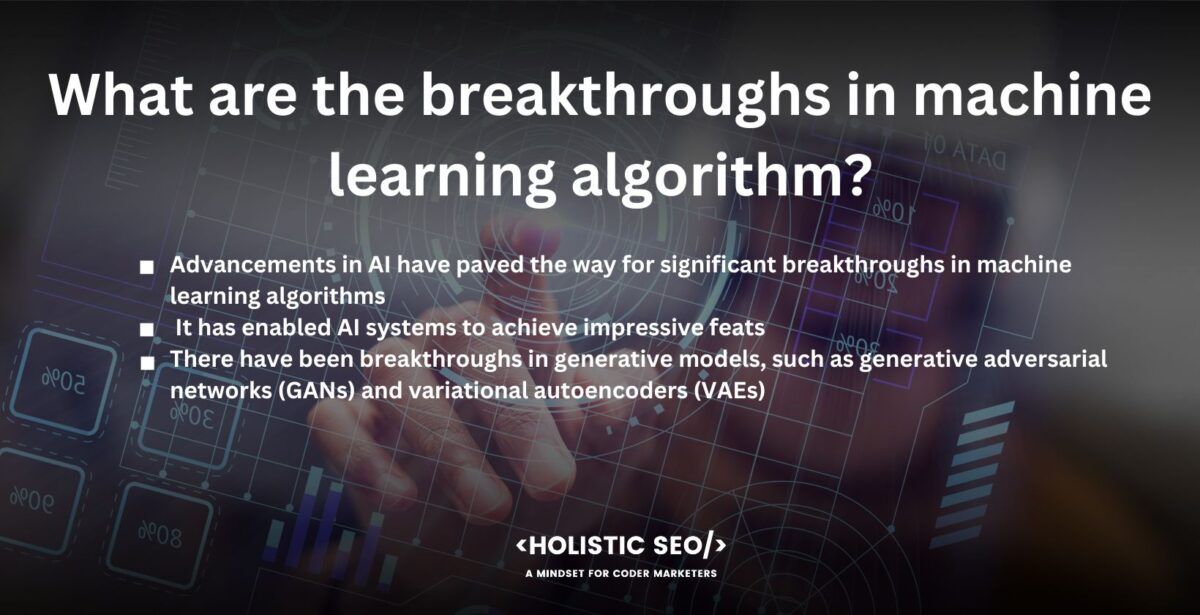
How Did AI Transform Industries with Its Latest Breakthrough?
The latest advancements in AI have brought about transformative changes across industries, reshaping business models, and driving innovation. One of the key ways AI has transformed industries is through its ability to process and analyze vast amounts of data. It has led to more accurate predictions, improved decision-making, and enhanced operational efficiency. For example, AI algorithms in healthcare analyze medical records, genetic data, and clinical images to assist in diagnosis, treatment planning, and drug discovery. AI-powered systems in finance analyze complex financial data, detect patterns, and make real-time trading decisions.
AI has revolutionized manufacturing by enabling the automation of repetitive tasks, enhancing precision and productivity. The transportation industry has witnessed the emergence of self-driving vehicles, empowered by AI technologies such as computer vision and machine learning, which promise safer and more efficient transportation systems. AI has transformed customer service through chatbots and virtual assistants, providing personalized and responsive interactions.
AI-driven recommendation systems in the retail sector enhance customer experiences by suggesting relevant products based on individual preferences and browsing history. The energy and utilities sectors benefit from AI in optimizing energy consumption, managing grids, and predicting maintenance needs. Overall, the latest breakthroughs in AI have unleashed unprecedented possibilities, empowering industries to streamline operations, improve customer experiences, and unlock new opportunities for growth and innovation.
What Are the Ethical Implications of Ai?
Listed below are the Ethical Implications of AI.
- Bias and Discrimination: AI is going to reinforce existing biases and discrimination if its systems are taught on biased data. Such a process leads to unfair results in areas like hiring, lending, and criminal justice.
- Privacy and Data Security: AI often relies on collecting and analyzing large amounts of personal data, raising concerns about privacy breaches and unauthorized access to sensitive information.
- Job Displacement: The automation potential of AI raises concerns about job displacement and the need for re-skilling or re-training of the workforce, leading to economic and societal challenges.
- Accountability and Transparency: AI systems, particularly complex deep learning models, are challenging to interpret and understand. Ensuring transparency and accountability for AI decisions is crucial, especially in areas with significant impacts on human lives, such as healthcare and autonomous vehicles.
- Autonomous Weapons: The development of AI-powered autonomous weapons raises ethical dilemmas regarding the delegation of lethal decision-making to machines and the potential for unintended consequences or misuse.
- Informed Consent: AI systems that process personal data must ensure informed consent and provide individuals with a clear understanding and control over how their data is used.
- Fairness and Equity: AI algorithms must be designed to mitigate biases and ensure fair treatment across different demographic groups, avoiding discrimination and unequal outcomes.
- Human-Centered Design: Prioritizing human well-being, safety, and dignity must be central to the development and deployment of AI systems, avoiding harm and preserving human autonomy.
- Social and Economic Impact: AI technology has the potential to exacerbate social inequalities and concentrate power in the hands of a few, necessitating proactive measures to ensure equitable access and benefits for all.
- Long-Term Implications: Exploring Ethical considerations in AI must extend to the long-term impact of AI on society, including its effects on democratic processes, human relationships, and the overall quality of life.
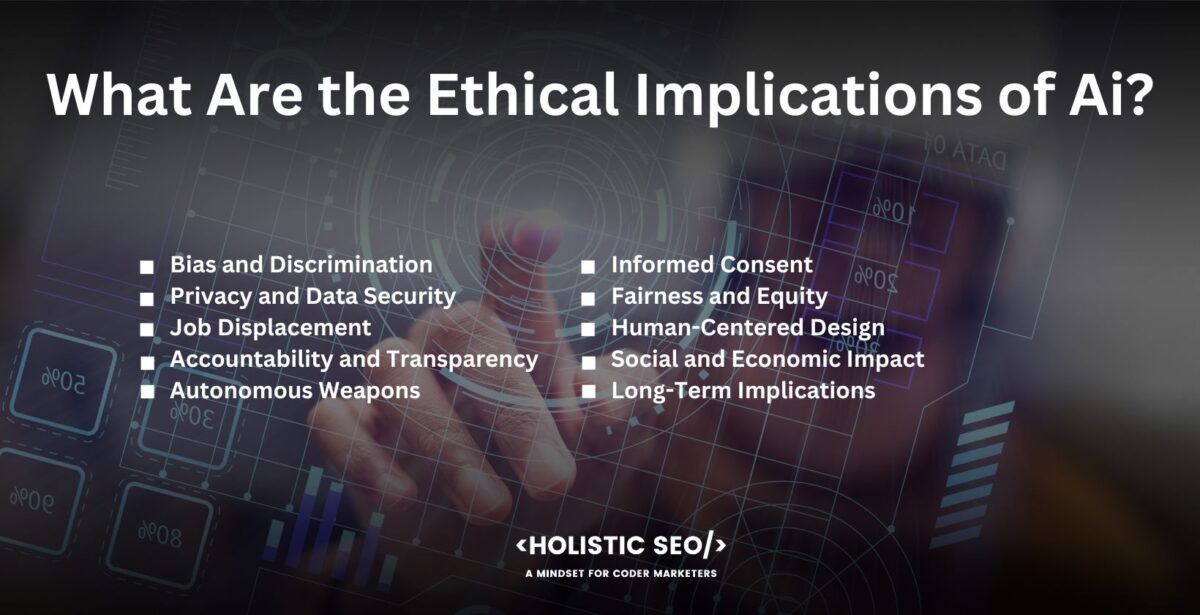
How are deep learning models evolving AI?
Deep learning models are playing a pivotal role in advancing AI technology. These complex neural networks, inspired by the structure and function of the human brain, have the capability to learn and extract intricate patterns from vast amounts of data. AI systems autonomously recognize, analyze, and understand complex patterns in images, text, speech, and other forms of unstructured data by leveraging deep learning algorithms.
Such new AI technology has revolutionized various domains, such as computer vision, natural language processing, and speech recognition. Deep learning models have significantly improved the accuracy and performance of AI systems, enabling them to achieve human-level or even superhuman-level performance in specific tasks.
The continuous evolution of deep learning models, along with advancements in computational power and data availability, is driving the development of increasingly sophisticated AI applications, ranging from autonomous vehicles and healthcare diagnostics to virtual assistants and recommendation systems. These deep learning models are at the forefront of pushing the boundaries of AI capabilities and unlocking new possibilities for solving complex problems and enhancing human experiences.
What are the Benefits of AI Technology?
Listed below are the Benefits of AI Technology.
- Automation and Efficiency: Artificial intelligence allows for the automation of routine tasks, resulting in greater efficiency and output. Such a process allows people to devote their time and energy to higher-level, more strategic endeavors.
- Improved Accuracy and Precision: AI algorithms analyze large amounts of data quickly and accurately, reducing errors and providing precise insights. It is particularly valuable in areas such as data analysis, pattern recognition, and predictive modeling.
- Enhanced Decision-Making: AI systems process vast amounts of information and provide valuable insights to support decision-making. They identify patterns, trends, and correlations that humans overlook, leading to more informed and data-driven decisions.
- Personalization and Customer Experience: AI enables personalized experiences by analyzing customer data and preferences. Such a process allows businesses to tailor products, services, and recommendations to individual customers, enhancing customer satisfaction and loyalty.
- Advanced Healthcare: AI technology has the potential to revolutionize healthcare by enabling early disease detection, precise diagnostics, and personalized treatment plans. It analyzes medical records, images, and genetic data to assist doctors in making accurate diagnoses and optimizing patient care.
- Smart Assistants and Chatbots: AI-powered virtual assistants and chatbots provide 24/7 customer support, answering queries, and guiding users through various processes. They enhance customer service, improve response times, and streamline interactions.
- Increased Safety and Security: AI systems are utilized for security purposes, such as facial recognition, behavior analysis, and anomaly detection. They enhance surveillance, detect potential threats, and help prevent criminal activities.
- Efficient Resource Management: AI optimizes resource allocation and energy consumption in various sectors, such as transportation, manufacturing, and agriculture. It leads to cost savings, reduced waste, and improved sustainability.
- Enhanced Creativity: AI technology assists in creative endeavors, such as generating music, art, or content. It provides inspiration, suggests ideas, and augments human creativity, leading to new and innovative outputs.
- Continuous Learning and Improvement: AI systems have the ability to learn from data and experiences, allowing them to continuously improve their performance over time. Adaptability and self-learning capability contribute to ongoing advancements in AI technology.
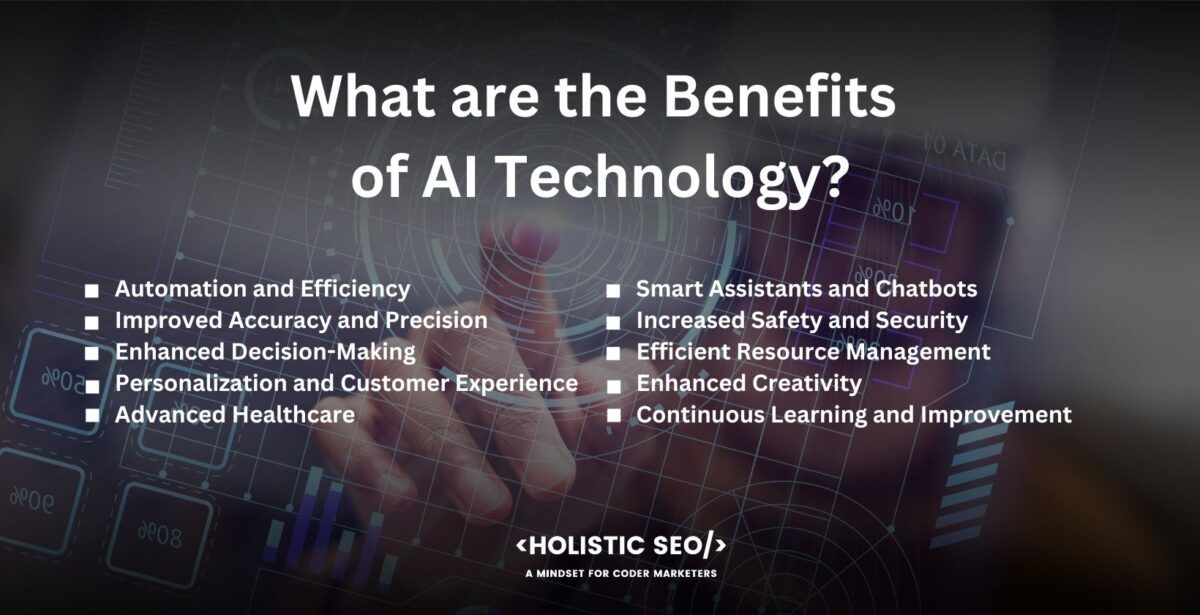
What are the Challenges with AI Technology?
Listed below are the Challenges with AI Technology.
- Data Quality and Bias: AI algorithms heavily rely on data for training and decision-making. If the data used is of poor quality or biased, it leads to inaccurate or unfair outcomes. Ensuring data quality and mitigating biases in AI systems is a critical challenge.
- Ethical and Legal Considerations: AI raises ethical dilemmas, such as privacy concerns, algorithmic transparency, and accountability. Determining who is responsible for AI decisions and addressing potential ethical issues requires careful consideration and regulatory frameworks.
- Lack of Explainability: AI algorithms often operate as black boxes, making it difficult to understand how they arrive at specific conclusions or decisions. The lack of interpretability and explainability hinders trust and limits the adoption of AI in critical applications.
- Limited Generalization: AI models trained on specific datasets struggle to generalize their knowledge to new or different scenarios. Such a limitation hinders the scalability and applicability of AI solutions across various domains.
- Security Risks: They are vulnerable to cyberattacks as AI systems become more pervasive. Adversarial attacks, data poisoning, and unauthorized access to AI models pose security risks that need to be addressed to maintain the integrity and reliability of AI systems.
- Workforce Displacement: The automation capabilities of AI raise concerns about job displacement. Certain roles and tasks become obsolete as AI technology advances, requiring a reevaluation of workforce skills and the creation of new job opportunities.
- Human-AI Collaboration: Effective integration of AI technology with human decision-making processes is a challenge. Ensuring that AI systems augment human intelligence, rather than replace it, requires careful consideration of human-AI collaboration models and interfaces.
- Continuous Learning and Adaptation: AI models struggle to adapt to evolving environments or handle unexpected scenarios. Developing AI systems that continuously learn, adapt, and handle novel situations is an ongoing challenge.
- Regulation and Governance: The rapid advancement of AI technology calls for appropriate regulations and governance frameworks. Balancing innovation and accountability, addressing potential biases and risks, and ensuring responsible AI development and deployment are essential challenges.
- Lack of AI Expertise: There is a shortage of skilled professionals with expertise in AI development, deployment, and management. Bridging the skills gap and promoting AI literacy across various disciplines is crucial for leveraging the full potential of AI technology.
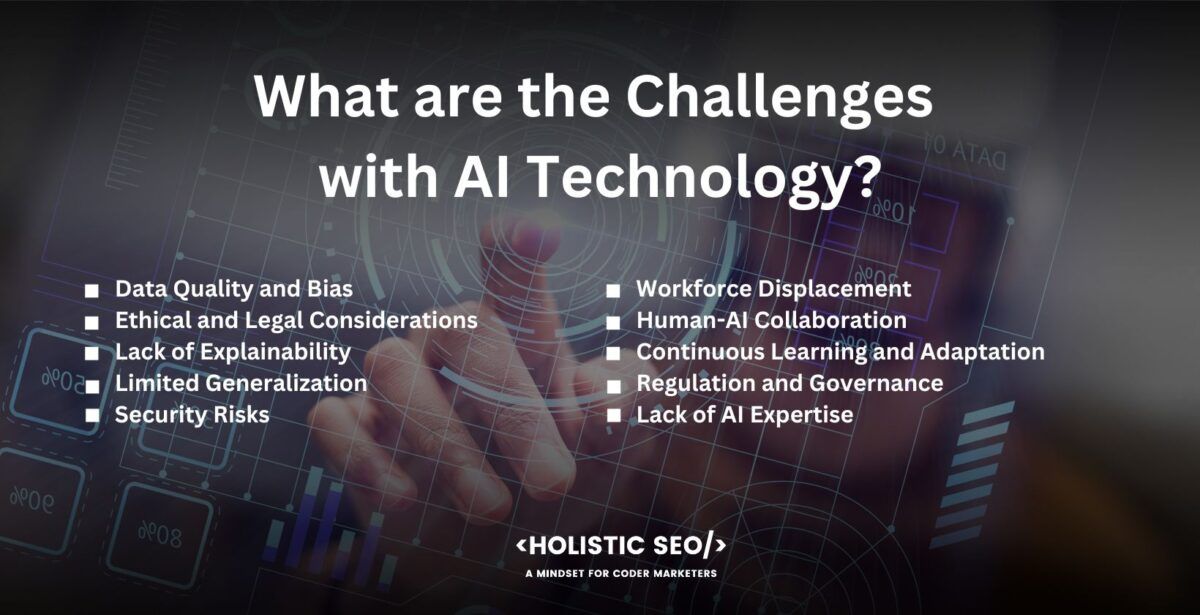
What are the Implications of Self Driving AI Vehicles?
Self-driving AI vehicles have profound implications that revolutionize transportation systems and reshape multiple industries. The newest AI technology fueling autonomous vehicles holds immense potential. Self-driving cars significantly enhance road safety, addressing a primary cause of accidents by minimizing human error.
Optimized route planning and coordinated vehicle movements lead to improved efficiency and reduced traffic congestion. Self-driving AI vehicles offer enhanced mobility solutions for individuals with limited driving capabilities, such as the elderly or those with disabilities, promoting independence and enhancing their overall quality of life. However, the widespread adoption of autonomous vehicles necessitates careful consideration of ethical and legal concerns, particularly those pertaining to liability and decision-making in critical situations.
The impact on employment within the transportation and logistics sectors must be thoughtfully managed. Ensuring the security and privacy of AI systems controlling these vehicles is crucial to mitigating cybersecurity risks. The integration of self-driving AI vehicles requires a comprehensive evaluation of technical, ethical, societal, and regulatory factors to ensure a safe and successful transformation of our transportation systems.
What Type of AI Technology are AI Newsletters?
AI newsletters harness a diverse array of AI technologies to enhance the process of content delivery and curation. These newsletters capitalize on cutting-edge advancements in AI, such as natural language processing (NLP) and machine learning algorithms, to effectively gather, analyze, and personalize content for their subscribers. NLP empowers newsletters to sift through vast volumes of data encompassing news articles, research papers, and industry reports, extracting pertinent information and insights.
Newsletters assimilate user preferences and behavior, thereby delivering customized content recommendations and elevating the overall user experience through the utilization of machine learning algorithms. These newsletters streamline tasks like content categorization, summarization, and sentiment analysis, automating processes and ensuring efficient and precise content selection by leveraging AI technology.
These AI newsletters provide subscribers with timely, curated, and personalized updates on the latest news, trends, and insights in the field of AI by leveraging AI to keep them informed and engaged in the rapidly evolving landscape of artificial intelligence.
Will the AI Technology be Able to Replace Most of the Work Force?
No, AI technology is not going to replace most of the workforce. It is quite improbable that AI technology is going to completely eradicate the need for human labor. It is more plausible to imagine a future in which AI augments and transforms employment rather than entirely replacing it. However, artificial intelligence has the potential to automate certain tasks and have an impact on specific industries. The reason is that AI has the potential to automate specific tasks and impact certain businesses.
Artificial intelligence is particularly good at automating routine and repetitive work, which frees up humans to concentrate on higher-level talents such as creative thinking, critical thinking, problem-solving, and emotional intelligence. AI systems have limits in domains that demand complicated human interaction, the ability to adapt to novel circumstances, and the ability to make ethical decisions. Careful consideration needs to be given to the social and economic repercussions that result from a widespread loss of jobs.
AI technology is more likely to create new job roles, change job functions, and necessitate the upskilling and reskilling of workers in order for them to effectively collaborate with AI systems. Such a trend is more likely to occur than it is for AI to replace the workforce. It is anticipated that the job of the future is going to involve a combination of human and artificial intelligence (AI) collaboration, in which humans contribute their one-of-a-kind qualities alongside AI technology to promote creativity and productivity.
Do AI Technology have a Human Like Decision Making or Free Will?
No, Artificial intelligence (AI) software does not have the ability to make decisions or exercise free will in the same way that humans do. AI systems are intended to examine data, identify patterns, and arrive at conclusions or recommendations based on a set of predetermined rules and algorithms. These algorithms are the product of human programmers, and they are built with the express purpose of resolving particular challenges or issues.
It lacks the subjective experience, consciousness, and complex cognitive capacities that are linked with human decision-making and free will, despite the fact that AI is capable of displaying outstanding capabilities in terms of data processing, pattern recognition, and decision-making within predetermined parameters. AI functions according to the pre-programmed algorithms and data it has been taught. It does not have subjective consciousness, feelings, intuition, or personal desires of its own.
AI systems utilize methods such as statistical analysis, mathematical modeling, and pattern recognition in order to arrive at conclusions or generate forecasts. They do not have any innate wants or intentions, nor do they have the capacity to exert personal action or volition on their own. Their behavior is dictated by the algorithms and data with which they have been programmed.
It is essential to keep in mind that while artificial intelligence (AI) technology replicates certain parts of human-like decision-making, it fundamentally operates within the confines of its programming and the data it has been trained on. Such a fact makes it necessary to keep in mind that AI technology simulates certain aspects of human-like decision-making. Research into the creation of AI systems is an ongoing subject of study; nonetheless, decision-making and free will that are truly analogous to those of humans are not now within the purview of AI technology.
Can Supervised Learning be Used for Image Recognition System?
Yes, supervised learning can be utilized for image recognition systems. The algorithm must first be trained on a huge collection of photographs, with each image associated with a certain label or category, to develop an image recognition system using supervised learning. Usually, training data consists of input images and their associated output labels.
The algorithm learns to acknowledge patterns and characteristics in images that are indicative of the various classifications or categories during the training process. It maps these patterns to the relevant labels, resulting in a model that generalizes and predicts new, previously unseen images.
It is used to classify fresh photos by studying their attributes and suggesting the most likely label or category once trained. The caliber and variety of the training data, as well as the complexity and potency of the machine learning algorithm used, all affect how accurate and effective the image recognition system is.
Supervised learning techniques such as convolutional neural networks (CNNs) are extensively utilized in image recognition systems because of their capability to learn and extract characteristics from images. These models work well in tasks including object recognition, facial recognition, and image categorization.
Overall, supervised learning is a powerful framework for constructing image recognition systems, allowing for automated picture analysis and classification based on patterns and features acquired from labeled training data.
- 48 Online Shopping and Consumer Behavior Statistics, Facts and Trends - August 22, 2023
- B2B Marketing Statistics - August 22, 2023
- 38 Podcast Statistics, Facts, and Trends - August 22, 2023