Artificial intelligence has emerged as a breakthrough technology with the ability to address time’s most critical issues, such as climate change. The convergence of AI and climate change gives a very rare opportunity to use advanced algorithms, machine learning, and big data analytics to better understand, mitigate, and adapt to the effects of climate change. AI helps to define and understand climate change by processing massive volumes of data and delivering previously unattainable insights. The article investigates the advantages and disadvantages of using AI in the context of climate change and provides an outline of how AI works in addressing global concerns.
AI for climate change is very advantageous in the fight against climate change from a benefits perspective. AI technology aid in the optimization of energy systems by assessing energy usage patterns, finding inefficiencies, and suggesting improvements to reduce greenhouse gas emissions. Artificial intelligence climate change algorithms improve renewable energy generation and distribution by streamlining operations, enhancing forecasting accuracy, and integrating many sources into the power grid. AI models help with climate prediction and modeling, allowing for a better knowledge of climate patterns, extreme weather occurrences, and their consequences. The information is essential for developing successful adaptation and mitigation strategies.
The application of AI in climate change mitigation includes some hazards. One of the key issues is the large amount of energy required to train and run AI models, which unintentionally contributes to carbon emissions. Relying on AI systems reduces human agency and accountability in climate change decision-making processes. There are ethical problems, including data privacy, transparency, and algorithmic biases, that must be properly addressed to produce equitable and just outcomes. Striking a balance between technological advancements and cautious application is essential to maximizing the advantages and minimizing potential risks.
AI systems rely on massive volumes of data to train models, spot trends, and make predictions. Examples of such data include climate records, satellite imaging, sensor data, and socioeconomic indicators. The data are processed by machine learning algorithms to discover correlations, produce insights, and develop prediction models. The discovery of complicated patterns and the extraction of knowledge that is put to use are made by AI techniques such as deep learning, reinforcement learning, and natural language processing. AI enables climate change researchers, governments, and organizations to make educated judgments, devise successful plans, and take preventive measures.
What is AI and Climate Change?
Artificial intelligence is the development of computer systems and algorithms that accomplish tasks that normally require human intelligence. AI includes techniques such as machine learning, natural language processing, and computer vision. AI systems are intended to analyze massive volumes of data, discover patterns, forecast future events, and automate decision-making processes.
Climate change refers to long-term changes in Earth’s climate patterns caused by factors such as rising greenhouse gas emissions, deforestation, and human activity. Change in the climate causes a variety of environmental effects, such as increased temperatures, sea-level rise, extreme weather events, and ecosystem changes.
The application of AI technology and approaches to addressing the difficulties posed by climate change is referred to as the intersection of AI and climate change. The intersection involves employing artificial intelligence to improve climate change understanding, mitigation, and adaptation activities. AI helps define and comprehend climate change by processing massive volumes of data, providing insights, and constructing predictive models. AI aids with energy system optimization, renewable energy generation and distribution, and climate prediction and modeling.
How can artificial intelligence help in mitigating climate change?
Listed below are the ways in which artificial intelligence help in mitigating climate change.
- Energy optimization: Artificial intelligence optimizes energy systems by studying energy usage trends, finding inefficiencies, and recommending modifications to reduce greenhouse gas emissions. Energy optimization allows for smart grid management, demand response, and load forecasting, resulting in more efficient energy consumption and a lower carbon footprint.
- Renewable energy integration: AI algorithms help in the integration of renewable energy sources into the power system. Optimizing renewable energy system management and maintenance, enhancing solar and wind energy forecasting accuracy, and providing effective energy storage choices improves reliability and economic feasibility.
- Climate prediction and modeling: AI-powered models help with climate prediction and modeling by giving useful insights into weather patterns, extreme weather occurrences, and their consequences. The knowledge allows greater preparedness, early warning systems, and the creation of adaptive solutions to offset the effects of climate change.
- Sustainable agriculture and forestry: AI optimizes agricultural practices by assessing soil conditions, meteorological data, and crop characteristics to improve resource management, reduce water usage, optimize fertilizer application, and raise crop yields. AI helps with deforestation monitoring, tree planting campaigns, and forest fire warning, assisting in forest conservation and sustainable management.
- Environmental monitoring and conservation: Remote sensing and satellite imaging analysis, for example, monitor and analyze environmental data on a massive scale using AI-based technologies. The result involves monitoring deforestation, air and water quality, biodiversity hotspots, and detecting illicit activity. The data aids in the design of focused conservation activities and the enforcement of environmental rules.
- Smart transportation and urban planning: AI improves transportation systems by studying traffic patterns, decreasing congestion, and optimizing routing to reduce fuel usage. AI offers assistance with urban planning by optimizing building energy usage, enhancing waste management systems, and supporting sustainable urban development.
- Climate change communication and education: AI-powered chatbots and virtual assistants offer engaging and easily accessible platforms for teaching about climate change, answering queries, spreading knowledge, and promoting sustainable practices.
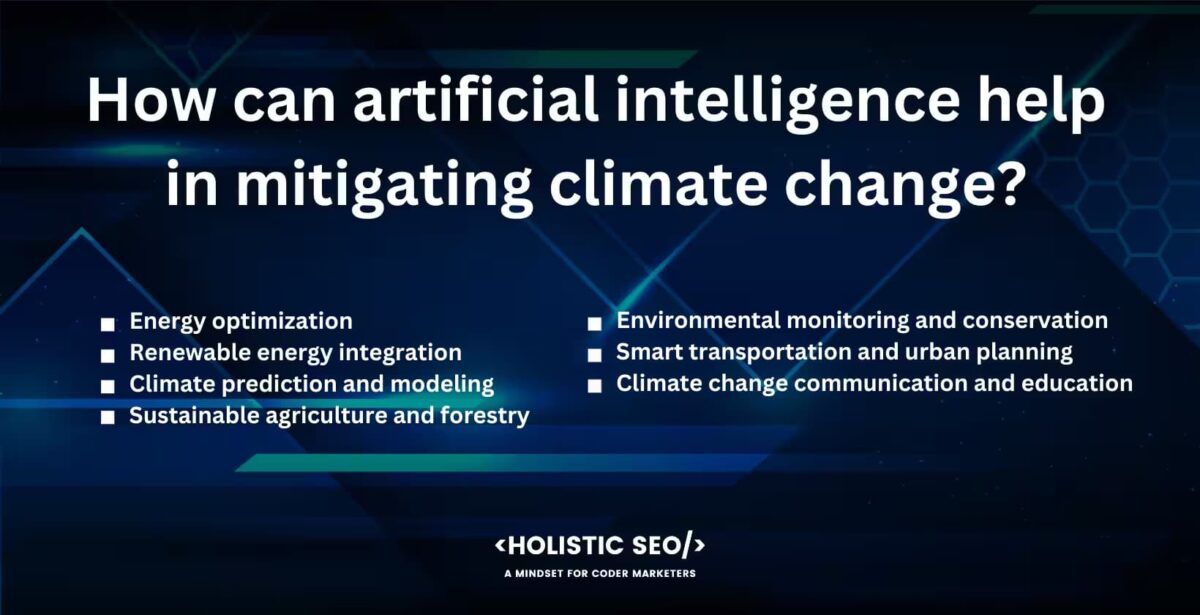
What are the examples of AI and Climate Change?
AI and Climate Change are related fields in which artificial intelligence technology addresses climate change concerns. One example of AI’s application in the current setting is climate modeling and prediction. Researchers use AI algorithms to evaluate large amounts of climate data, uncover patterns, and construct more accurate climate models. The models explain the Earth’s complex climate system and predict future climate scenarios, enabling policymakers to make informed mitigation and adaptation decisions.
Optimization of renewable energy systems is another example. AI algorithms assess real-time data on weather conditions, energy demand, and grid capacity to maximize renewable energy output, storage, and distribution. The assessment of data allows for improved integration of renewable energy sources into the power system, reducing dependency on fossil fuels and minimizing greenhouse gas emissions. The ability of artificial intelligence to optimize energy systems and forecast renewable energy generation helps to create a more sustainable and efficient energy infrastructure.
AI allows precision agriculture practices, which help offset the effects of climate change on food production. Farmers optimize irrigation, fertilization, and pest management by merging AI technologies with sensors, satellite imaging, and data analytics. AI systems assess variables such as soil moisture, crop health, and weather patterns to deliver real-time recommendations, resulting in more resource-efficient farming techniques and a lower environmental effect.
What are the latest breakthrough in using AI and Climate Change?
Numerous businesses and startups are stepping up with creative solutions that leverage the potential of AI and green technology in response to the pressing need to combat climate change. Sipremo is one example of a startup that is on a mission to make cities safe, clean, and sustainable. They created an AI system that predicts future disaster and climate change events, going beyond standard reactive tactics. The foresight gives a tremendous opportunity window for communities to take proactive action before the disasters occur, allowing communities to lessen or even avert the harmful environmental impact that befalls the impacted areas.
The Ocean Cleanup is a non-profit group that has identified the devastation caused by plastic trash in oceans. They are actively deploying AI object detection algorithms to generate scientific observations of floating macroplastic litter as part of their activities. The approach allows the identification and classification of plastic waste in the water, hence improving understanding of the distribution and abundance of the ecological concern. The Ocean Cleanup is going to be successful in strategizing and performing targeted cleanup operations to remove plastic from the ocean using AI. They are ultimately working towards their ambitious goal of eradicating 90% of floating ocean plastic by 2040.
AI is used by Bangalore-based firm Brahm Works to decarbonize India’s agricultural industry. Their AI-powered technology optimizes agricultural inputs and outputs, with a particular emphasis on fertilizer nutrition control. Brahm Works helps farmers to generate higher yields while lowering greenhouse gas emissions by delivering real-time analytics and individualized assistance. The innovative idea illustrates the potential of artificial intelligence in transforming agricultural practices and increasing sustainability in a vital sector of food production. The latest breakthroughs in AI demonstrate how technology promotes good change in the fight against climate change.
How can AI be used to improve renewable energy systems?
AI is utilized in a variety of ways to improve renewable energy systems. Significant use is optimizing the operation and management of renewable energy generation. AI systems examine huge amounts of data from weather conditions, electricity consumption, and grid performance to create accurate predictions and uncover the most efficient ways to generate and distribute renewable energy. AI assists renewable energy systems in better integrating with the grid, improving energy projections, and maximizing the utilization of existing resources.
A key use of AI is the monitoring and maintenance of the infrastructure for renewable energy sources. Sensors and monitoring systems powered by artificial intelligence collect data on the operation of solar panels, wind turbines, and other renewable energy assets in real-time. The data is then analyzed by AI algorithms to find patterns, detect anomalies, and predict equipment breakdowns. Artificial intelligence-based monitoring systems improve the reliability and efficiency of renewable energy systems by proactively detecting and repairing errors, along with decreasing downtime and maintenance costs.
AI help with energy storage optimization. Efficient energy storage is essential for balancing supply and demand because renewable energy is inherently intermittent. Artificial intelligence algorithms assess prior energy use patterns, weather forecasts, and grid conditions to optimize energy storage and establish the most efficient charging and discharging periods for storage systems. AI contributes to increasing the usage of renewable energy while minimizing reliance on fossil-fuel-based backup systems.
AI is hastening the development of smart grid and demand response systems. The use of AI algorithms in grid management allows for real-time synchronization of renewable energy generation with power demand. AI monitors consumption trends, forecasts peak demand periods, and optimizes energy distribution to provide a consistent and stable power supply. AI provides demand response algorithms, which enable consumers to adjust their energy consumption based on price signals or grid conditions, resulting in more efficient energy consumption and reduced grid pressure.
What are the benefits of using AI and Climate Change?
Listed below are the benefits of using AI and Climate Change.
- Enhanced data analysis: AI is capable of processing massive volumes of data from a variety of sources, including satellite photography, weather stations, and climate models. AI algorithms extract useful insights, find patterns, and generate predictions from the data, allowing for a better knowledge of climate dynamics.
- Efficient renewable energy management: AI helps renewable energy systems run more efficiently by forecasting energy demand, optimizing energy generation, and controlling energy storage. Artificial intelligence helps to maximize the use of renewable energy sources while ensuring a dependable and efficient energy supply.
- Early warning systems: Artificial intelligence is utilized to create early warning systems for extreme weather occurrences and natural disasters. AI systems recognize patterns and deliver timely alarms by evaluating historical data and real-time information, enabling preventive efforts to reduce the impact of such incidents.
- Conservation and biodiversity protection: AI uses satellite imagery and other data sources to monitor and safeguard natural ecosystems. AI identifies endangered species, tracks deforestation, and aids in the development of conservation measures, all of which contribute to the preservation of biodiversity.
- Data-driven policy making: Analytics enabled by AI assist policymakers in making evidence-based decisions. AI provides insights into successful policies for reducing climate change and supporting sustainable development by evaluating data on emissions, climate patterns, and socioeconomic aspects.
- Sustainable urban planning: AI aids in creating sustainable cities by evaluating data on energy usage, traffic trends, and infrastructure. Evaluating such data leads to better urban design, more energy-efficient buildings, and better transit networks.
- Effective resource allocation: AI helps in resource allocation by assessing data on water usage, agricultural methods, and natural resource management. AI allows for more efficient resource usage, waste reduction, and the promotion of sustainable behaviors.
- Improved climate modeling and prediction: AI approaches such as machine learning improve climate models by examining past climate data and adding complicated connections. AI increases the precision of climate predictions, which aids in long-term planning and adaptation efforts.

1. Enhanced data analysis
The application of AI approaches to extract significant insights, patterns, and correlations from massive and complex climate-related information is referred to as enhanced data analysis. The analysis involves employing machine learning algorithms, data mining techniques, and predictive analytics to evaluate massive volumes of climate data and extract relevant information that is used to inform climate change research, modeling, and decision-making processes.
The significance of enhanced data analysis in the context of climate change stems from its ability to unleash the potential of large data and provide a greater understanding of climate patterns, trends, and impacts. Researchers and scientists acquire significant insights into climate dynamics, identify dangers, and design effective mitigation and adaptation measures by leveraging AI capabilities.
Climate modeling and prediction are two real-life uses of better data analysis employing AI. AI algorithms examine historical climate data, satellite imagery, and other important environmental parameters to build more accurate and detailed climate models. The models aid in the simulation of future climate scenarios, the prediction of extreme weather occurrences, and the assessment of the impact of climate change on particular ecosystems and areas.
Climate modeling and forecast rely significantly on improved data analysis enabled by AI. The DeepMind team created the AlphaFold is a real-life example of an AI-based method to forecast protein structures. The groundbreaking technology improves climate models and helps predict climate change impacts.
2. Efficient renewable energy management
Using AI to maximize the effectiveness and sustainability of renewable energy generation, distribution, and consumption is efficient renewable energy management. The procedure aims to enhance renewable energy systems’ operational operations and decision-making using AI algorithms and data analytics approaches.
The capacity of AI-based renewable energy management to overcome issues such as unpredictability, intermittency, and poor predictability is a major reason for its prominence. AI technology enables the dynamic tuning of renewable energy systems to achieve a balance between supply and demand, enhance grid stability, and optimize system performance.
Smart grid systems are real-life examples of efficient renewable energy management employing AI. The systems analyze real-time data from numerous sources, such as weather conditions, energy consumption trends, and renewable energy generation, using AI algorithms. AI alters energy production, storage, and distribution based on the insights to ensure optimal exploitation of renewable resources and efficient delivery of energy to end users.
Google’s data centers have been optimized for energy efficiency with the help of AI-powered algorithms. AI algorithms constantly adjust cooling settings and energy usage based on real-time data on energy demand, temperature, and cooling systems, resulting in significant reductions in energy costs. Efficient data center energy management achieved through AI helps mitigate the negative effects of global warming on the environment and enhances overall energy productivity.
3. Early warning systems
Early warning systems use AI technology to detect and predict climate-related hazards and provide timely alerts and notifications to individuals, communities, and organizations. The systems leverage advanced data analysis, machine learning algorithms, and real-time monitoring to identify threats such as extreme weather events, natural disasters, and other climate-related risks.
Early warning systems are essential in the face of climate change because they help communities and governments respond to the increasing frequency and severity of extreme weather events. AI provides preventative actions such as evacuation planning, infrastructure reinforcement, and disaster response coordination by delivering early signals, resulting in less loss of life, property, and economic disruption. The systems help to increase resilience and adaptive ability in the face of climate-related dangers.
The use of AI-based early warning systems for flood detection and management is an example of real-life early warning applications. AI algorithms identify regions at risk of flooding and deliver early alerts to people and authorities by monitoring elements such as rainfall patterns, river levels, and soil moisture content. AI enables early evacuation, the deployment of flood mitigation measures, and the successful coordination of emergency response actions.
The European Flood Awareness System is another example of an AI-powered early warning system. The system combines data from weather forecasts, river levels, and soil moisture readings to deliver accurate flood forecasts and warnings throughout Europe. The approach allows authorities to take required actions ahead of time, such as evacuations and resource mobilization, to limit flood damage and ensure public safety.
4. Conservation and biodiversity protection
Conservation and biodiversity protection relate to initiatives aimed at maintaining and preserving the Earth’s natural ecosystems, species, and genetic diversity in the face of climate change. The conservation activities are enhanced and maximized when integrated with AI technologies, resulting in more successful conservation plans and actions.
The preservation of ecological balance, the protection of endangered species, and the maintenance of healthy and resilient ecosystems are all reasons why conservation and biodiversity protection are essential to society. Biodiversity is critical for ecosystem function, providing key services such as pollination, nutrient cycling, and pest control.
Climate change mitigation relies heavily on conservation and biodiversity protection. AI-powered technologies assist in the monitoring and management of protected areas, the identification of places of high ecological importance, and the evaluation of conservation activities. Conservationists design methods to protect biodiversity, maintain ecosystem services, and promote sustainable land use practices in the face of climate-related concerns by understanding the changes occurring in ecosystems.
There are numerous real-life examples of AI being used in conservation and biodiversity protection. For example, AI-powered image recognition systems detect and track endangered animals by analyzing camera trap photos or satellite footage. AI allows researchers to monitor and protect endangered populations more efficiently by automating the process of species identification.
A nonprofit organization called The Rainforest Connection uses AI-powered acoustic monitoring systems to protect endangered forests and wildlife. They install solar-powered devices loaded with AI algorithms that identify sounds of illicit operations such as logging, poaching, and deforestation in real time. The AI algorithms analyze the audio data to distinguish between natural and human sounds, alerting local authorities and conservation teams to hazards. The proactive approach allows for quick reactions to illegal operations, assisting in the preservation of biodiversity-rich habitats and the protection of endangered animals.
5. Data-driven policy making
Data-driven policymaking is the practice of using comprehensive and accurate data, coupled with AI technologies, to inform and shape policy decisions and strategies related to climate change. The practice involves the systematic collection, analysis, and interpretation of data to gain insights into climate trends, impacts, and mitigation measures, ultimately guiding evidence-based policy development.
Data-driven policymaking ensures that climate change strategies are founded on accurate and updated facts. Policymakers examine the viability of various policy alternatives, understand their effects, and identify the actions that have the biggest positive effects on lowering greenhouse gas emissions and advancing sustainability. They are made by AI-powered analysis. The strategy improves the effectiveness and efficiency of policy initiatives aimed at tackling climate change concerns.
A real-life example of Data-driven policy is the use of artificial intelligence to analyze socioeconomic and environmental data to identify vulnerable groups and places at high risk from climate change. Policymakers establish tailored policies and deploy resources to promote adaptation measures, boost resilience, and ensure equitable outcomes by knowing the individual vulnerabilities and impacts faced by different regions and populations.
The City of Copenhagen in Denmark utilizes AI to support data-driven policymaking in its efforts to become carbon-neutral. They analyze data on energy consumption, transportation patterns, and emissions to identify areas for improvement and develop targeted policies.
6. Sustainable urban planning
Sustainable urban planning is the discipline of designing and managing cities in a way that promotes environmental sustainability, resilience, and quality of life for citizens. The method involves incorporating sustainable development, climate adaptation, and mitigation principles into urban planning procedures to create cities that are ecologically harmless, socially inclusive, and economically vibrant.
Sustainable urban planning is essential because there are cities that face increasing pressures from population growth, resource shortage, and climate change impacts. Artificial intelligence-assisted planning allows the creation of communities that prioritize energy efficiency, sustainable mobility, green infrastructure, and resilience to climate-related dangers. Urban planners make knowledgeable decisions that encourage sustainable development, mitigate environmental consequences, and improve the general well-being of urban populations by utilizing AI.
Real-life examples of sustainable urban design that make use of AI and climate change data are already being applied in cities all over the world. One example is the application of AI algorithms to the analysis and optimization of transportation systems. AI analyzes huge amounts of data on traffic patterns, public transit usage, and emission levels to improve transportation efficiency, reduce congestion, and increase the use of sustainable forms of transportation, including cycling and public transportation.
Singapore’s Smart Nation is another real-life example, and its initiative mixes artificial intelligence with urban planning to develop sustainable and smart cities. AI algorithms evaluate data from sensors and infrastructure systems to optimize energy usage, control traffic flows, and improve waste management. The process allows the city to minimize its carbon footprint, enhance efficiency, and provide a better quality of life for its citizens.
7. Effective resource allocation
The process of optimizing the distribution and consumption of resources to minimize waste, maximize efficiency, and accomplish targeted climate change results is referred to as effective resource allocation. AI assists with resource identification, allocation, and management, resulting in increased sustainability and mitigation measures.
The effective allocation of resources is necessary for attaining sustainable development and reducing the effects of climate change. AI assists in cutting greenhouse gas emissions, minimizing the environmental footprint, and ensuring resource availability for future generations by optimizing the use of resources such as energy, water, and materials. Improved resource allocation helps to build a more sustainable and resilient society by maximizing resource utilization, reducing waste, and mitigating environmental impacts.
Real-life examples of successful resource allocation utilizing artificial intelligence are already being implemented in a variety of areas. Optimization of renewable energy deployment is one such example. AI examines geographical data, weather trends, and energy demand to determine the best locations for solar panels or wind turbines to be installed. Optimization increases energy-generating capacity while decreasing dependency on fossil fuels, resulting in a more sustainable energy mix.
The City of Los Angeles in the United States has deployed an AI-driven system to maximize water resource allocation and conservation. Water scarcity is a problem in the city as a result of extended droughts and rising water consumption. They examine data from multiple sources, such as weather trends, water usage patterns, and infrastructure problems, using AI algorithms to identify the potential for water saving and efficient distribution. The AI technology assists water management in detecting leaks, managing irrigation systems, and delivering real-time insights for improved decision-making. The strategy has resulted in huge water savings, less water waste, and better overall water resource management in the city.
8. Improved climate modeling and prediction
Improved climate modeling and prediction is the application of AI technology to improve the accuracy, precision, and efficiency of climate models, allowing for more trustworthy estimates of future climate conditions. AI evaluate large volumes of climate data, uncover complex patterns, and provide more exact predictions about climate trends, extreme events, and their potential repercussions by employing modern machine learning algorithms and data analysis tools.
Improved climate modeling and prediction provide insights into the potential regional and global implications of climate change. Artificial intelligence-based technologies allow scientists and policymakers to better comprehend the intricate interconnections within the climate system, assess the occurenceof catastrophic events, and devise mitigation and adaptation policies. The information is essential for developing sensible climate policy, putting resilience measures in place, and reducing the socioeconomic and environmental effects of climate change.
Real-life examples of AI-enhanced climate modeling and prediction are already demonstrating their use in a variety of applications. For example, AI-based climate models have been used to improve hurricane strength forecasts. AI algorithms create more accurate predictions of storm intensification and tracking by studying previous hurricane data, atmospheric conditions, and other pertinent elements. The Climate modeling and prediction allows for improved preparedness and reaction actions.
The European Centre for Medium-Range Weather Forecasts (ECMWF) employs AI approaches to improve weather and climate prediction. They use machine learning algorithms to evaluate past climate data and atmospheric patterns, allowing for more accurate predictions of extreme weather occurrences such as hurricanes and heat waves. The predictions assist in issuing timely warnings, enabling governments, organizations, and communities to take appropriate measures to protect lives and property.
Is using AI and Climate Change will make no error?
No, using AI in the context of climate change does not guarantee the absence of errors. AI systems are developed by humans and are subject to limitations, biases, and errors.
Several variables contribute to the risk of errors in AI applications for climate change. The quality and accuracy of the data utilized to train AI models are crucial elements. Unreliable predictions or suggestions result from the use of data that is inadequate, biased, or of poor quality. The complexity and uncertainty of climate systems present hurdles for AI models since they have the risk to fail to effectively represent all of the nuances and variations of climate patterns.
AI models are susceptible to changes in input data or assumptions, and they are prone to failing to account for unforeseen occurrences or nonlinear interactions within the climate system. AI system effectiveness is partly dependent on the algorithms and methodology used, which have limits or inherent biases.
Strict validation, testing, and model improvement are essential to reduce errors in AI applications for climate change. Human skills and subject knowledge must be used in conjunction with AI technologies to ensure a thorough grasp of the climate system and its intricacies. Regular monitoring, verification, and updating of AI models based on real-world observations and feedback are required to improve their accuracy and reliability.
What is the success rate of using AI and Climate Change?
The success rate of AI in climate change applications depends on ongoing model building, study, and improvement. AI algorithms must continually be validated, tested, and improved to improve performance and handle any potential biases or constraints. The effectiveness and success of AI applications in tackling climate change are determined by a variety of criteria, including the unique use case, data quality, model accuracy, and the complexity of the climatic concerns being addressed.
AI has shown promising potential in enhancing the understanding of climate patterns, improving renewable energy management, optimizing resource allocation, and aiding in climate modeling and prediction. The success rate varies based on the application and the difficulties being addressed. AI algorithms have shown better accuracy in predicting extreme weather occurrences such as hurricanes and droughts. The result is better preparedness and response measures to be implemented in sensitive areas. AI algorithms have helped maximize energy generation and storage in renewable energy management, enhancing overall efficiency and lowering reliance on fossil fuels.
It is crucial to recognize that AI is unable to solve every problem related to climate change, though. AI technologies, human skills, and policy interventions are needed to address climate system complexity, uncertainty in future projections, and the need for holistic approaches.
What are the risks of using AI and Climate Change?
Listed below are the risks of using AI and Climate Change.
- Insufficient data quality: AI systems rely primarily on high-quality data for accurate modeling and prediction. Inadequate or biased data result in incorrect outcomes and poor decision-making.
- Unintended consequences: Artificial intelligence models are capable of generating unanticipated results or activities that have a harmful impact on the environment or communities. It is due to faulty algorithms, a lack of contextual awareness, or unanticipated interactions within the complex climate system.
- Ethical considerations: AI presents ethical questions about privacy, responsibility, openness, and justice. The collection and use of personal or sensitive data for climate change applications must be controlled appropriately to respect individuals’ rights and avoid discriminatory behaviors.
- Overreliance on AI: Excessive reliance on AI systems without understanding their limitations or including human judgment leads to poor decision-making and ignoring of important socio-political, cultural, and economic elements in climate change.
- Technological limitations: Artificial intelligence systems are certain to have biases, uncertainties, and mistakes in their algorithms. The restrictions reduce the accuracy and efficacy of AI systems in simulating or forecasting complicated climate occurrences.
- Rebound effects: Adoption of AI technologies for climate change adaptation or mitigation occasionally result in unforeseen rebound consequences. For example, advances in energy efficiency through AI result in higher energy use in other areas, canceling out the intended benefits.
- Lack of interpretability: Deep learning techniques in particular are difficult to analyze or understand when used in AI models. The lack of interpretability hinders the understanding and trust in AI choices, particularly in climate change scenarios that require transparency and accountability.
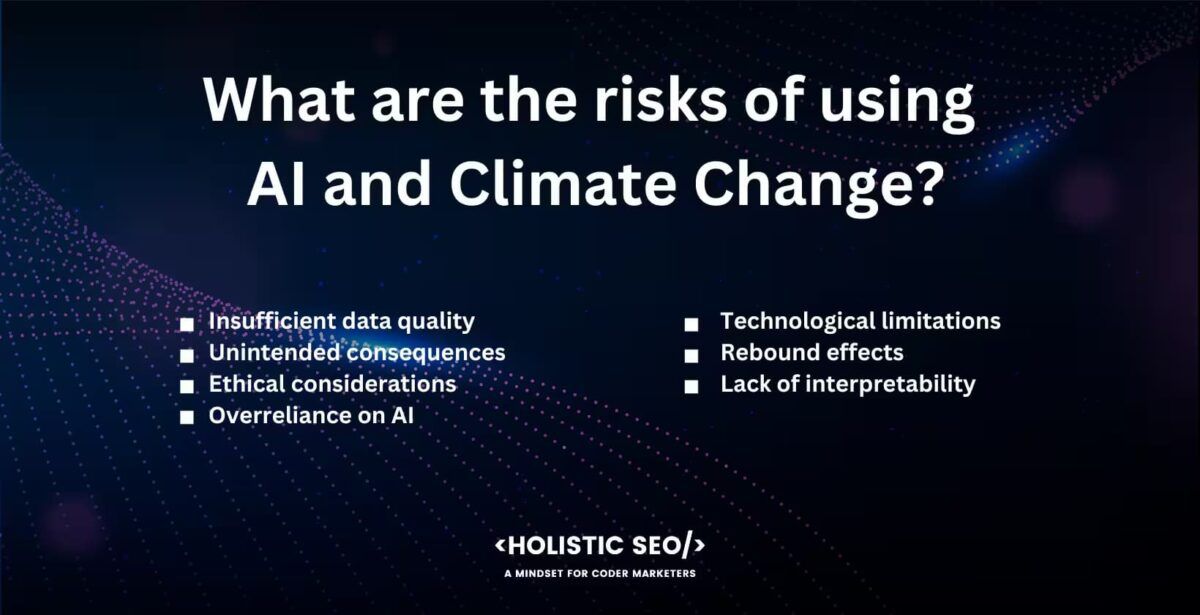
1. Insufficient data quality
Insufficient data quality refers to the inadequacy or poor quality of data utilized in AI applications for climate change. It implies that the data is not thorough, accurate, impartial, or relevant enough to properly develop AI models or produce accurate forecasts. Poor data quality compromises the effectiveness and dependability of AI systems, resulting in erroneous analysis and decision-making.
AI models need high-quality data to understand complicated climate trends, predict future events, and develop effective mitigation and adaptation methods. AI systems produce misleading or untrustworthy conclusions without accurate and complete data, making it difficult to make informed decisions and address climate change.
Real-life examples of applications of poor data quality are found in climate modeling and prediction. Incorrect or inadequate data inputs lead to inaccurate climate models, which lead to poor policy decisions and inefficient mitigation actions. Insufficient data quality in environmental monitoring, including temperature, precipitation, and air quality, reduces climate change estimates and hinders mitigation efforts.
2. Unintended consequences
Unintended consequences mean unforeseen and unintended outcomes that arise from the use of AI. The effects emerge when the implementation of AI technologies creates results or effects that were not anticipated or intended by the designers or users. Unintended consequences are significant because they have an influence on climate change mitigation and adaptation efforts. Understanding and resolving the consequences contribute to the responsible and effective use of AI in addressing climate change challenges.
AI systems make conclusions based solely on data patterns without regard for larger social, cultural, or economic factors. Unintended consequences include worsening current inequalities or negatively impacting certain communities. For example, an AI-based system used to optimize energy pricing mistakenly increase expenses for low-income households, putting a strain on vulnerable populations.
Real-life examples of unintended consequences in the application of AI and climate change are commonly found in automated decision-making processes. There is a risk of ignoring significant social, cultural, and ethical dimensions of climate change if AI systems are exclusively depended upon for essential decision-making without proper human oversight. Unintended consequences results in biased or unfair outcomes, a failure to understand local settings or a disregard for human values and preferences.
AI algorithms optimize processes to achieve certain goals, such as lowering energy usage or boosting efficiency. Unintended consequences emerge if environmental aspects are not properly considered. For example, an AI system developed to optimize transportation routes to reduce carbon emissions accidentally increase traffic congestion or causes ecological issues in specific places.
3. Ethical considerations
Ethical considerations in the context of employing AI for climate change refer to the moral and societal implications involved with the development, deployment, and effect of AI technology in tackling climate-related challenges. The factors cover a wide spectrum of moral values, such as equity, responsibility, fairness, and transparency. Understanding and addressing ethical concerns is critical to ensure responsible and fair use of AI.
The significance of ethical issues resides in guaranteeing that AI technologies are deployed in a way that is consistent with social values and norms and that they do not reinforce any existing prejudices or injustices. The creation and application of AI systems are guided by ethical principles and frameworks, which encourage equity, responsibility, and transparency.
AI-based systems are capable of analyzing enormous volumes of data to find environmental trends and judge the health of the ecosystem. Ethical issues surface, when it comes to data privacy and permission. It is crucial to ensure that privacy is respected and that consent is sought in accordance with ethical and legal norms whenever personal data is collected as part of the monitoring process.
Monitoring and reducing carbon emissions are just two real-life examples of ethical considerations in AI and climate change. AI-powered systems assist in tracking and analyzing emissions data to aid in climate action. Ethical considerations must be made when deciding how the data are collected, stored, and used. Maintaining public trust and respecting individuals’ rights requires ensuring privacy, data protection, and informed consent.
4. Overreliance on AI
Overreliance on AI for climate change refers to an excessive reliance on AI technology to address climate-related difficulties without recognizing their limitations or potential consequences. Overreliance on AI happens when AI is viewed as the solution to all climate change problems, detracting attention from other crucial components such as human judgment, expertise, and group decision-making procedures.
The importance of recognizing and correcting overreliance on AI rests in avoiding potential pitfalls and providing a comprehensive strategy for addressing climate change. AI is not a replacement for human judgment and is unable to replace the need for interdisciplinary collaboration, stakeholder participation, and socio-political issues. Recognizing AI’s limitations and creating a balance between its use and other methodologies is vital for effective decision-making and long-term solutions.
Key ethical, cultural, and political concerns are lost when human judgment is eliminated from the decision-making process while AI analyzes complex data and makes recommendations. Policy outcomes without diverse opinions and collective deliberation result in unforeseen repercussions and backlash.
The excessive dependence on climate models powered by AI for long-term forecasts is a real-life example of overreliance on AI. The models are inevitably restricted by the data that are trained on and the assumptions they contain, while AI improves climate modeling capabilities. Using AI models without taking into account other elements such as historical trends, professional knowledge, and uncertainties results in incorrect projections and poor policy choices. The incorporation of domain expertise and a thorough understanding of climate dynamics must be added to AI-driven modeling as a supplement.
5. Technological limitations
The inherent constraints and obstacles connected with AI technology are referred to as technological limitations in the context of employing AI for climate change. The constraints are caused by a variety of causes, including the complexity of climate systems, the availability of data, processing capacity, algorithmic constraints, and the uncertainty inherent in projecting future climate patterns.
Understanding technological limitations is crucial for controlling expectations and ensuring the responsible and successful use of AI in tackling climate change. Stakeholders make educated decisions, set realistic goals, and design strategies that harness AI while taking into account its inherent limits by identifying the limitations.
One real-life example of technological limitations in AI and climate change is the prediction and modeling of extreme weather events. The complexity of climate systems and the constraints in data quality and resolution make it difficult for AI to predict extreme weather events, despite the fact that it analyzes large volumes of previous climate data and patterns. Predicting the precise incidence, timing, and intensity of disasters such as storms, droughts, or floods remains difficult due to the dynamic and multidimensional nature of the phenomena.
The use of AI in carbon capture and storage technology is another example. The goal of CCS is to absorb and store CO2 emissions from power stations and industrial processes. AI improves the efficiency and effectiveness of CCS systems by streamlining the capture and storage procedures. Technological constraints such as the high prices of CCS infrastructure, limited storage capacity, and the technical feasibility of capturing emissions from many sources pose barriers to the widespread deployment and scalability of AI-driven CCS solutions.
6. Rebound effects
Rebound effects refer to the unforeseen repercussions or counterproductive outcomes that result from utilizing AI technologies to address climate concerns. The effects occur when the expected benefits of AI-driven solutions are outweighed or hindered by unforeseen negative implications elsewhere in the system.
Understanding rebound effects is critical because it allows people to think about the larger implications and potential trade-offs of AI applications in climate change mitigation and adaptation. Understanding unforeseen repercussions helps to avoid instances in which the benefits of AI deployment are overshadowed by undesirable consequences, resulting in suboptimal outcomes.
The adoption of energy-efficient technologies in buildings is one real-life example of rebound effects. AI has the potential to optimize energy use and efficiency, resulting in lower carbon emissions. The rebound effect happens when cost savings from energy efficiency lead to higher consumption or extra energy-intensive activities. Residents in energy-efficient buildings are more prone to use more appliances or equipment, potentially canceling out the energy savings realized through AI-driven optimization.
AI aid in the development of intelligent traffic management systems, routing algorithms, and self-driving vehicles, all of which improve fuel efficiency and lower pollution. The rebound effect occurs when the availability of AI-enabled transportation solutions leads to greater travel demand or longer trips. Emissions savings realized as a result of AI-driven gains in transportation efficiency outweighed by higher total travel and congestion.
7. Lack of interpretability
Lack of interpretability refers to the difficulty in understanding and describing how AI systems make decisions or arrive at specific outcomes in the context of climate change. It refers to the difficulty in understanding the underlying logic and reasoning behind AI-generated outputs, which hinder AI systems’ transparency, responsibility, and trustworthiness.
The importance of interpretability in AI and climate change lies in the need for stakeholders to understand and validate the outputs produced by AI models. Interpretability enables policymakers, scientists, and decision-makers to assess the reliability and accuracy of AI-driven predictions, recommendations, or policy suggestions. Interpretability helps identify any biases, errors, or limitations in the AI models, allowing for necessary improvements or adjustments to be made.
Climate modeling and prediction provide real-world instances of the lack of interpretability in AI and climate change. Climate models use complex algorithms and massive amounts of data to simulate and anticipate future weather trends. The aspects and variables influencing the model’s predictions are difficult to properly analyze and explain because of the inherent complexity of the models. The lack of interpretability makes it difficult for stakeholders to comprehend how certain forecasts or scenarios were created, and the underlying assumptions and uncertainties involved.
Climate risk assessment and prediction models are real-life examples of uses of lack of interpretability in AI and climate change. The models evaluate massive amounts of data and make predictions about future climatic situations using advanced algorithms and machine-learning approaches. Climate risk becomes difficult for stakeholders to comprehend and validate the aspects that contribute to the forecasts if the models are not interpretable, though. Lack of interpretability erodes the model’s credibility and adoption, impeding effective decision-making and action.
How does using AI and Climate Change affects the Environment?
The use of AI in the context of climate change has both beneficial and bad environmental consequences. The ultimate impact is determined by how artificial intelligence is deployed, managed, and incorporated into climate change mitigation and adaptation measures.
AI positively influence environmental management and conservation efforts by making them more effective and efficient. AI assists optimize resource allocation, improving renewable energy systems, improving waste management, and safeguarding biodiversity by analyzing massive amounts of data and discovering trends. AI programs scan satellite imagery to detect deforestation or monitor wildlife populations, allowing for targeted interventions that reduce environmental damage.
AI help with early warning systems for natural catastrophes, extreme weather occurrences, and climate change consequences through predictive modeling and forecasting. The proactive approach improves preparedness and responsiveness, potentially lowering the negative consequences on ecosystems and human populations. Optimization algorithms driven by AI help optimize transportation routes, lowering fuel usage and greenhouse gas emissions.
It is critical to understand that the employment of AI in climate change projects has detrimental environmental repercussions. AI systems necessitate a significant amount of computational resources, which result in higher energy usage and carbon footprints, particularly when deployed at large scales. The production and disposal of AI hardware contribute to electronic waste and other environmental concerns.
The dependence on AI lead to a human predisposition to completely transfer decision-making to algorithms without evaluation or consideration of ethical and environmental repercussions. The reliance on AI systems overlooks the value of human judgment, social dynamics, and the complexities of environmental concerns.
Adopting responsible AI practices is essential for ensuring the good impact of AI on climate change. The process involves taking into account the environmental consequences of AI technologies over their whole life cycle, supporting energy-efficient AI designs, and incorporating sustainability concepts into the development and deployment of AI systems. Ethical factors such as openness, fairness, and inclusion must be incorporated into AI design and decision-making procedures to avoid any unforeseen negative outcomes.
Yes, there are ethical concerns related to the use of AI in addressing climate change. Artificial Intelligence technologies become more incorporated into climate change mitigation and adaptation efforts, so it is essential to evaluate the ethical implications and potential risks connected with their use.
One key ethical worry is the risk of bias and discrimination in AI systems. Biases in training data or in the algorithms themselves preserve inequalities and disparities, especially among disadvantaged people who are disproportionately affected by climate change. Biased algorithms aggravate existing socioeconomic gaps if AI is used to allocate resources or determine which communities receive assistance during climate-related disasters.
Ethical considerations include transparency and interpretability. AI models are complicated, making it difficult to understand how they make judgments. The lack of interpretability raises concerns about accountability and equity. Trust is undermined and the capacity to protest or question those decisions is limited when judgments that affect individuals or communities are purely dependent on AI algorithms.
The chance of automated decision-making with AI without sufficient human monitoring is another ethical problem. AI must not take the place of human judgment and critical thinking, even though it improves decision-making processes. The wider social, cultural, and ethical components of climate change that call for human discussion and interaction get overlooked if decisions are made by AI systems. AI Ethics must be followed to guarantee that AI used to combat climate change respects human values, promotes openness, and includes human oversight in decision-making.
- 48 Online Shopping and Consumer Behavior Statistics, Facts and Trends - August 22, 2023
- B2B Marketing Statistics - August 22, 2023
- 38 Podcast Statistics, Facts, and Trends - August 22, 2023