AI in finance indicates the use of artificial intelligence in various transactions involving money and investments. AI in finance refers to the application of technology like machine learning or ML, which mimics human intelligence and decision-making. Its purpose is to improve how financial organizations evaluate, manage, invest, and secure money.
Artificial intelligence, or AI, is the term used to describe the creation of computer systems that are capable of doing activities that traditionally call for human intelligence. AI entails developing algorithms and models that give computers the ability to absorb and comprehend data, gain knowledge through experience, and arrive at conclusions or judgments.
The management, development, and study of money, investments, and other financial instruments are all included in the broad definition of finance. Finance entails the management of assets, liabilities, and risks in variousa variety of financial systems and the distribution of resources.
Financing is the practice of giving money or capital to people, companies, or enterprises. Financing entails securing the necessary financial resources to finance projects, investments, or operations, which is frequently accomplished using variousa variety of strategies, including loans, the issuance of bonds, the sale of stock, or the pursuit of venture capitalists or angel investors as sponsors.
AI is fundamentally altering how the financial services sector operates, and both the customers and the financial sectors benefitare benefiting from it. Financial service firms have new potential to boost their earnings with the help of AI in the finance sector.
What is AI in Finance?
AI in Finance pertains to the usage of tools such as machine learning (ML) which imitate humans’ cognitive and decision-making abilities to improve how financial organizations evaluate, control, allocate funds, and safeguard money.
The word “finance” refers to variousa variety of topics, including the development, management, and study of investments and financial assets. Finance entails employing potential revenue flows to finance current initiatives through the use of credit and debt, securities, and investment. Finance is strongly tied to the historical value of the currency, interest rates, and other associated subjects because of its temporal component.
Artificial intelligence (AI) technology is pervasive in the financial sector as it continues to advance. AI completely transforms how people handle money, from automating client service to spotting fraud and choosing investments. AI is altering the user experience by enabling quicker, contactless transactions with real-time credit approvals, better fraud protection, and cybersecurity.
AI has altered the financial industries’ viewpoint in terms of effectively utilizing data insights, designing an innovative company structure to boost company productivity, applying fresh dynamics, etc. Financial organizations have begun utilizing machine learning to identify and stop fraudulent transactions with real-time detections using an increased processing power and storage capacity brought by AI.
How does AI in Finance work?
AI in finance work by transforming how financial institutions evaluate data, automate procedures, and make choices. AI has redefined the financial sector by increasing its productivity, lowering risks, and offering better client experiences with the aid of cutting-edge algorithms and machine learning approaches. AI has the capability to manage large-scale data processing, automation, and intelligent decision-making.
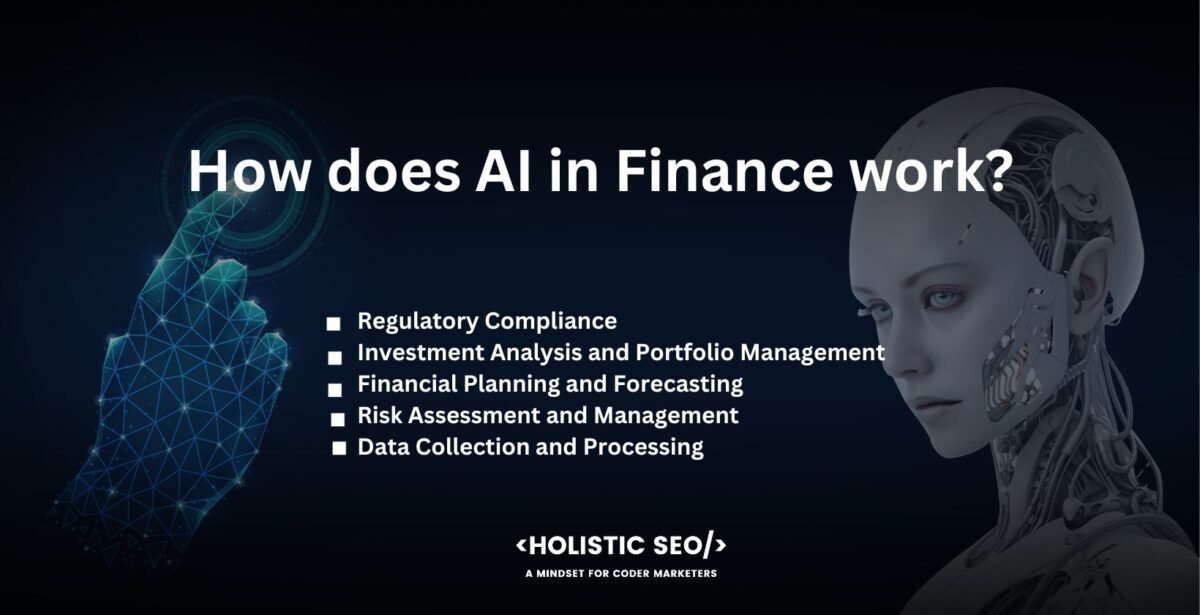
Listed below are the ways AI in finance work.
- Regulatory Compliance: Financial firms proactively handle regulatory requirements and prevent fines by using AI in finance. AI helps to examine massive amounts of information and discover potential breaches or non-compliant operations.
- Investment Analysis and Portfolio Management: AI analyzes historical data, market patterns, and news to find investment opportunities, evaluate risk-return profiles, and optimize portfolio allocation. AI aids investment professionals in decision-making, asset allocation optimization, and portfolio rebalancing in response to shifting market conditions.
- Financial Planning and Forecasting: AI algorithms evaluate massive amounts of financial data, economic indicators, and market trends to produce precise financial forecasts. AI algorithms enable organizations to plan budgets, set goals, and make strategic decisions as a result.
- Risk Assessment and Management: AI analyzes data trends, historical records, and market information to identify prospective hazards, such as credit defaults or market swings. It aids banking entities in monitoring and mitigating risks by offering early warnings, simulating situations, and enhancing the way risks are managed.
- Data Collection and Processing: AI speeds up data gathering and processing by automating data extraction, cleaning, and aggregation from multiple sources. It consolidates and organizes data in an organized format, allowing for better decision-making and data-driven insights, which decreases manual tasks, increases data accuracy, and enables efficient analysis.
1. Regulatory Compliance
Regulatory compliance is the use of AI algorithms and technologies to monitor, identify, and resolve potential breaches or non-compliant activities. Regulatory compliance works in accordance with regulatory norms and guidelines in the context of artificial intelligence for finance.
Regulatory Compliance is important for banking entities to maintain legal and ethical operations, safeguard customer interests, and come in accordance to the rules. It is to stay in business, avoid fines, and defend one’s reputation. Automation of compliance processes, improved monitoring capabilities, and increased effectiveness in recognizing and resolving compliance issues are all important contributions made by AI.
One example of regulatory compliance through AI in Finance is Anti-Money Laundering (AML). AI algorithms are capable of analyzing enormous volumes of transactional data, customer profiles, and outside data to find suspicious behaviors, patterns, and anomalies that indicate signs of money laundering. It assists financial institutions in adhering to AML requirements and in alerting the proper authorities to suspected fraudulent actions.
Know Your Client or KYC is another example of regulatory compliance. AI-powered solutions automate the authentication and screening of client identities by processing papers, extracting pertinent information, and comparing it to databases. AI models speed up customer onboarding procedures, ensure compliance with KYC standards, and improve the precision and effectiveness of identification verification.
2. Investment Analysis and Portfolio Management
Investment Analysis and Portfolio Management refers to the application of AI algorithms and models to the analysis of historical data, market patterns, and other pertinent elements. It is to assess investment potentialities, reach wise conclusions, and maximize asset allocation within a portfolio.
The importance of Investment Analysis and Portfolio Management lies in its use to maximize returns and minimize the risks that investors and financial institutions encounter in managing finance. Predictive modeling, pattern recognition, and advanced data analysis skills offered by AI in Finance enable more precise risk management, portfolio optimization, and investment decisions.
One example of Investment Analysis and Portfolio Management is Predictive Analysis. Artificial intelligence (AI) algorithms examine past financial data, market indicators, and macroeconomic factors to forecast future market trends and asset performance.
Another example where Investment Analysis and Portfolio Management are used is Algorithmic Trading. Algorithms carry out trades in accordance with predetermined rules or flexible methods using artificial intelligence. AI models are capable of spotting short-term trading opportunities and quickly executing trades by examining real-time market data, news moods, and historical patterns. AI increases trading efficiency and liquidity as a result.
3. Financial Planning and Forecasting
Financial planning and forecasting pertain to the use of AI algorithms and models to analyze historical financial data, macroeconomic variables, and market trends. It is to produce forecasts and projections relating to financial performance, earnings, expenses, and other financial metrics.
Financial planning and forecasting are essential for businesses and financial institutions to make wise decisions, set achievable goals, and allocate resources efficiently. AI improves such processes and produces more precise financial projections and strategic insights by enabling enhanced data analysis skills, pattern recognition, and predictive modeling.
One example of AI-aided Financial planning and forecasting is Revenue Prediction. AI algorithms anticipate future revenue by analyzing past sales data, market trends, consumer behavior, and other pertinent variables. Models based on artificial intelligence help organizations plan their sales targets, optimize pricing tactics, and allocate resources appropriately by taking fluctuations in demand, market circumstances, and other factors into account.
Cost optimization is another process that benefits from financial planning and forecasting guided by AI. AI is capable of finding areas for cost optimization by analyzing historical financial data, expense trends, and market developments. AI assists companies in streamlining operations, identifying ways to reduce costs, and forecasting upcoming expenses. For instance, AI models recommend the ideal inventory levels to save carrying costs while assuring a sufficient supply based on demand patterns.
4. Risk Assessment and Management
Risk assessment and Management refer to the application of AI algorithms and models to evaluate, analyze, and reduce risks related to financial transactions, investments, and market circumstances.
Risk Assessment and Management are vital for financial institutions to guard against potential losses, safeguard investor interests, and assure regulatory compliance. AI improves such procedures by providing more precise risk assessments, real-time monitoring, and proactive risk mitigation techniques.
Credit Risk Assessment is one example of Risk Assessment and management through AI. AI-powered algorithms are capable of analyzing transaction data, financial statements, credit profiles, and other pertinent information to determine creditworthiness and forecast default probabilities. AI models assist financial organizations in making informed decisions about the approval of loans, identification of risk costs, and determining credit limits by consideringtaking into account past data and patterns.
AI in finance works well with Fraud Detection. Artificial intelligence (AI) systems are capable of analyzing vast amounts of transactional data, consumer behavior, and outside data to spot correlations suggestive of fraudulent activity. Artificially intelligent models identify anomalies, strange behaviors, and fraudulent transactions by utilizing machine learning techniques. AI models allow financial institutions to act quickly to stop or lessen financial losses.
5. Data Collection and Processing
Data collection and Processing relate to the automated gathering, extraction, cleaning, and organizing of financial data from various sources so that it is ready for evaluation and decision-making.
Data collection and processing are crucial for banking entities to have access to accurate, current, and complete data which are going to be used for analysis, reporting, and selection. AI enhances such operations by using automation to obtain data, lowering human labor, enhancing information quality, and facilitating effective data analysis.
Automated Data Extraction is a good example of data collection and processing using AI. Artificial intelligence (AI) algorithms are capable of automatically extracting pertinent financial data from a variety of sources, including financial statements, market feeds, news articles, and regulatory filings. AI speeds up data collecting, lowers errors, and does away with the need for human data entry.
NLP or Natural Language Processing is another example of AI-empowered Data collection and processing. Most NLP approaches allow AI systems to process and evaluate unstructured financial data, such as that found in news stories, social media feeds, and analyst reports. NLP enhances users’ understanding of market dynamics by extracting useful insights, and analysis of sentiment and perceptions of the market.
What are the examples of AI in Finance?
AI in finance has brought rapid change in various sectors of the finance market. There are numerous examples of AI applications in finance across various areas. A few applications of AI in finance are observed in Customer Service, Portfolio Management, Algorithmic Trading, and Risk Management.
Consumer service powered by artificial intelligence makes use of Chatbots or virtual representatives. Chatbots are capable of providing answers to client questions. They offer personalized financial advice and automateautomates repetitive processes like creating new accounts or changing consumer information. For instance, the chatbot named “KAI” from Mastercard assists customers with account inquiries, transaction histories, and expenditure tracking. KAI offers customers individualized support and financial insights through a range of platforms, including SMS, WhatsApp, and Messenger. It utilizes machine learning algorithms and natural language processing to function as a customer representative does.
Portfolio Management is another illustration of the use of AI in finance. Portfolio management becomes more efficient through the help of artificial intelligence. AI evaluates enormous volumes of financial data and provides insights into trends, dangers, and potential avenues for investment. AI provides assistance to investors in making wise choices. One great example of a Portfolio management system powered by AI is the Wealthfront. Wealthfront is a robo-advisor that uses AI algorithms to manage investment portfolios for clients.
Wealthfront mixes conventional portfolio theory with artificial intelligence to build tailored investment portfolios for clients depending on their objectives, risk tolerance, and financial situation. The software automatically rebalances the portfolio while continuously tracking its progress when market circumstances and the client’s goals alter. Wealthfront is a popular choice for investors due to its AI-powered portfolio management, which enables personalized and effective investing strategies.
Algorithmic Trading is an example of AI in finance. Algorithmic trading is made more feasible since AI recognizes patterns, evaluates historical and current market trends, and forecasts future pricing. AI systems for Algorithmic trading carry out transactions in real-time while maximizing profits and optimizing investment plans using pre-programmed rules and conditions. Financial organizations and shareholders make decisions based on data and keep an edge in the intensely competitive world of trading withWith the aid of such a technology.
Risk Management is a great example of AI in finance. Artificial intelligence identifies potential dangers and foresees future situations by analyzing complicated financial data, offering insightful information that enables banks and other financial organizations to make well-informed decisions. BlackRock’s Aladdin platform is an illustration of risk management utilizing AI. The software helps investment managers evaluate and handle risks by looking at factors, including volatility in the markets, credit danger, and risk associated with liquidity. Aladdin’s risk management capabilities reduce the occurrenceoccurence of losses and increase returns, allowing investors to improve their investment techniques while making well-informed choices.
What are the latest breakthroughs in using AI in Finance?
There are various latest breakthroughs of AI in finance. LSome of the latest AI-powered finance discoveries include the XAI or Explainable AI, DRL or Deep Reinforcement Learning, Quantum Computing, and NLP or Natural Language Processing.
One of the most relevant technologies of AI in finance is XAI which stands for Explainable AI. The Explainable Artificial Intelligence (XAI) program was created in 2015 by the Defense Advanced Research Projects Agency or DARPA. The purpose of the program is to help end users better comprehend, believe in, and manage artificially intelligent systems. The goal of explainable AI (XAI) is to make AI models in finance easier to understand and more transparent. XAI gives financial organizations insights into risk evaluations, credit ratings, and investment suggestions, enabling them to comprehend the thinking behind AI-driven decisions. XAI promotes confidence and regulatory compliance by offering justifications for AI-based results. The four-year XAI research project got underway in 2017 and ended on year 2021.
Deep Reinforcement Learning refers to the various Artificial Neural Network layers that are used in the architecture to mimic how the human brain functions. Reinforcement Learning is basically a particular kind of machine learning algorithm where the machine learns to tackle a multi-level problem through trial and error. The optimization of trading strategies has shown potential using deep reinforcement learning or DRL. DRL algorithms make trade decisions, adjust to shifting market conditions, and optimize trading execution by learning from historical data and market dynamics. The strategy improves algorithmic trading performance by fusing deep learning with reinforcement learning.
Quantum Computing is a branch of quantum information science where the information is stored and processed using the principles of quantum physics. Classical computers use binary “bits” as their fundamental unit of information, but quantum computers use “qubits.” Numerous sectors, including the automotive, defense, financial, and pharmaceutical industries, are expected to benefit financially from advances brought by quantum computing. Users are enabled to create impenetrable encrypted communications networks, circumvent existing encryption techniques, and create extremely accurate sensors using such a procedure.
Quantum computing makes use of algorithms that tackle complicated optimization issues, risk assessments, and portfolio optimization more effectively. It is to enable quicker and more precise financial computations. The widespread use of quantum computing in banking and its practical applications are still in the early stages of development, yet changes banking in a massive way.
Natural Learning Processing or NLP is the field of computer science specifically associated with artificial intelligence (AI), which is concerned with providing computers the capacity to comprehend written and spoken words in a manner similar to that of humans. NLP blends statistical, machine learning, and deep learning models with computational linguistics or the rule-based modeling of human language. Computers are given the capacity to process human language in the form of text or audio data and fully understand what is being said or written, including the speaker’s or writer’s intentions and sentiments with the use of NLP.
AI systems are capable of evaluating and comprehending unstructured financial data, such as news stories, earnings reports, and social media sentiment, due to the development of NLP techniques in the banking industry. NLP improves market sentiment analysis, news-based trading methods, and decision-making by drawing insights from textual data.
It’s critical to remember that the latest breakthroughs in AI and developments in finance continue to improve quickly, and new innovations are anticipated.
What are the benefits of using AI in Finance?
The application of artificial intelligence (AI) in finance has transformed the financial services sector, from algorithmic trading that maximizes trade execution and profitability to tailored financial services that address specific needs. AI in finance boosts financial operations’ efficiency, security, and satisfaction among customers.
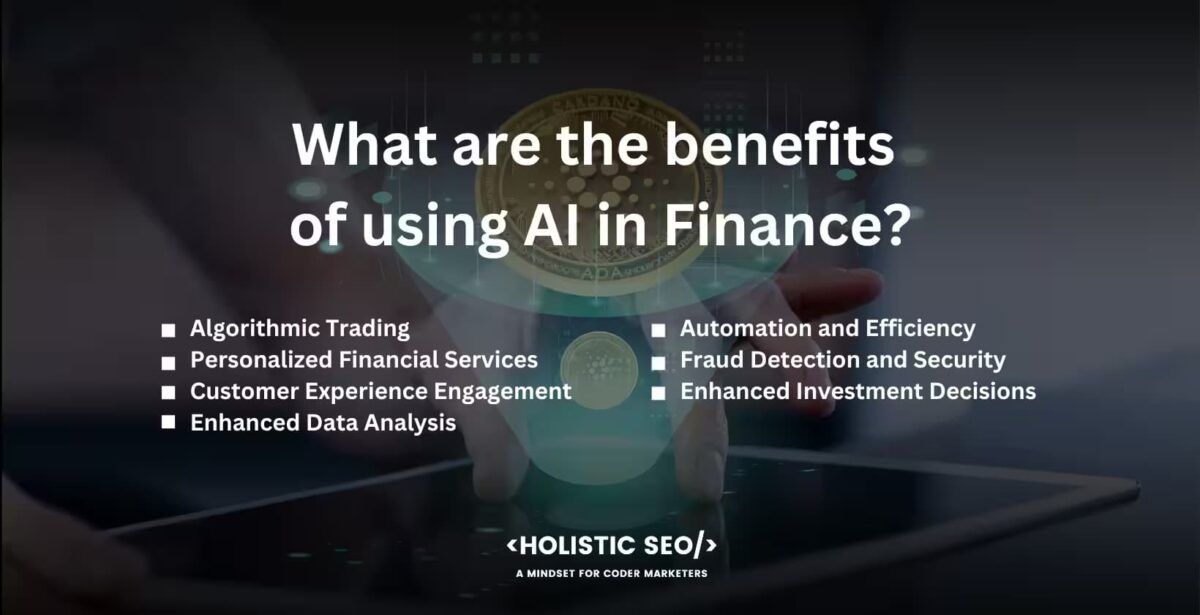
Listed below are the benefits of using AI in finance.
- Algorithmic Trading: AI in finance enables algorithmic trading by swiftly and accurately finding financial patterns, carrying out deals, and evaluating enormous volumes of financial inputs on a real-time basis. AI increases profitability and decreases human error in trading decisions as a result.
- Personalized Financial Services: AI-powered financial services assess customer data, including spending patterns and investing preferences. It is to provide individualized suggestions and personalized financial solutions which increase the client’s engagement and satisfaction with the financial process.
- Customer Experience Engagement: Financial organizations offer immediate and individualized customer service through the help of Artificial intelligence (AI)-based chatbots and virtual assistants. The chatbots in finance respond to users’ questions, facilitate transactions, and address difficulties whenever they arise during the course of the financial process. AI enhances the entire customer experience and engagement as a result.
- Enhanced Data Analysis: Large amounts of financial data are efficiently analyzed by AI algorithms. They are capable of spotting trends, correlations, and other information that people sometimes overlook. Financial forecasts, risk analysis, and strategic decision-making become more precise as a result.
- Automation and Efficiency: Data input, document processing, and regulatory compliance are just a few of the repetitive, time-consuming operations that AI automates. AI automation allows human resources to work on higher-value jobs and boost productivity and operational effectiveness.
- Fraud Detection and Security: Artificial intelligence (AI)-enabled systems have the ability to recognize abnormalities, trends, and unusual activity in a real-time manner. AI assists in the detection and prevention of fraudulent transactions, boosts financial security, and reduces potential losses.
- Enhanced Investment Decisions: AI algorithms are capable of examining previous market information, economic metrics, and other pertinent elements which produce data-driven investment insights and recommendations. Investors and fund managers make more knowledgeable and potentially successful investment decisions as a result.
1. Algorithmic Trading
Algorithmic Trading refers to the quick and accurate execution of trades in financial markets using AI and sophisticated algorithms. Numerous concurrent market data sets must be analyzed to recognize patterns and effortlessly execute transactions based on pre-established rules or strategies.
Algorithmic trading is otherwise known as automated trading, black-box trading, or algo-trading. The trading involves placing a deal using a computer program that adheres to a predetermined set of guidelines called an Algorithm. The deal produces profits at a pace and frequency that are beyond the capabilities of a human trader.
The importance of Algorithmic Trading lies in its ability to increase trade efficiency, lower transaction costs, and reduce human error. Algorithmic trading has grown in importance in the financial industry over the course of time. Trading opportunities are taken advantage of, and deals are executed quickly, which are not achieved when done manually.
High-Frequency Trading (HFT) is one application of algorithmic trading in the real world. HFT is an algorithmic trading technique that includes carrying out a lot of deals in a matter of milliseconds or even microseconds. HFT companies examine market data and carry out trades at breakneck rates using AI algorithms. The algorithms are made to find and take advantage of slight price disparities or market inefficiencies to make money through quick trading.
Smart Order Routing or SOR is another illustration of algo-trading. Brokerages and financial organizations utilize SOR, an algorithmic trading approach, to automatically route orders to the most advantageous exchanges or venues. AI algorithms assess market circumstances, liquidity, and order book data to choose the appropriate execution venue for trade. It ensures that the trade is executed at the best price and with the least amount of slippage conceivable.
Another real-time use of Algorithmic Trading is Statistical Arbitrage. It is a technique that aims to make money off of pricing irregularities or mispricings in financial instruments. Statistical correlations between connected assets are found via historical data analysis by AI algorithms. AI then executes trades based on departures from predicted values. The goal of such a technique is to spread out risk and maximize tiny rewards over a large number of trades.
2. Personalized Financial Services
Personalized financial services are those that adjust financial goods, suggestions, and assistance to each individual customer’s unique needs and preferences using AI algorithms and data analysis. Personalized financial services include evaluating consumer data, including spending patterns, earnings, and investment aspirations, to offer individualized financial solutions.
The importance of Personalized financial services lies in their ability to deliver more relevant and tailored offerings to customers which increases customer pleasure, engagement, and loyalty. Financial companies better satisfy the specific demands of each consumer and enhance their overall financial well-being by comprehending their wants and offering individualized solutions.
Robo-advisors are one of the practical instances of Personalized Financial Services. Online services known as robo-advisors offer automated portfolio management and investment advice based on user inputs and algorithms. They use AI algorithms to evaluate consumer risk tolerance, investment objectives, and market conditions to develop customized investment portfolios. A wider spectrum of investors is given the opportunity to access low-cost investment options provided by robo-advisors.
Another illustration of personalized financial services is Digital Financial Planning Tools. Financial planning solutions driven by AI examine user financial data and offer specific suggestions for saving, investing, and budgeting plans. The tools assist users in findingto find potential cost-saving opportunities, propose investments depending on their risk tolerance, and monitor their progress toward monetary objectives. Investment tracking tools that includelike Personal Capital and budgeting apps such aslike Mint are some examples of such helpful tools.
Personalized Credit Scoring illustrates a real-life use of Personalized Financial Services. AI systems evaluate a person’s creditworthiness by examining variousa variety of data, including credit history, financial transactions, and alternative data sources. It is normally done by examining variousa variety of data, including credit history, financial transactions, and alternative data sources. Lenders provide tailored loan terms, interest rates, and credit limits based on a more thorough analysis of a borrower’s financial status, increasing credit availability and streamlining the loan approval procedure.
3. Customer Experience and Engagement
Customer Experience Engagement describes the process of improving consumer involvement and interactions with financial entities through the use of AI technology. Customer experience involves utilizing AI-powered chatbots, virtual assistants, and personalized communication for seamless and customized client experiences.
The importance of Customer Experience and engagement is its ability to increase client fulfillment and participation, which aids the banking sector’s focus on client experience. Financial organizations improve client interactions, respond to inquiries quickly, and create a more convenient and effective customer experience by utilizing AI to deliver rapid and personalized help.
Virtual assistants and chatbots are effective illustrations of how user involvement and experience are applied in the real world. Numerous financial institutions use AI chatbots and virtual assistants to assist with transactions, answer client questions, and provide account information. They use machine learning and natural language processing to accurately interpret and reply to consumer inquiries. For instance, Erica, the virtual financial assistant at Bank of America, assists clients with bill payments, account queries, and advice on finances.
The voice assistants for financial services are another illustration. Financial services incorporate voice assistants like Apple’s Siri, Google Assistant, and Alexa from Amazon. Voice commands are used by customers to carry out actions, including like checking account balances, paying bills, or transferring money. Accurate voice recognition and interpretation are brought by AI algorithms, resulting in a seamless and user-friendly process.
4. Enhanced Data Analysis
Enhanced data analysis is the process of using artificial intelligence (AI) algorithms and techniques to process and analyze massive amounts of financial data more accurately, effectively, and quickly. Data analysis includes drawing important conclusions, patterns, and connections from data sets that are expectedly difficult for people to recognize and understand.
Data analysis is essential in the banking sector for making wise decisions, spotting trends, controlling risks, and increasing overall operational effectiveness. Financial companies improve productivity and gain a competitive edge by using AI-powered enhanced data analysis to find hidden trends, get deeper understanding, and make data-driven decisions.
One real-life example of Enhanced Data Analysis is Trading and Investment Analysis. Trading and investment methods make considerable use of AI-powered data analysis. A great deal of historical market information alongside economic indicators are processed by machine learning algorithms to find patterns, trends, and correlations that guide investing choices. Algorithmic traders create and backtest investing strategies on the Quantopian platform using AI and data analysis.
Predictive Analytics is another great example of Enhanced Data Analytics. Predictive Analysis forecast future market trends, consumer behavior, or financial results by evaluating previous data. It aids financial organizations in identifying pricing optimization options, prospective investment opportunities, and demand forecasting. Predictive analytics, for instance, is used by hedge funds and asset managers to estimate stock price changes and guide investment decisions.
5. Automation and Efficiency
Automation and efficiency in finance refer to the application of artificial intelligence (AI) technologies to automate tasks, lessen manual labor, and boost operational effectiveness. Automation involves integrating robotic process automation (RPA) and AI methods for automating routine tasks, increasing productivity, and optimizing workflows.
Automation and Efficiency are essential for the finance sector to handle massive amounts of data, boost precision, lower costs, and boost overall production. Financial organizations enhance efficiency and cut costs by automating repetitive work, freeing up human resources for more strategic endeavors, and streamlining operations.
One of the real-life use of Automation and Efficiency is Automated Document Processing. Information extraction and processing from documents like contracts, financial accounts, and invoices are automated using artificial intelligence algorithms. Artificial intelligence (AI) systems are capable of accurately extracting data using optical character recognition (OCR) and natural language processing (NLP). It decreases human labor and increases productivity in tasks such aslike data input and document processing. Businesses such aslike ABBYY offer AI-powered document processing alternatives for financial entities.
Compliance and Regulatory Reporting is another illustration of how Automation and Efficiency are applied to procedures in daily life. Artificial Intelligence automate compliance operations by tracking and analyzing massive amounts of data to detect potential regulatory infractions, highlight questionable activities, and guarantee compliance standards are followed. Manual compliance checks take less time and effort, and financial institutions fulfill their regulatory requirements better as a result.
6. Fraud Detection and Security
Fraud detection and security in finance refer to the application of AI technology to identify and prevent fraudulent acts. Fraud detection and security assureassures the integrity and safety of financial structures and operations. Detection of fraudfraus entails using AI algorithms to examine trends, anomalies, and behavioral data to identify and reduce the risk of fraud.
The importance of Fraud Detection and Security lies in its ability to secure consumer funds, uphold confidence, and defend financial systems. The finance sector must prioritize fraud prevention and security. Financial organizations identify fraudulent transactions in real time, reduce losses, and improve the overall security posture of their operations by using AI for fraud detection.
One of the real-life examples of Fraud Detection and Security is Biometric Authentication. AI-powered biometric authentication systems use methods that invlovelike voice recognition, fingerprint scanning, and facial recognition to confirm users’ identities when they access financial services. The systems add an extra layer of security by guaranteeing that secured access to sensitive financial information or protected conduct of transactions. Examples include banking apps for mobile devices that use fingerprint or face recognition for secure login and transaction authorization.
Behavioral Analytics is another example that illustrates the use of Fraud Detection and Security on a real-life basis. Behavioral Analytics is the process where AI systems evaluate client behavior, transaction patterns, and historical data to build profiles and spot outliers in behavior. Banking institutions proactively alert future fraud attempts by noticing questionable behavior or activity. Unauthorized account access, strange spending patterns, or attempts to manipulate financial systems are a few examples of what is included in behavioral analytics.
7. Enhanced Investment Decisions
The Enhanced investment decisions are the use of data analysis and AI technologies to optimize investment methods, improve the management of portfolios, and make more knowledgeable investment judgments. The use of AI algorithms is necessary to analyze market data, spot trends, evaluate risk, and produce investment recommendations.
Enhanced Investment Decisions are crucial in the finance sector as they offer insightful data to improve decision-making. Financial organizations enhance portfolio performance, lower risks, and increase returns for their clients by using AI for investment analysis.
Automated portfolio management is an illustration of how Enhanced Investment Decisions are used in a real setting. AI-powered platforms investigate huge amounts of market data, economic factors, and past performance to improve portfolio management. The platforms employ machine learning algorithms to reallocate assets, rebalance portfolios, and decide which investments to make in accordance with predetermined investment plans. Platforms like Wealthfront and Betterment, which provide automated investment management services, are two examples.
Another real-life example of Enhanced Investment Decisions is Sentiment analysis. AI systems review news stories, social media posts, and other information sources to determine how investors and market participants feel about particular assets or industries. Financial companies better understand market dynamics and make wiser investment selections by adding sentiment analysis to their investment decisions. Tools for sentiment analysis are frequently used by hedge funds and asset managers to understand market movements and investor sentiment.
Is trading using AI in finance make no error?
No, trading using AI in finance generates errors. It’s crucial to remember that trading platforms powered by AI do make mistakes occasionally.
AI-powered algorithms are being used by financial traders to quickly assess marketplace data, identify patterns, and make trading decisions. AI implementation has aided traders in making more profitable trades. Knowing how AI is changing the trading sector gives traders additional knowledge on how to increase productivity at minimal or no expense. Large-scale data processing, pattern recognition, and decision-making are all capabilities of AI systems.
Trading still generatesgenerate flaws or restrictions in AI-based trading systems. First off, the caliber and applicability of the data that AI algorithms are trained on determine how accurate they are going to be. Incomplete, skewed, or unrepresentative training data result in forecasts that are incorrect or trading decisions that are not optimal.
AI systems are vulnerable to unanticipated developments or changes in the market that are not covered by their training set of data. Artificial intelligence-driven trading techniques sometimes make mistakes as a result of unpredictable market volatility, unexpected news developments, or regulatory changes that deviate from predicted patterns.
Algorithms are still susceptible to human errors such as faulty assumptions made during the development stage, coding flaws, and parameter tuning problems, since they are created by people. The mistakes spread to trading choices and ledlead to unforeseen effects.
AI systems are susceptible to manipulation or hostile attacks. Malicious actors who want to influence the inputs or outputs of AI systems emerge and inject biases or inaccuracies into trading decisions.
It is crucial to regularly monitor and assess the performance of AI-based trading systems to minimize potential errors and guarantee the overall effectiveness and dependability of the trading methods. Putting risk management procedures into place, and employing human oversight is essential to help minimize such errors.
What is the success rate of investment decisions made by using AI in Finance?
The success rate of investment decisions made by using AI in Finance is challenging to quantify with a particular percentage. It is because the efficiency of AI-driven investment strategies depends on various factors, such as the accuracy of the data used, the level of sophistication of the AI algorithms used, the financial approach used, and the current state of the market.
AI is capable of offering insightful information, data analysis, and pattern recognition abilities that enhance financial decisions when used properly. Artificial intelligence (AI) systems have the ability to quickly evaluate massive amounts of information, recognize trends, and even find opportunities for investment that human analysts miss.
Investors and banking institutions typically employ a variety of criteria, such as risk-adjusted returns, performance consistency, and benchmark comparisons, to assess the success of AI-driven investment decisions. Human skills and judgment are paired with continuous tracking, review, and improvement of AI models to improve the results of investments.
What are the risks of using AI in Finance?
There are certain hazards that must be carefully considered in spite of the many advantages of using AI in banking. The risks must be managed for AI to be implemented in the financial sector responsibly and securely.
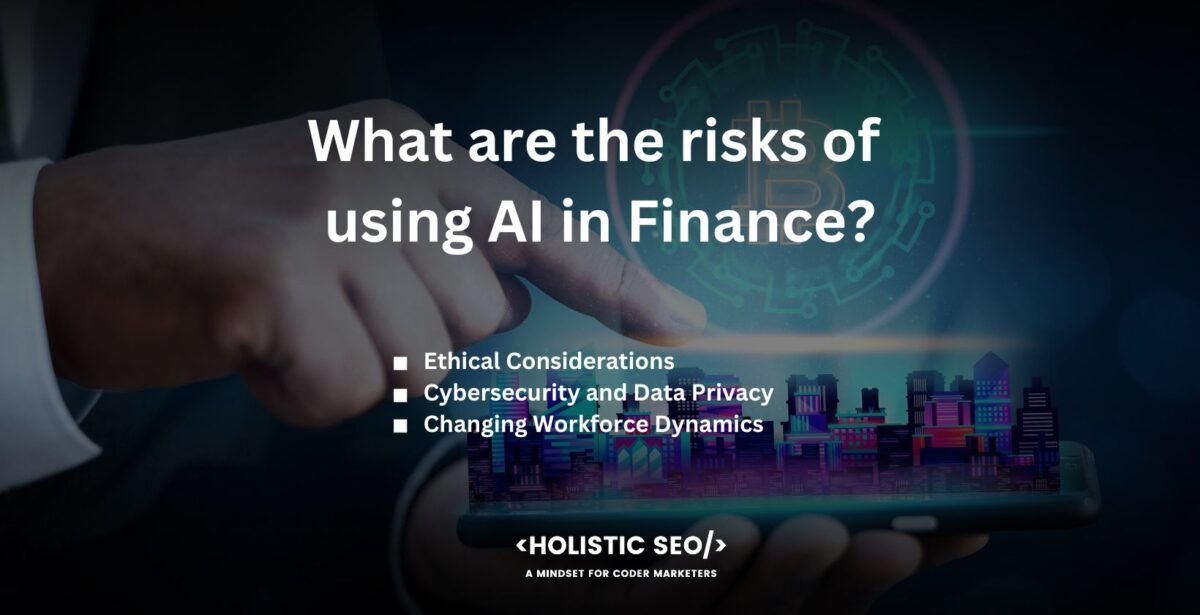
Listed below are the risks of using AI in Finance.
- Ethical Considerations: The application of AI in finance poses ethical questions about potential discrimination, lack of transparency, and prejudice in algorithmic decision-making. Artificial intelligence (AI) systems sometimes unintentionally reinforce biases found in the data they are trained on, resulting in incorrect results or discriminatory actions.
- Cybersecurity and Data Privacy: Financial organizations are more vulnerable to cybersecurity concerns and potential data privacy violations as a result of the rising use of AI in finance. AI systems are prime targets for assaults because they rely on enormous volumes of private data.
- Changing Workforce Dynamics: AI adoption in finance disrupts the labor market, causing job displacement and shifting skill needs. Some jobs become obsolete as a result of routine task automation. Changing workforce dynamics necessitates skill upgrades or job transitions into other occupations that make use of AI technologies.
1. Ethical Considerations
The application of AI in banking creates ethical problems, due to potential biases, a lack of transparency, and discriminatory effects in algorithmic decision-making. It is critical to address such risks to ensure the ethical and responsible use of AI technology in finance.
Ethical considerations are of the utmost significance as AI-driven finance affects people, businesses, and society as a whole. Algorithms that are biased produce unfair results, prejudice, and exclusion. It is crucial to address ethical problems and improve openness in algorithmic decision-making in order to retain public confidence in AI-based financial systems.
Bias in lending decisions is one example of a danger related to ethical considerations in financial organizations. Artificial intelligence (AI) algorithms employed in lending choices unintentionally integrate past data biases, producing discriminatory results. For instance, loan applications from particular demographic groups are disproportionately rejected if a lending algorithm heavily depends on old data that shows biased lending practices.
It maintains systemic prejudices and inequities along the way.
Market manipulation and algorithmic trading are two examples of dangers that raise ethical questions. Concerns about market manipulation are raised by the use of AI in algorithmic trading. Sophisticated trading algorithms have the ability to disrupt markets or provide traders unfair advantages by taking advantage of market conditions or misleading other market participants.
It is necessary to create strong standards and frameworks for algorithmic accountability, transparency, and fairness in Exploring Ethical Considerations in AI. Data governance procedures are used to guarantee varied and representative information sets. AI systems are routinely checked for biases, and regulatory monitoring is used to encourage ethical and equitable usage of AI technology in finance.
2. Cybersecurity and Data Privacy
The risks associated with data privacy violations and cybersecurity breaches are introduced by the usage of AI in banking. The dangers are brought on by the massive volumes of private financial information involved and the potential weaknesses of AI systems. It is essential to manage such threats to safeguard private data, uphold trust, and prevent illegal access or data misuse.
The importance of Cybersecurity and Data privacy lies in its ability to protect client data, stop financial fraud, and maintain the integrity of financial systems. Financial data breaches or unauthorized access cause large financial losses, reputational harm, and weakened client confidence. Compliance with data privacy rules is crucial to safeguard individual privacy rights and maintain reliableresponsible data management methods.
The risks connected to data privacy and cybersecurity include Breaches of Data. Financial firms that employ AI systems are susceptible to hacks intended to access confidential financial information. A recent example is the Equifax data breach, which occurred in 2017. Hackers took advantage of flaws in the company’s systems to access the personal and financial information of millions of customers, which had serious financial and reputational repercussions.
Another example of the dangers associated with data privacy and cybersecurity is unauthorized access to AI systems. AI systems themselves are susceptible to intrusion or manipulation, which result in the misuse of financial data or the interruption of business activities. For example, a cryptocurrency exchange’s AI-driven trading system was compromised by a hacker in 2020, who used it to manipulate prices and cause big losses for traders.
Implementing strong security measures, such as encryption, access controls, safe data storage, and regular system audits, are necessary to address issues pertaining to AI in Cybersecurity and data privacy. Banking organizations must follow laws governing data privacy, get informed consent for data use, and set up stringent procedures for handling and sharing data.
3. Changing Workforce Dynamics
Risks associated with shifting workforce dynamics, including job displacement, skills gaps, and the need for workforce adaptability, are associated with the usage of AI in finance. It has an impact on the duties and roles of human workers, as AI technology automates some operations. A reorganization of the workforce and the need for upskilling or transitioning into new roles become a necessity as a result.
It’s critical to comprehend and manage shifting workforce dynamics to enable a smooth transition and assist staff in adjusting to the changing environment. Financial providers need to address the potential effects on employment, support upgrading programs, and provide chances for staff to use their knowledge of AI technology in tandem with one another.
Job displacement is one example of a danger brought on by shifting workforce dynamics. Some mundane and repetitive jobs that were previously carried out by human personnel are replaced by AI automation. For instance, automated chatbots and virtual assistants take the place of customer service agents for straightforward questions, eliminating the need for human participation and eliminating jobs in such positions.
Another example of a risk related to shifting worker dynamics is the need for upskilling and reskilling. Employees must develop new skills and competencies to efficiently use AI technologies when the industry adopts them. Critical skills include data analysis, programming, AI algorithm creation, and ethical issues forFor those working in positions requiring data-driven decision-making or managing AI systems, for instance.
How does using AI in Finance affect the workforce in Finance industry?
The use of AI in the finance sector has big effects on the workforce, changing dynamics and positions within the industry. AI changes the nature of the labor’s skillsets, which calls for workforce upskilling. Certain repetitive and rule-based operations that have traditionally been carried out by humans, such as data input, document processing, and basic customer care, are automated by AI. People in such positions lose their jobs as a result of automation.
Another thing is that it creates opportunities for workers to advance into more strategic and difficult roles that make use of AI technology. Data analysis, algorithm building, risk assessment, and decision-making based on AI-driven insights are all components of such roles.
Individuals in the finance industry must develop new skills and knowledge toin order to adapt to the shifting workforce dynamics. Financial companies have a duty to fund training and upskilling initiatives to assist their staff in such a shift. Collaboration between humans and AI systems becomes crucial.
The effective implementation of AI in the workforce necessitates a proactive strategy for upskilling, encouraging collaboration between humans and AI, and managing the transition successfully.
What financial institutions use AI in Finance?
There are severala number of financial organizations that have integrated AI into their daily operations. AI is being more widely used in finance as businesses become more aware of its potential advantages in terms of improved decision-making, risk management, customer engagement, and operational efficiency. Financial organizations make use of enormous quantities of data, automate procedures, and gather insightful information for an edge in the quickly changing financial environment of today through the implementation of AI.
One of the financial organizations that use Ai in their finance section is Enova. Enova leverages AI and machine learning in its lending platform to deliver sophisticated financial analytics and credit evaluation. Non-prime consumers and small enterprises address issues like emergency expenses and access to bank loans for their operations without putting themselves or their lenders in an unfavorable position, with its assistance.
Software for processing documents that combines machine learning and human verification is available from Ocrolus. Ocrolus is a financial institution that makes use of AI in handling financial matters. People analyze financial documents more quickly and accurately by using software, businesses, and organizations. With a focus on mortgage lending, business lending, consumer lending, credit scoring, and KYC, Ocrolus’ software examines bank records, pay stubs, tax documents, mortgage forms, invoices, and more to evaluate loan eligibility.
DataRobot is another financial institution that employs AI in its finance through machine learning software. DataRobot enables financial institutions and businesses to construct precise predictive models quickly when making decisions about things like fraudulent credit card transactions, digital wealth management, direct marketing, blockchain, lending, and other things. Alternative lending companies are enabled to forecast which consumers are to default, improving the accuracy of their underwriting judgments with the help of DataRobot’s software.
- 48 Online Shopping and Consumer Behavior Statistics, Facts and Trends - August 22, 2023
- B2B Marketing Statistics - August 22, 2023
- 38 Podcast Statistics, Facts, and Trends - August 22, 2023