Lexical Semantics (lexicosemantics) is the relationship of words to each other. Lexical Semantics include different types of word relations such as meronyms, holonyms, antonyms, synonyms, hypernyms, and hyponyms. Semantic Similarity means the closeness and relevance between two words. Semantic relations between words (lexical semantics) and distance between words’ meanings (semantic closeness) are used by semantic search engines with Natural Language Processing and Natural Language Understanding.
Lexical Semantics and Semantic Similarity (Closeness) are used to understand the Main Context and Micro-contexts within a document. Search Intent Coverage, Related Search Activity Prediction, Query Anchor, and Query Completion Predictions are affected by the word’s association with another word within semantics.
In this Lexical Semantics and Semantic Closeness Guide and SEO Case Study, the definitions of the related concepts, their usage methodologies, and some concrete-early SEO effects will be demonstrated.
Before continuing further, let me introduce you to myself. I am Koray Tuğberk GÜBÜR, the Owner and Founder of Holistic SEO and Digital. In this article, I will try to fill some gaps between my previous SEO Case Studies with the Lexical Semantics and Semantic Relevance. From my Semantic SEO Course, and the other guides and tutorials, you can learn more about my SEO Case Studies.
The Importance of Lexical Relations for SEO Case Study with 30 different Websites have been written one year ago. It is not published to protect some websites. The active start and end dates of the projects are given transparently. The organic search performance after the active working end date comes from the search engines’ historical data, trust that is caused by the quality increase of the SEO campaign. Every project had at least 80 content briefs before the active project management’s end date.
All the projects below are accepted by Holistic SEO & Digital for solely SEO Case Study creation and publication to prepare the SEO Culture and Industry for the incoming Semantic SEO Course. An NDA is signed with only Kanbanize.com, thus there is no GSC Data.
To join the Semantic SEO Course, click the image below, and subscribe to the Holistic SEO Newsletter.
Some of the Topical Maps, Semantic Content Network Designs, and Content Briefs for these websites and projects are shared in the incoming Semantic SEO Course lectures for educational purposes.
The safety of the projects on the website is guaranteed by waiting 1 year for the publication of the case study.
Some of the case study subject websites are processed in incoming other case studies, and books with different angles in holistic SEO with different contexts, and SEO practices.
- Importance of Information Responsiveness (Book)
- Importance of Cost-of-Retrieval (Book)
- B2B SEO Case Study
- SaaS SEO Case Study
- Expanding a Topical Map SEO Case Study
These SEO Case Studies and Books will be published in 2022, and early 2023. The lexical semantics video explains the lexicosemantics for SEO, and linguistics with 26 projects.
Background of the Semantic Relevance, Similarity, and Lexical Semantics SEO Case Study
In this Lexical Semantics and Semantic Relevance-focused SEO Case Study, I will demonstrate 6 different websites. To protect the clients, I won’t be able to give the name of all websites. But you will be able to see the effectiveness of understanding the Lexical Relations, Semantic Similarity, and Relevance between words for SEO.
Some of these SEO Projects are newly launched, and some of them are still active. I will demonstrate the changes and methods and concepts behind these changes.
- The Methodology Summary can be found below.
- Understanding the Distance between Words as Vectors.
- Creating the sentence structures for the questions and the answers.
- Matching the answers and the questions to sharpen the context.
- Using accurate information with different forms and connections.
First Website with Lexical Relations and Semantic Relevance and Similarity for SEO
TeamColorCodes is the first website that will be explained over Lexical Semantics and Semantic Similarity SEO Case Study. Lexical relations of words and semantic relevance, and distinctiveness, become more prominent if a website contains too many entities of the same type. Since all the attributes are the same, and to decrease the content production cost, since most SEOs use Programmatic SEO, it requires using a “Structured Language Model” with lists, tables, and of course, ads. In TeamColorCodes, to increase the Information Retrieval Score, and Information Extraction Responsiveness, the Lexical Relations, Semantic Relevance, and Distinctiveness between the words, concepts, and entities are used.
“Working with Koray on Teamcolorcodes has been a great experience so far. It’s a lot of work to get everything right and do it ‘the Koray way’, but the results are there, so it’s all worth it. The focus of this project has been completed on semantic content, and we’ve edited many pages.
We monetize Teamcolorcodes.com with ads and one very nice side effect we’ve seen is that the RPM of the pages we applied Koray’s strategies to, increased by a lot because of all the extra content that was added to the pages (more content = of course, more room for ads).”
Paul Jensen
We hope to continue working with Koray and improve the site even more.”
Below, you can find the graphic of the Google Search Console for the last 6 months’ performance change.
To achieve this specific increase, the most relevant attributes for the entities are chosen, proper questions are generated, and answers. Multiple content formats and language models are placed and integrated. The query count, and the impressions, along with the clicks are increased properly. The Contextual Search SEO Case Study is an A/B Test of the specific results.
The last 3 months’ comparison can be seen above. Below, there is a screenshot from the client. The pages that are adjusted and optimized with Semantics had a lower exit rate with longer session durations and conversions.
A quick 3 months of jumps can be seen below from the Ahrefs.
The screenshot below was taken 3 months later than the previous screenshots. The increase continued, and big impression jumps started with re-ranking events. The last 7 months’ comparison can be seen below.
TeamColorCodes.com has benefited from the Query-Document-Intent templates that have been processed in the Semantic Content Networks SEO Case Study.
This is an SEO A/B Test sample from TeamColorCodes.com with Semantic SEO. You can also create new web page samples with new content templates to see how a search engine perceives your new content template.
The lines above were written 9 months ago. The updated version of Teamcolorcodes.com’s organic traffic change is below.
A further 18% organic click increase, and a 98% organic query count increase happened. Despite the lost queries in 4-10 and 1-3, the total traffic and topical coverage increased. Most of the lost clicks or queries are from company-related information, rather than the company’s color-related attributes. Thus, Google consolidated the relevance by constructing new indexes, and related query networks with lexical semantics in entity-oriented search.
vinjected e-commerce web pages by a security breach. This is a note to show the prominence of solid semantically optimized relevance and responsiveness for content networks.
Second Website with Lexical Relations and Semantic Relevance and Similarity for SEO
The second website is a project that has been started based on recipes for the same theme and context. To keep SEO growth fast and economical, publication frequency has been increased with the root and prominent attributes of the recipe objects. The internal links and main questions are determined based on the popularity of entities. Taxonomy and ontology are used before creating the first articles.
Below, you can see the first 3 months’ graphic of the second lexical relations website sample.
This screenshot is from 6 months ago.
The second website has produced more content items, and the first and last three months’ comparison difference can be seen for the growth.
The jump in traffic can be seen below the second website.
From the SEMrush, you can see the jump.
The second website has benefited from the momentum of publication with a high update rate, and proper-clear sentence structures along with Recipe Schema Markup for initial ranking. During this time, most of the “Bar-related” equipment, recipes, and facts are processed. Later, the project owner leveraged the same process with semantically relevant questions and answer pairs with higher publication frequency.
The Project Owner is one of the best operators that I have ever met. Thus, they are able to consolidate a high level of understanding into a low level ofa cost but high level of efficiency with proper implementation. Semantic SEO with Question and Answer Pairs can help a website to be a quick authority source as below.
You can see the latest results below.
The screenshot has been taken 6 months later than the previous ones. Below, you can see the last 28 days of the difference.
Below, you can see the last 6 months of comparison.
Below, you can see the Ahrefs results.
Below, you can see the SEMrush results.
“As an entrepreneur who innovates the SEO, Koray Tuğberk GÜBÜR is one of the rare people that I can connect with and trust, truly. Talking about Search Engines, and their decision trees, and creating new SEO strategies are some of the fun activities that you can do with Koray. It is good to have brave SEO methods in the mainstream SEO media too.”
- From Israel with Love
Third Website with Lexical Relations and Semantic Relevance and Similarity for SEO
The third website is SunnyValley.io. SunnyValley.io is a SaaS company that focuses on Network Security and Firewall services. Thus, to provide a better Lexical Relation advantage with Semantic Relevance and Similarity, most of the network security topics are processed. This is still an ongoing project with branding and branded query increase campaigns.
For those types of projects, a small topical map and initial semantic content network creation, and then deepening the context from a single topical map node are better. For this project, that context-deepening point is “Firewall types” and “Advanced Next-gen Network Security”. This phase is still ongoing.
“As a SaSS and Next-Generation Firewall Company, network security is the priority topic for Sunnyvalley.io. During our Semantic SEO Project, we changed our product name to ZENARMOR. We have cleaned many unnecessary web pages and dealt with technical SEO and UX improvements from SEO first perspective. We have trained our authors and followed a topical map and content briefs that have been prepared. After a baseline, we have started to focus on the brand-entity value of Sunnyvalley.io by deepening our content network for specific sections.“
Murat Balaban,
Owner of Sunnyvalley.io
Below, you can see the progress and re-ranking event after having enough levels of the quality signal dataset for Sunnyvalley.io from Ahrefs.
Below, you can see the specific re-ranking event from the GSC Data.
SEMrush’s data for Sunnyvalley.io is below. It is a rare situation that, Ahrefs calculates the total traffic amount better than SEMrush.
The Sunnyvalley.io project will be explained in the SaaS SEO guide later. But basically, for SaaS companies, topical maps, coverage, and a proper semantic content network are necessary to create a proper topical authority. The entity-oriented search SEO Case Study is useful to understand the Sunnyvalley.io-like websites’ relevance to Semantic SEO. Because, if a company is a service provider as an entity, Google doesn’t cluster it together with other entities from the same industry. It means that the company is not being recognized properly by Google. But, if the company is a specific entity from the industry, it shows that the entity is recognized, classified, and also ranked for certain categorical queries.
In this context, the “comparison” and “alternative” related articles are written. From a local search point of view, the company is connected to other closely related security and network-related entities. From an open web perspective, it is compared to other cybersecurity projects.
The current situation of the project from Ahrefs is below.
The current situation of the project from SEMrush is below.
The naked Google Search Console Data is below.
The Sunnyvalley.io is processed in the SaaS SEO Case Study projects for helping new startups.
Fourth Website with Lexical Relations and Semantic Relevance and Similarity for SEO
This is another project that I will publish later. But, I wanted to include it here regarding the “re-ranking”. In the Google Ranking Algorithms SEO Case Study, I have explained this perspective in the context of “re-evaluation of a source for quality”. DogFoodCare will have more than 10,000 clicks per day in the future. I said this for Vizem.net, in one of my emails as below, just check.
And, this is the updated version.
And this is the nearly two months later version.
Actually, it takes more than 10,000 clicks a day at the moment. The same situation will also happen for DogFoodCare.com after the initial re-ranking algorithms.
The hard part of this project is onboarding the authors with a true educational session series. We have written 20 articles in 3 months and published them all at the same time. These 20 articles are more quality than the competitors. They have the critical entities within them as the main entity, and they have triggered a re-evaluation for the specific source. With the 20 semantically engineered articles, we have gained more than 20,000 new queries. And, clicks have increased by 100%, while impressions have increased by 300%.
You can see the increased comparison below. There are 30 days between these two screenshots.
If this website completes the topical map and semantic content network, as I say, during the fundamental Google Broad Core Algorithm Updates, it can gather millions of organic traffic per month.
One more note, if you read the Topical Authority SEO Case Study, the “Neighborhood Content” concept is relevant to this project. The query gains came from the newly published content, but the click increases came from the old content. The old content ranked better due to the newly published quality content. Because new content doesn’t have enough historical data or coverage for its own focused entity groups, they increase the overall quality of the source. And, it helps for further clicks for the more “rankable” website sections.
This last note will be explained with “relevance attribution” and “radius” in a later SEO Case Study.
Fifth Website with Lexical Relations and Semantic Relevance and Similarity for SEO
Kanbanze.com is one of the most friendly companies to work with. I am glad that I have encountered these friendly team members from Bulgaria. At least 50% of the time, international clients do not have a long-term partnership with the spirit, which prevents me from performing a proper SEO Case Study or publishing the results. But, Kanbanize.com is a friendly and honest cooperative that I want to thank for their cooperation and tidy manner.
“Koray’s expertise is a mix of hardcore SEO topics, in-depth technical knowledge, and a vast experience in various industries. He’s very diligent and pedantic about the task to be delivered and has definitely raised the bar high. On top of that, he’s a nice and pleasant person to work with. Highly recommended!”
Dimitar Karaivanov
During the Kanbanize.com SEO Project, many training sessions were performed with advanced SEO specifics for content writing or technical SEO.
“Koray’s technical SEO expertise has been a great asset to our team. He helped us dive deep into SEO and really understand some advanced topics, which have been very helpful for our organic performance. He’s always open to answering questions and providing advice for handling complex issues that can elevate anyone’s SEO performance!”
Nikolay Tsonev
“Koray is one of the best SEO experts I’ve ever met. His knowledge and expertise in the field are beyond the boundaries of traditional SEO practices. Koray goes deeper than usual in every aspect of SEO science including, semantics, keyword research, article structure, technical SEO, and others.”
Pavel Naidenov
With Kanbanize.com, we have worked for around 6 months. During these processes, we have created a semantic content network to complete the specific “gaps” between the entity-oriented topical graph. We have prioritized “Agile” and “Production Efficiency for teams”, and anything related to the “Kanban Boards”. We have focused on the machines’ methods to perceive a language and, we have performed proper declarations and consistent definitions across the semantic content network. Since we were completing an existing content network, sometimes we have revised the old content. Sometimes we have internally linked and bonded both sections to support each other.
“Koray has been of great help to us with his technical expertise on SEO and guidance on expanding our content topical reach. What I liked the most is the openness and willingness to share knowledge and experience in every possible way – training, case studies, recorded sessions, valuable suggestions, and more. The working process was tailor-made to suit us and to facilitate communication, and our feedback and questions were always addressed. I’m glad I had the opportunity to work with Koray and his team.”
Iva Marinova
The lines above were written nearly 9 months ago. You can see the updated version of the website below.
During this time, organic traffic increased a further 54%. The 4-10 query count increased to 28.9%. The top 3 ranking query count increased by 27.1%. Kanbanize.com continues to implement the topical map and semantic content network design with the SOPs and training of Holistic SEO & Digital for Semantic and Technical SEO. Before leaving the project, more than 80 content briefs, and certain principles are provided to the content production team. The current situation of the company’s growth is visible from the Ahrefs graphic below.
The increased organic growth of the subject website with semantic SEO, and lexical relations’ relevance contribution is visible via the SEMrush graphic below.
Sixth Website with Lexical Relations and Semantic Relevance and Similarity for SEO
The last subject website for explaining the prominence of Lexical Relations for SEO and content communication is VSSMonitoring.com. VSSMonitoring.com is handled similarly to Kanbanize.com. The project is accepted for the purpose of publication of an SEO Case Study while providing a topical map, semantic content network design, and training along with the content briefs for future publications. The project is managed actively between June 2021 and January 2022. After January 2022, the project continued to implement the same principles with a higher content publication frequency.
The sitemap update history for the company is visible above. During the active project management of the company, most of the website is updated, and new content is published based on semantic SEO principles and methodologies.
Some of the results that are acquired for the project are below.
- 3200 New Top 3 Queries, %391 Increase
- 8236 New 4-10 Ranking Queries, %173 Increase
- 53,000 New 10-100 Ranking Queries, %99 Increase
- 100% Organic Click Increase, %600 Organic Impression Increase
The start, end, and latest search updates’ effects are visible below.
VSSMonitoring.com is an affiliate website that focuses on monitors mainly. The topical map that is created focused on monitor types, monitor comparisons, and monitor-related informative concepts. After the end date, the teachings and content briefs that are created are continued to be used.
VSSMonitoring.com GSC result screenshots from the timeline that is worked together actively.
6 months GSC Organic search performance comparison is above while 3 months year over year comparison is below.
Seventh Website with Lexical Relations and Semantic Relevance and Similarity for SEO
K9Web is another affiliate website that leveraged lexical relation, semantic relevance, and similarity. The project has been managed from June 2021 to January 2022 actively. After May 2022, the created content briefs, topical map, and semantic content network design are used. In the K9Web.com project, besides a topical map, and semantic content network design with content briefs, there is one more extra thing that is done which is an “article template”. To optimize the semantic relevance, and similarity with lexical relations, an article template is created for every different conceptual group or entity type. And, authors implemented the same connections, or the textually structured data graph for other main entities, and contexts.
K9Web.com continued to implement the semantic content network structure that is created for the project.
The K9Web organic search performance graphic from the Google Search Console for the worked timeline is below.
The 3 months organic search performance comparison for the actively worked timeline is below.
The 6 months comparison for the actively worked timeline is below.
The over 96% organic lick, and 85% organic impression increase for the 3 months comparison Y-O-Y is seen below.
The K9Web.com topical map focused on dog breeds, dog anatomy, dog fur, and dog foods. K9web is an affiliate for dog food brands. Thus, focusing on the dog food brands, dog foods, dog breeds and their attributes such as barking, energy level, or characteristics is helpful to increase lexical relations granularity along with semantic relevance and similarity.
In the coming Semantic SEO Course, the K9Web.com and VSSMonitoring.com topical maps and semantic content networks will be shared for teaching purposes.
Eighth Website with Lexical Relations and Semantic Relevance and Similarity for SEO
The eighth Website is Snuffstore.de. Snuffstore.de focuses on e-cigarettes and vaporizers for e-commerce purposes. The main language of the website is German, thus implementing the semantics is a little harder in terms of auditing the articles. But, thanks to the usage of certain authorship rules and algorithmic rules, quality audits have been performed successfully.
Organic search performance growth graphic of Snuffstore.de in 5 months.
The Snuffstore.de is a good example case study for extending an existing topical map, thus it will be processed further in the coming SEO Case Studies. To improve and dense the contextual relevance and semantic similarity of the content network, the specific types of e-cigarette and nicotine types of entities, and their association directions are used in the topical map. The e-commerce and conversion rate optimization-focused optimizations are performed in the second phase.
The 6 months organic search performance comparison of Snuffstore.de is visible above. The impression increase demonstrates the growth for new ranked queries, and not losing the average position makes the growth more successful.
“Running businesses in several different verticals online and across borders, we have become dependent on working with top-skilled professionals to get the results and placements we need for our web-based entities. Mr. Koray Tuğberk GÜBÜR is one of the most knowledgeable people we have come across in the SEO space. Working with him, we have been able to improve existing assets as well as launch new campaigns to drive growth.”
Teemu Salminen – Snuffstore Partner
Ninth Website with Lexical Relations and Semantic Relevance and Similarity for SEO
The ninth website that leveraged the lexical relations with a new topical map creation over an existing content network is Roxiecosmetics.co.uk. Due to the recession in the UK, the project has been managed actively for 6 months, and after the 6 months, the technical guides, content briefs, and a semantic content network design are taught and left to the client project. Roxiecosmetics.co.uk has a kind and noble leadership for digital marketing, and the brand improves its overall organic ranking performance. The Roxiecosmetics.co.uk is a helpful case study to understand the concept of Ranking States along with lexical relations. Because, the brand continued to lose traffic until a broad core algorithm update that can re-evaluate the published new content, and change existing content. In other words, certain types of topical authority and relevance assessments are not performed until a certain re-ranking trigger.
From negative to positive ranking state is visible below.
The Roxiecosmetics.co.uk will be processed in terms of expanding a topical map case study in the future. Below, the section that is created from the 0, only for improving the topical relevance, authority via relevance, and quality attribution is given with its organic performance growth.
Most of the organic growth comes from the section that is created from 0 for topical authority based on semantic SEO principles.
The quality increase or quality thresholds are evaluated by the search engines with comparative ranking. The improved quality of a website section affects the next section’s quality in the context of re-ranking.
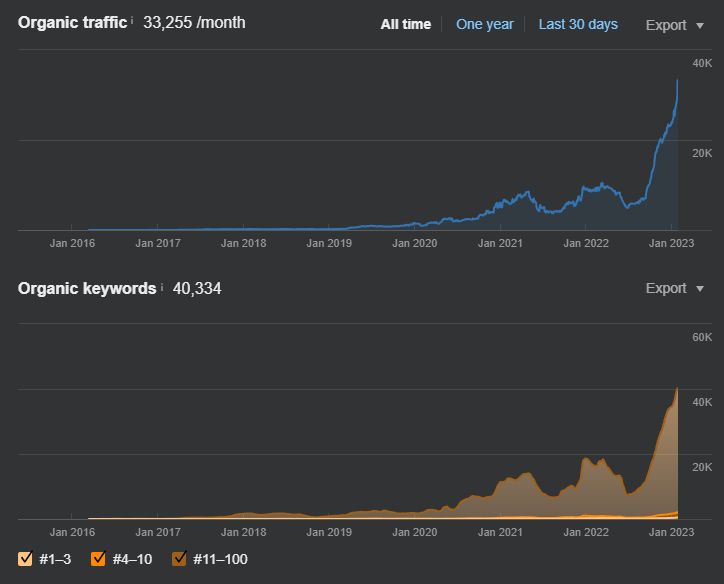
45 days later version of the traffic growth for Roxiecosmetics.co.uk is above.
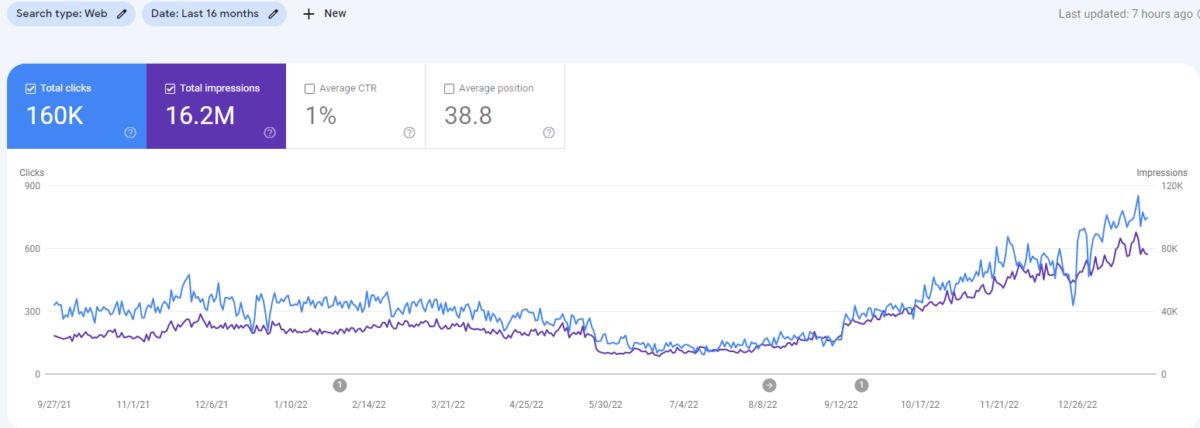
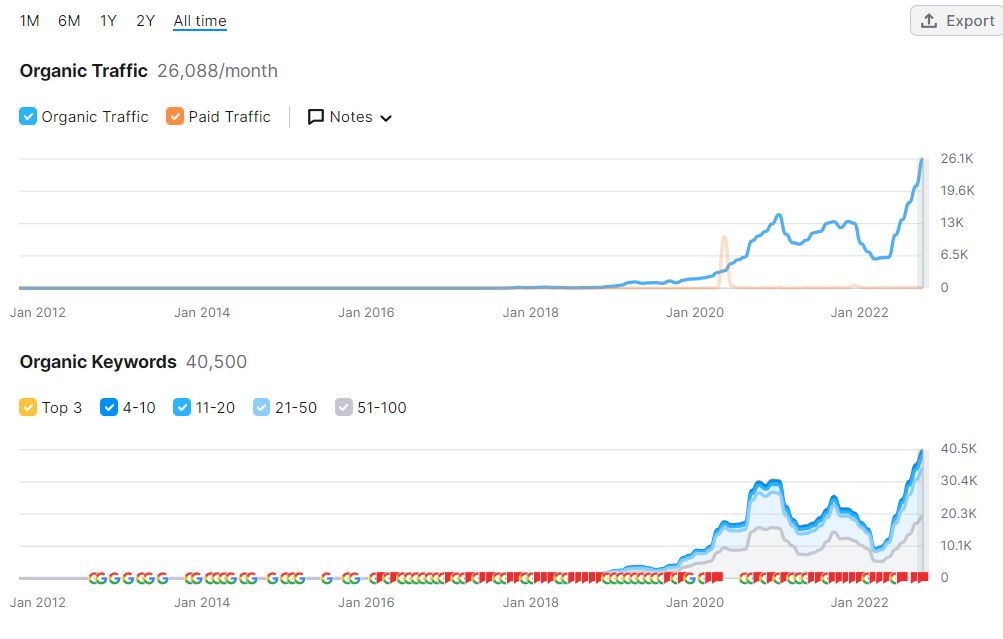
Tenth Website with Lexical Relations and Semantic Relevance and Similarity for SEO
ABCFinance.co.uk is a similar case study to Roxiecosmetics.co.uk. Thus, it will be processed together with the “extending a topical map” case study to explain the creation, and integration of topical maps to each other. Due to the online recession in the UK, the project is actively managed for 6 months. The ranking State concept for SEO is observed for ABCFinance.co.uk as well because until a broad core algorithm update that reverses the website’s identity, and authority from the negative ranking state that comes from previous BCAUs, the organic growth is delayed. After the latest spam, product review, helpful content, and in September Broad Core Algorithm Updates, the website started to improve its organic performance as before.
The lexical relations for ABCFinance.co.uk is used between “loan”, “credit”, and “mortgage” concepts. The UX, Layout, internal linking, and local SEO-related improvements for brand identity are performed too.
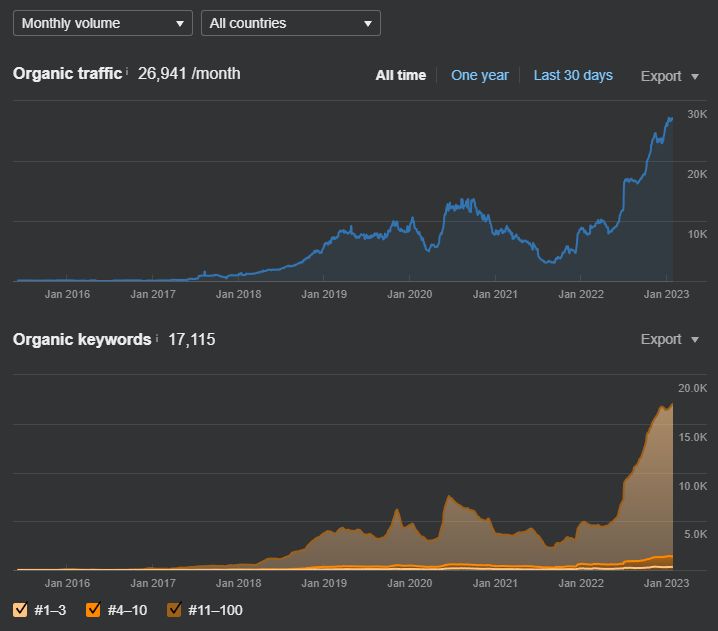
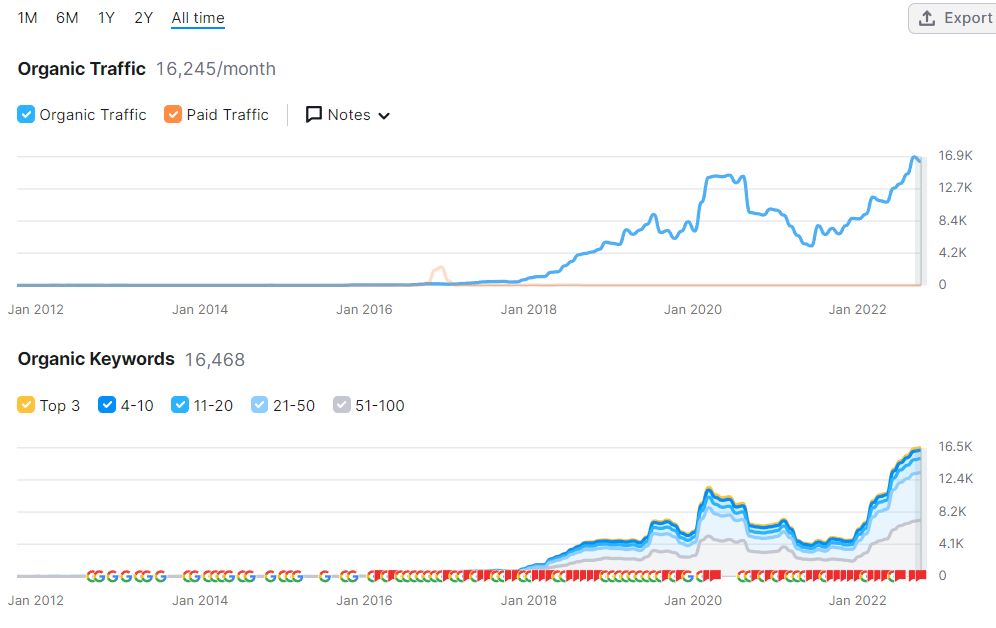
Eleventh Website with Lexical Relations and Semantic Relevance and Similarity for SEO
The eleventh website is a case study that I have published before, but it is highly relevant for the lexical relations and expanding a topical map, since the website existed before. The BKMKitap.com is a case study for SEO that shows the prominence of Broad Core Algorithm Updates because every BCAU affects the semantic closeness, relevance, and similarity of concepts. When a source improves the lexical relations for contextual improvements, it shows that the website has a higher chance of being ranked.
The BKMKitap.com is processed by extending a topical map case study together with some other sources to help SEOs to understand how to create a semantic SEO strategy to exceed the quality thresholds while shadowing the relevance and responsiveness of the competitors.
“By implementing an entity-focused SEO perspective with innovation, and following the new SEO Concepts, we were able to regain the lost organic traffic due to the latest Google Broad Core Algorithm Updates. By focusing on Core Algorithm Updates to improve the authority of BKMKitap.com with Koray’s guidance, we have created more granular and detailed content for e-commerce and informative web pages.”
Haluk Bolaban – BKMKitap.com Marketing Director
Twelfth Website with Lexical Relations and Semantic Relevance and Similarity for SEO
The twelfth website for the lexical relations SEO Case Study is similar to BKMKitap.com because it explains expanding a topical map to increase the topical relevance, and authority while expanding the industrial sectors of the brand. Thus, Encazip.com is processed also for brand identity expansion, which is closely related to the topical map expansion.
The Encazip.com SEO Case Study was published before, but updated 4 times after every over 100% organic search performance growth. The overall performance growth of the website is visible below.
The improvements for Encazip.com thanks to semantic SEO principles and holistic SEO techniques are below.
In the context of topical map and brand identity expansion, the section below is only for the “credit” which is a new industry for the brand, and its topical map is similar to ABCFinance.co.uk’s.
The section below is only for the Insurance industry, and it is similar to Sigortam.net in which I have even written the top-ranking content’s article templates with my own hands. It is important because still it ranks the first rank for the query “insurance” in Turkey.
Encazip.com is a multi-layered complex SEO Case Study, thus it should be watched and read from its own article and video, but lexical relations are used for its all layers.
We started working with Koray as he perfectly fits our needs. His technical and strategic SEO knowledge is unparalleled but more than that he has helped us by ensure SEO is understood by everyone in the business, and embedded into our culture, rather than just being a short term project. He has built a huge amount of credibility and trust both within the team, and our external shareholders, and I wouldn’t hesitate to recommend him.
Çağada Kırım – Encazip.com Founder
Thirteenth Website with Lexical Relations and Semantic Relevance and Similarity for SEO
The thirteenth website is TheCoolist.com. The website focuses on the “ranked entity lists” based on different contexts. Since it has “numeric values inside titles”, it is easier for search engines to get the purpose of the content, and the “comparative, superlative” context. The same approach is used for the new content briefs and topical maps that are created. Thus, TheCoolist.com is processed for the topical map extension SEO Case Study as well.
The chosen improvement point for TheCoolist.com is the “personality types”, because of all the “ranked lists of entities”, they are connected to home decoration, lifestyle, personality differences, personal matters, and social skills. Thus, inside the topical map, a conceptual gap is closed by creating highly authoritative content networks.
You can see the overall performance increase of TheCoolist.com for the last 6 months. The authorship team and author leaders are trained based on the semantic SEO principles of Holistic SEO, and certain SOPs, and training lectures are shared. Technical SEO sprints are implemented to improve crawl efficiency and search engine communication. Thus, site-wide improvements and certain content updates, content production methodology changes, and created new sub-folders affected the site-wide rankability.
Below, the improvements for the newly created personality subsection are visible.
The SEMrush organic performance change graphic for TheCoolist.com for the last 6 months is below.
The personality-related subfolder organic search performance that focuses on closing the topical gap by leveraging lexical semantics and relations is below.
“TheCoolist started as a playground for all things fashion and cool. With 10+ years of experience running content businesses, I decided it was time to take the site to a new level. We did well for a couple of years but then found that our growth wasn’t truly sustainable. That’s when I started to read everything I could about SEO, learn how the game has changed, and figure out the important signals I was ignoring.
Fast-forward a few months ago, I end up watching a Matt Diggity interview with Koray, called “Topical Authority Changes the Game”. And boy was it a game changer. While Koray speaks quite fast on hard-to-understand topics regarding patents and next-gen SEO, I couldn’t stop listening. Although I had almost no understanding of the concepts Koray was using, the inner voice was telling me it was all correct. Like 1+1 = 2 in math, it felt like everything he was saying made sense, everything was connected to things Google has been asking from publishers.
When the TheCoolist and Holistic SEO partnership started, the learning curve was steep for the team. The very complex terms used in our weekly calls seemed like we’ll never get to fully understand the big picture. I was afraid our writers and editors will just come up one day and say “we’ve had enough, this is too hard”. But I was sure it was the right path, so I pushed everyone to listen to a podcast, read an article, or check a YouTube video, as many times as possible to make sure we got the concepts right. And it paid off. We’re now stronger than ever, TheCoolist is growing, and the partnership has turned into a beautiful friendship as well.
Thank you, Koray for teaching us what SEO actually is. It was hard, it was painful (sometimes still is) but I have confidence that it will pay off.”
Alex Ion – Founder of TheCoolist
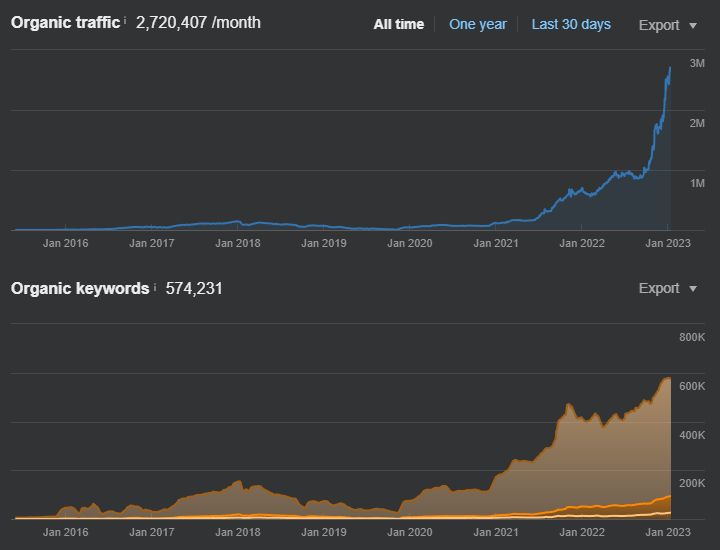
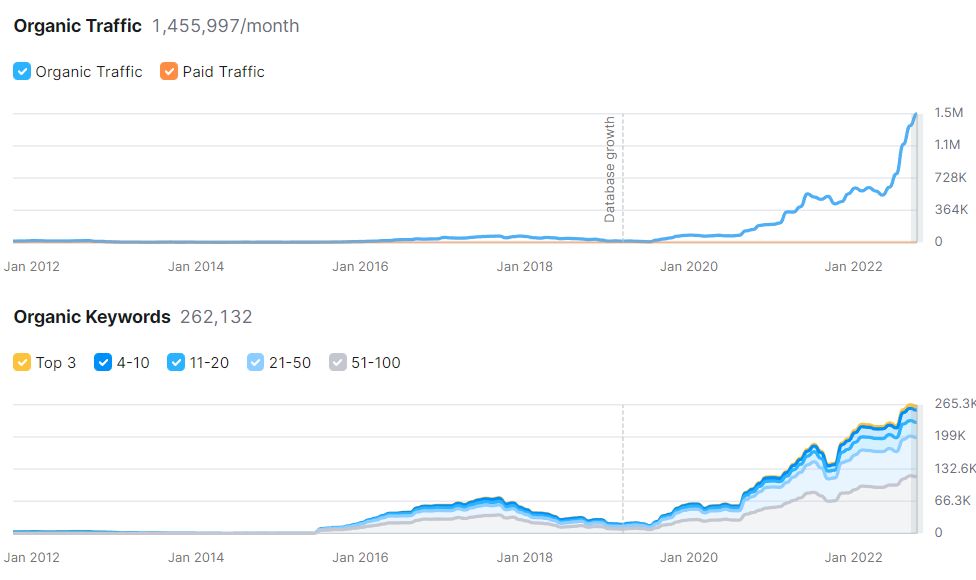
TheCoolist.com is featured during Google’s own Bard Announcement, and it directly demonstrates how our topical authority methodology and semantics are demonstrated in Google’s Artificial Generative and General Intelligence for search experience.
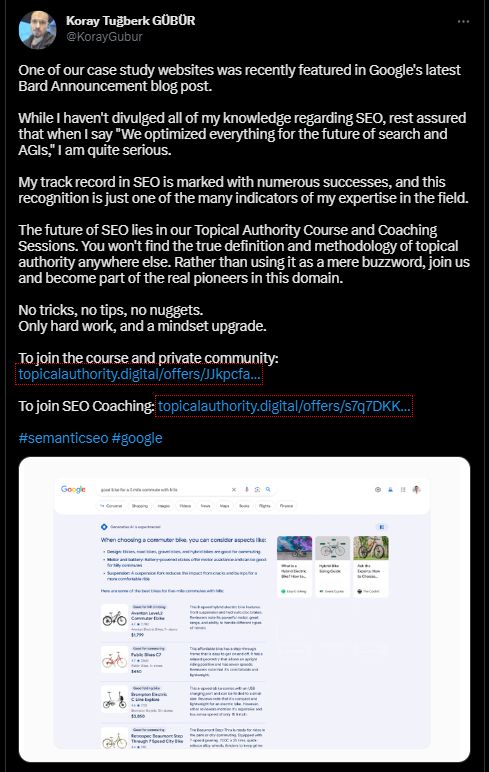
Fourteenth Website with Lexical Relations and Semantic Relevance and Similarity for SEO
The fourteenth website with lexical relations, semantic relevance, and similarity for SEO is Barschools.net. The overall website is supported with technical SEO, and article production, along with training for the company personnel for better content production.
The company continued to increase its overall organic performance thanks to strong brand authority, and relevance for a certain topic. The recipe sub-folder that was created for Barschools.net helped the brand to show its overall relevance for alcoholic drinks, not just for bartender courses. And, the lexical relations are used to bring the bartender occupation closer to the course, education, and cocktail preparations. The cocktail sections’ initial performance increase is below.
The same organic growth is visible from Ahrefs below.
The recipe section’s overall performance increase is visible below.
The used recipes for this section had structured data, crawlability, and image-related issues. But, recipe structured data, recipe instructions, recipe definition, ingredients, preparation needs, nutrients, and similar recipes are included to improve the lexical relevance between other recipes. The context vectors are created from 0 for every recipe and ingredient to improve the uniqueness and specificity of the context. To understand the specificity of the context, read the Contextual Search SEO Case Study. Lastly, Multilingual SEO is beneficial to understand the specific website further, in terms of asymmetric multilingual websites. Because cocktails are not popular in every country in the same way. Thus, translating every content to every language is not a proper SEO practice.
Fifteenth Website with Lexical Relations and Semantic Relevance and Similarity for SEO
The fifteenth website is Myros.com which is processed inside the Query Semantics SEO Case Study. Query Semantics is relevant to Lexical Semantics, but it is completely different from each other. Thus, Query Semantics focuses on the query term meaning, rather than the word meaning. Myros.com is a B2B Website that leverages B2C queries, thus query semantics are needed to be leveraged. To learn more about the case study, you can read the query semantics-related case study video, or you can read the article.
The entire e-commerce web pages including categories, products, main categories, and blog-related documents for the gift industry based on the local themes of Turkey are created with lexical relations mainly. The taxonomy and ontology of the semantics for the gift context and locale taxonomy are united. Myros.com leverages the local gift contexts, in other words, gift themes for every city in Turkey. Thus, gift attributes and locale attributes are united within a mixed taxonomy.
The overall organic search growth of Myros.com is below from SEMrush.
The Ahrefs graphic for Myros.com’s organic growth is below.
The overall traffic change of the website from GSC is below.
The Query Semantics SEO Case Study video is below.
Sixteenth Website with Lexical Relations and Semantic Relevance and Similarity for SEO
The sixteenth website with lexical relations and semantic relevance and similarity is Serapool.com. It is a pool construction company in the B2B industry. Since it is related to B2B SEO, the conversion funnels are complicated, and the conversion path is longer. Similar to Myros.com, query semantics and B2C query contexts are used to improve overall topical authority. The improved organic growth helped the client to demonstrate its expertise while helping the conversion rate and new client acquisition.
The lexical relations are used from pool construction to the pool materials, with different angles such as even cleaning materials. Because, according to the construction materials, cleaning materials change. With the help of those types of connections, the source exceeded the quality threshold.
Being present through digital channels is different from traditional methods for an industry that has a B2B business model. At this point, it is also important to consider how effective the owned media is among digital channels. We started working with Koray in this context since we needed to do things differently from ordinary SEO methods. The Semantic SEO strategies implemented by Koray are the most advanced globally. At the end of the process, we won a lot of visitors and conversions. Thanks to the semantic SEO concept and Koray Tuğberk!
The overall organic search performance growth is visible from Ahrefs. The overall organic search performance increase from SEMrush for Serapool is below.
The 6 months comparison from the GSC Naked Data is below for Serapool B2B SEO Project.
The Serapool is processed inside the B2B SEO Case Study as a separate subject website in the context of B2B Content Marketing, beyond semantics.
“Being present through digital channels is different from traditional methods for an industry that has a B2B business model. At this point, it is also important to consider how effective the owned media is among digital channels. We started working with Koray in this context since we needed to do things differently from ordinary SEO methods. The Semantic SEO strategies implemented by Koray are the most advanced globally. At the end of the process, we won a lot of visitors and conversions. Thanks to the semantic SEO concept and Koray Tuğberk!”
Hüseyin Bayram
Serapool Marketing Director
Seventeenth Website with Lexical Relations and Semantic Relevance and Similarity for SEO
The seventeenth website with lexical relations and semantics for SEO is Civar.com which is another search engine that focuses on local stores’ storage for helping consumers to find the same product in the closest store via a different Information Retrieval system. Civar.com is a search engine that takes its organic traffic, not from branding, or searches ecosystem, but directly from Google. Thus, the company needed a proper taxonomy, and ontology for the product graph, and a URL, website, and information tree were created for higher contextual relevance and consolidation.
Civar.com has a 0 Domain Rating (An Ahrefs Metric). Thus, it is processed inside the Information Responsiveness Book as a subject website.
The launch of the website is announced by me below. Thus, the project has been managed actively for the most important initial sections.
The updated version of the website’s organic search performance is below.
Not staying active, and not keeping the technical SEO improvements as they should be is one of the reasons for the search performance recession, but it can easily be connected to the SEO culture’s lack of the client as stated in the tweet.
Eighteenth Website with Lexical Relations and Semantic Relevance and Similarity for SEO
The eighteenth website with lexical relations and semantic relevance and similarity to SEO Case Study is Diamondrehabthailand.com. The active management of the project is handled at the beginning launch. The project’s topical map and semantic content network are created, designed, and after the initial launch, from the “Quality Threshold SEO Case Study”, some authorship SOPs are shared with the client so that some broken context signals from the articles can be saved.
The initial launch results from SEMrush are below.
The initial launch results from the Ahrefs are below.
The performance for organic search from Google Search Console for the actively managed time is below.
The topical map and the semantic content network of Diamond Rehab Thailand focus on the definitional concepts and methodological guides for luxury rehab. The addiction types, addiction reasons, symptoms, solutions, treatments, and approaches are used with lexical relations. For example, alcoholism addiction is in relation to drug addiction as holonymy and meronomy. Addiction is in relation to both hypernyms.
Nineteenth Website with Lexical Relations and Semantic Relevance and Similarity for SEO
The nineteenth website with lexical relations and semantic relevance, and similarity to the SEO case study, is Fit1001.com. Fit1001.com focuses on exercises, supplements, and diets. A healthy lifestyle and weight loss are central entities for the topical map. The initial launch of Fit1001.com is actively managed by me. The important part here is that Fit1001.com uses 10% of a foreign (English) topical map and semantic content network. In other words, it leveraged an existing design’s translation. This is another proof of the language-agnostic and meaning-based nature of semantic search engines.
The Ahrefs organic search performance for the subject website is below.
The organic search performance of Fit1001.com from the SEMrush is below.
The initial launch of Fit1001.com from the Google Search Console organic search performance is below.
Twentieth Website with Lexical Relations and Semantic Relevance and Similarity for SEO
Arabamkacpara.co is the twentieth website with lexical relations and semantic relevance and similarity SEO Case Study is an exact match domain. Thus, it will be handled in Exact Match Domain SEO Case Study at a different time. The Arabamkacpara.co initial launch is handled directly by me as a subproject of the Vava.cars. Vava.cars is a used car trader and portal for end users. Thus, “arabamkacpara” means “how much my car is”. It has a car evaluation engine to keep the website responsive and relevant to certain queries.
The section below shows the initial launch of Arabamkacpara.co from Ahrefs.
The organic traffic increase with lexical semantics for the Arabamkacpara.co is below.
Since, this is an exact match domain project, the distance between the “brand name” and the “query context” is important. To own a phrase as a brand, the website has to create brand value and overall topical relevance for the brand name terms. Thus, changing the meaning of the query, and making Google interpret the query as a brand name, is the purpose of an exact match domain. To improve the lexical relevance of the brand names to the query phrases, the SEO should focus on the abbreviations, or synonym phrases of the same query with alternate brand names.
Twenty-first Website with Lexical Relations and Semantic Relevance and Similarity for SEO
The twenty-first website of the lexical semantics and semantic similarity, relevance SEO Case Study is Complaintsboard.com. Complaintsboard.com is a competitor to Sitejabber, Pissedconsumer, and some other global review websites such as Trustpilot. The main advantage of the review websites is that they always have high PageRank, and since they are third-party review websites, Google ranks them for the brands’ names so that objective reviews can be visible to everyone. Another advantage of review websites is that the company is directly linked by the reviewed website’s homepage or about us page. But, Complaintsboard.com mainly focuses on the lexical semantics since it doesn’t have the proper brand power, or review widgets that the third-party websites use for promotion.
The initial results from the Ahrefs for Complaintsboard.com is above.
The initial results for Complaintsboard.com from SEMrush are above. The Complaintsboard.com is processed in the Cost-of-retrieval SEO Case Study to explain the technical SEO and semantic SEO intersections.
The latest Core Algorithm Update affected Complaintsboard.com negatively, but it was mainly a “source-type” switch on the SERP. Thus, some competitors were impacted harshly by it. For example, you can see Sitejabber’s organic search performance changes as below.
But, the same didn’t happen to Complaintsboard.com thanks to lexical semantics and cost-of-retrieval understanding and optimizing per web page.
“Working with Tugberk as a guru of Holistic SEO for 2 years changed my understanding of how search engines see websites. Slowly but steadily, we have improved the performance of the website. Content delivery to users became faster than ever before. Discovering new things brought new energy to our team at the time when ComplaintsBoard.com got hit by Google updates hard. I am confident that cooperation with Holistic SEO will help to fulfill the full potential of ComplaintsBoard.com and make the most valuable platform for resolving consumer complaints.”
Sergey Ku – Owner of Complaintsboard.com
Twenty-second Website with Lexical Relations and Semantic Relevance and Similarity for SEO
Vava.cars is another SEO Project that used lexical semantics since its early beginnings. But, the project used heavy “branding” and “digital marketing” rather than a full-scale holistic SEO mindset. There are various reasons for it, such as a lack of IT teams, or small content production possibilities. Thus, from B2B to B2C migration, the Vava.cars SEO Campaign found more room for organic search growth. The first thing that is done for the Vava.cars is to register it to Google’s own Knowledge Graph for further Expertise-Authoritavness and Trustworthiness signals. Because the brand is a subsidiary of Petrol Ofisi which is one of the most important companies in Turkey. To create a new reliable brand inside the B2B SEO Campaigns, I had to register the brand to Google’s Knowledge Graph so that a new brand can rank higher for the most competitive and conversion-focused queries. Since we didn’t have a proper content production possibility, I focused on mainly the direct conversion queries, until the B2C migration. During the B2C or Direct to Consumer migration from B2B Mindset, the car model, and the brand graph are created inside a proper taxonomy, and information tree to improve the semantic relevance between the web pages while creating a better contextual crawl path for the crawlers.
I created the Wikipedia page below for the company.
The company is registered to the Google Knowledge Graph by having an entity ID. The same entity registration is visible inside Google Trends.
To support the brand power, I registered the company to the different physical map locations inside Yandex, Microsoft Bing, Apple Maps, and Google Maps with the same name, address, and phone number types of consistent information.
Thanks to this, the company is able to show its type, and focus on the search engine for further relevance.
But, the lack of IT teams, or authors’ content production capacity, the project continued with branding. The lack of server-side rendering and client-side rendering-dependent technologies created big ranking problems until the brand has enough historical data for gaining the trust of the search engine. But, thanks to consistent brand search and conversions from certain queries helped the brand to appear in auto-suggestions and the related search terms.
“Araç değerleme” is the most important conversion keyword. And, the main purpose was to bring the brand closer to the specific query with historical data. The other big companies are already inside the auto-suggestions, such as “sahibinden”. The next step was to bring “vavacars” closer to the “sahibinden”.
In the related search terms, you see the “wiki” and sahibinden” together with the Vavacars. It shows that the brand is related to the biggest competitors, and it has a registration inside an industrial directory. There are more optimizations for especially the local SEO for reviews, images, main category choices, phone and message answers, bad review dealing, and registering the business places.
Some local SEO-related improvements are given below. The Google Maps Excel file template is used for adding and managing multiple businesses at the same time.
The Ahrefs organic search performance graphic below demonstrates the brand’s growth with SEO.
The same graphic is way much better inside SEMrush for Vava.cars. But, I must tell you that Ahrefs is 80% more accurate for this project.
The Vava.cars project started with Holistic SEO & Digital in 2020, and it still continues.
Twenty-third Website with Lexical Relations and Semantic Relevance and Similarity for SEO
The twenty-third Website with Lexical Relations and Semantic Relevance and Similarity for SEO is Diyetkolik.com. Diyetkolik and Fit1001 come from the same nature and the same client. Implementing the semantics for two different contexts. Diyetkolik focuses on dietitian services for healthy weight loss and well-being in a fit lifestyle. The calorie calculations and food recipes bring the main traffic to the website. Diyetkolik.com used semantic SEO and Technical SEO improvements since the beginning.
From web security improvements, to crawl efficiency optimization, progressive web applications, or semantic SEO practices such as a topical map, and semantic content network design for different contexts such as nutrients, minerals, amino acids, food types, recipes, and more. The organic growth for Diyetkolik.com is below. From the image above, you can see the speed and richness of the technical SEO sprint that is implemented on Diyetkolik.
The Diyetkolik Project started in October 2020, and for over two years it continues. Below, you can see the start date and change in the traffic for the later stages of SEMrush more clearly.
Twenty-fourth Website with Lexical Relations and Semantic Relevance and Similarity for SEO
The twenty-fourth website with Lexical Relations and Semantic Relevance and Similarity for SEO is JookSMS.com which is another small SaaS company that focuses on SMS Marketing. The JookSMS.com topical map and the semantic content network design focus on the SMS Marketing concepts, guides, strategies, and methodologies analysis for the conversions, purposes, and different types of SMS styles for conversions. The technical SEO implementations and sprints are followed especially for uniting the unnecessary web pages or decreasing the amount of the total web page count to increase the PageRank per web page.
The initial launch and ranking changes for JookSMS.com from SEMrush are below.
The initial launch and ranking changes for JookSMS.com from Ahrefs are below.
The Google Search Console data for the organic search performance of JookSMS.com is below.
The concept of source shadowing is explained in the Cost-of-retrieval SEO Case Study and the book. JookSMS.com is processed further inside the SaaS SEO Case Study.
Twenty-fifth Website with Lexical Relations and Semantic Relevance and Similarity for SEO
The twenty-fifth and the last website in the SEO Case Study is from 3D Printing and CNC Machining industry as a B2B and publicly traded company in New York. Thus, its name is classified, but the NDA Contract is shared to demonstrate the reason for hiding the company name. The twenty-fifth Website with Lexical relations and semantic similarity, relevance SEO Case Study focuses on 3D Printing Types, Materials, Laser Cutting and Cutters, CNC Machining, methods, techniques, historical improvements, services, and prices. The 3D Printing and Plastic Injection Molding are put into the center of the topical map with relative importance signals, and upper organizations’ authority is used for higher search authority.
The results for the twenty-fifth SEO project in Lexical Semantics SEO Case Study are below from Ahrefs.
The results for the twenty-fifth SEO project in Lexical Semantics SEO Case Study are below from SEMrush.
The results for the twenty-fifth SEO project in Lexical Semantics SEO Case Study are below from Google Search Console for the last 16 months.
The quick organic search performance growth from Google Search Console 6 months comparison is visible below.
The 3 months comparison from Google Search Console is below.
The year-over-year 3 months comparison for Google Search Console is below.
The NDA Agreement with the client is below.
The specific SEO Case Study is processed further inside the B2B SEO Case Study with other projects.
Twenty-sixth Website with Lexical Relations and Semantic Relevance and Similarity for SEO
The twenty-sixth website with lexical semantics (word semantics), relations, and semantic relevance with similarity is from the “EB 5 Visa“and “foreign investor” industries. The EB5 visa and investment consultancy company name is Eb5brics.com. EB5Brics.com is a project that I personally care about due to the high friendship, and noble spirit of the owner of the company. EB5brics.com is an authoritative visa consultancy company from the USA that targets people who try to have citizenship or residency in the United States with different investment strategies. The project has leveraged different types of Technical SEO and Semantic SEO improvements along with internal link optimization methodologies. The twenty-sixth project of the Lexical Relations and Semantics SEO Case Study focuses on “Visa”, “Visa Types”, “Investment Strategies”, “Investment as a Foreigner”, “Investment for Citizenship”, “EB5 Visa”, “GreenCard” and “From X Country to Y Country Visa Strategies”. To have better lexical relations, specific content briefs, and semantic content networks are designed with a topical map.
My journey with the owner of the EB5Brics started around March 8, and it was an immediate contact. During the project, he worked proactively for consolidating the information from me and implementing it on the website. The proactive communication might be seen below, the same day in March, after the first email, the project started.

I am giving these types of details because I will remember these days after my consultation years, sweetly. Ahrefs organic search performance graphic for EB5brics.com is below.
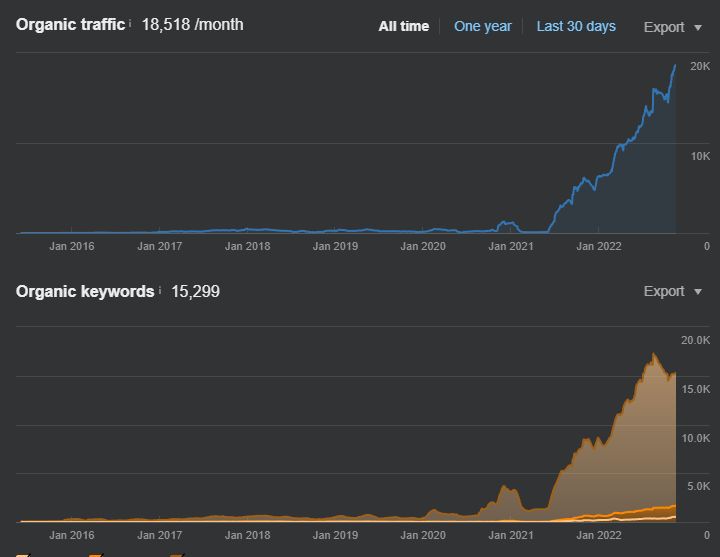
The SEMrush Organic Search Performance for EB5brics.com is below.
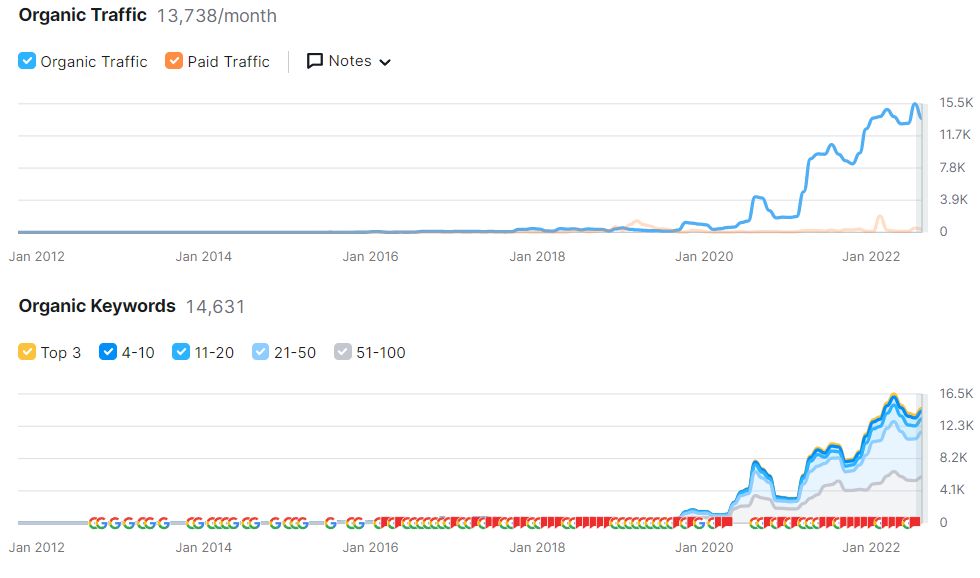
The last 6 months of comparison data from organic search performance from Google Search Console is below.
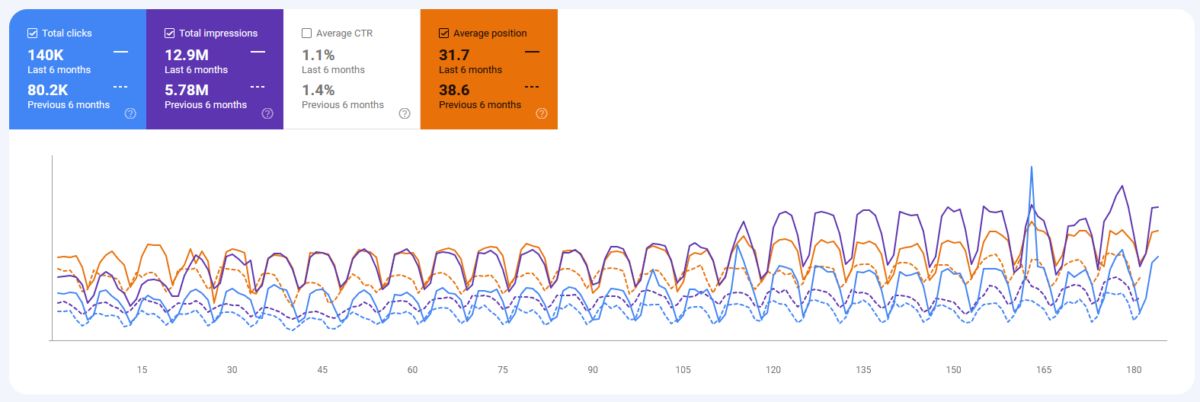
The year-over-year 3 months comparison data is below.
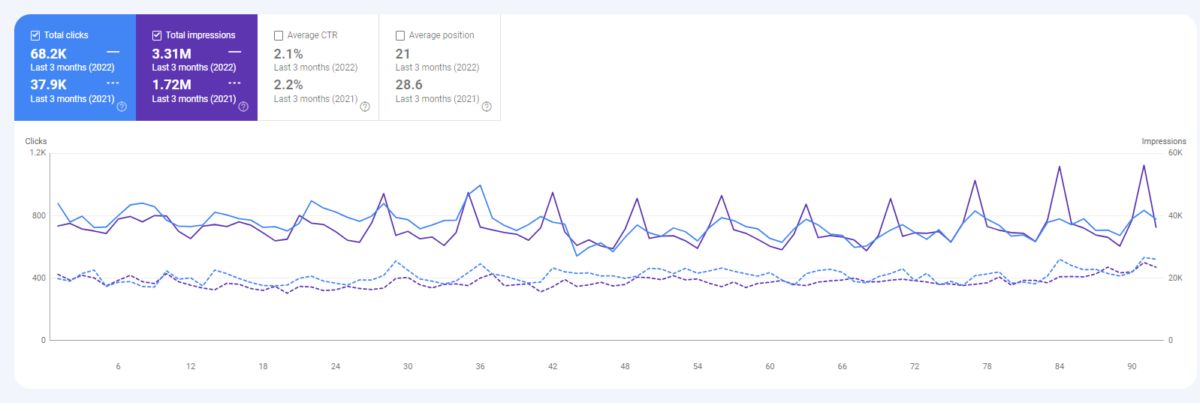
I am always happy to know that there are good people that reach out and stay patient despite my migraine attacks.
Koray Tuğberk GÜBÜR – Holistic SEO & Digital Founder
Twenty-seventh Website with Lexical Relations and Semantic Relevance and Similarity for SEO
The twenty-seventh website with Lexical Relations and Semantics has four different languages, Russian, Persian, Turkish, and Arabic. The twenty-seventh website with lexical semantics is Realtyproperty.group which focuses on citizenship via real estate investment. The project is processed further inside the Cost-of-retrieval and Information Responsiveness Books and Case Studies because it continued to rank with duplicate content and inferred redirections from another original source due to various reasons. It is done to see whether the problem was content or a heavy, bloated website. Thus, a brand-new domain is opened without links, and it is used as below.
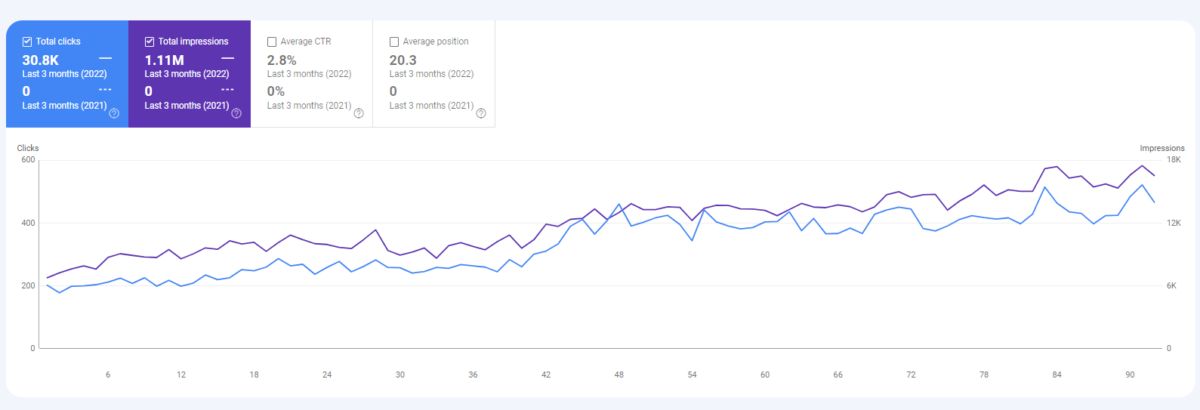
Since the website is new, the last 3 months of comparison from GSC, as year-over-year shows from 0 to 30,000 organic clicks, and from 0 to 1,110,000 impression increase.
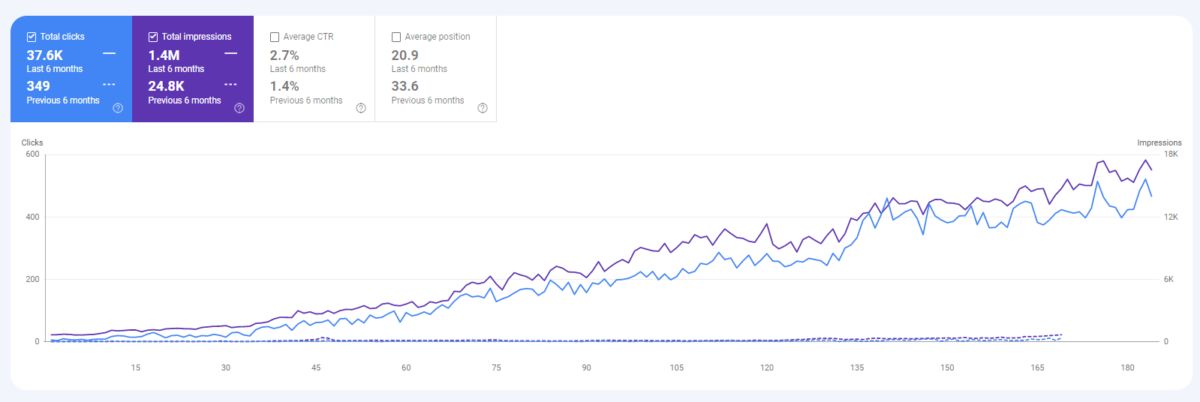
The six months of organic search performance comparison demonstrates how new the website is ranking better than the 10 years of old company website without any support, and a simple WordPress setup thanks to lexical semantics for semantic search engines.
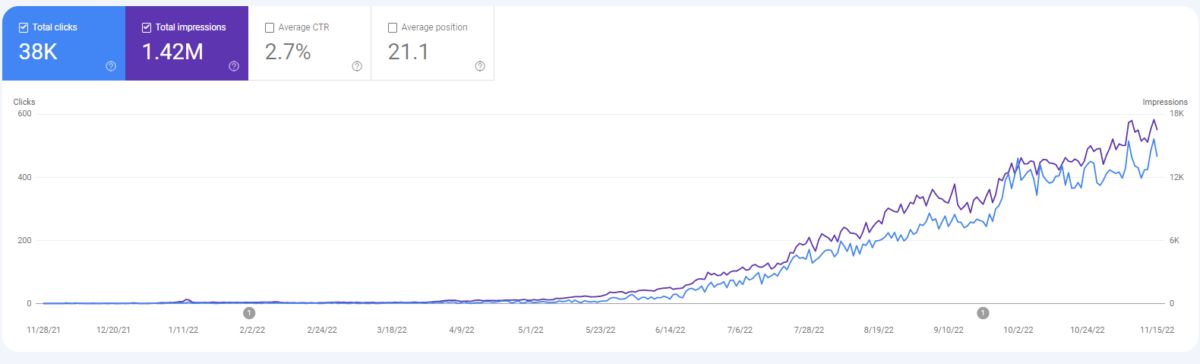
The Ahrefs organic search performance change for the brand-new domain without links and with duplicate content is below.

The SEMrush organic search performance graphic is similar to the previous one (Ahrefs organic search performance for 27th lexical relations website), as below.
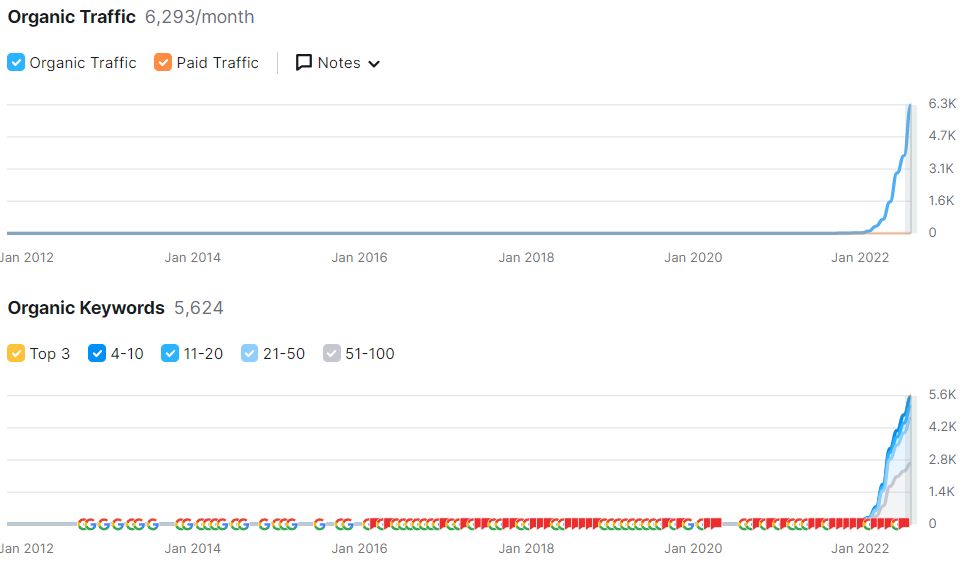
The overall traffic increase and the initial ranking cycle of the website are below.
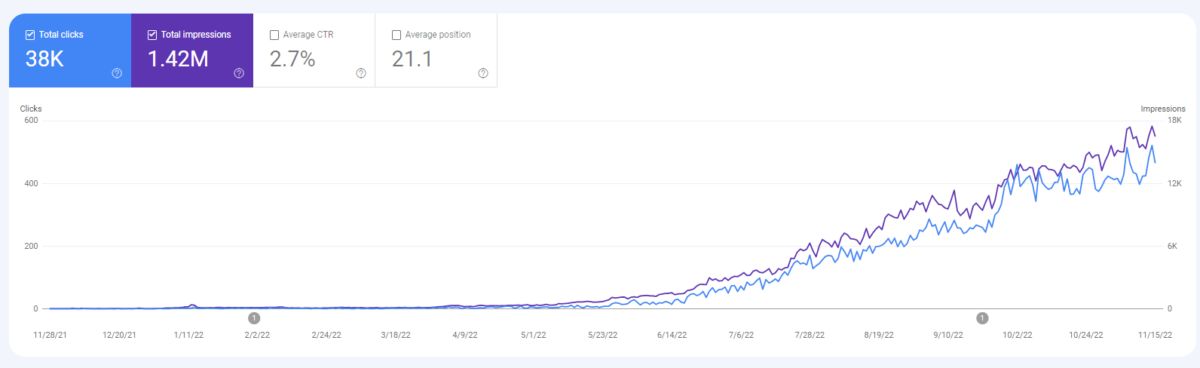
To use the lexical semantics and relations properly, the local taxonomy and proximity along with real estate investment, and travel guides for different destinations and actions are used. The connections between countries, cities, and activities along with lifestyle and real-estate costs or citizenship in the country are explained. The company has an active YouTube Channel too, which is helpful for further topical relevance and E-A-T Signals.
Twenty-eight Websites with Lexical Relations and Semantic Relevance and Similarity for SEO
The twenty-eight website with lexical relations and semantics is from the Solar Panels industry, it focuses on solar energy and solar panel production with installations in Australia. This project started 65 days ago, and it had problems with the creation of an author team. During this time, to let the designed semantic content network and topical map perform better for the initial rankings, we focused on technical SEO improvements and implementations. The traffic increased by over 100% in 65 days.
The lexical semantics are not used for the project yet, actively. But in the future, the project will be continued to add here with updated results with the open information and data. It is put here to show that this is an always-on SEO case study with semantics and lexicosemantics, and how a topical map can perform better if we focus on technical SEO before putting the first content. If you do not have authors, you can use other SEO verticals for a holistic SEO approach.
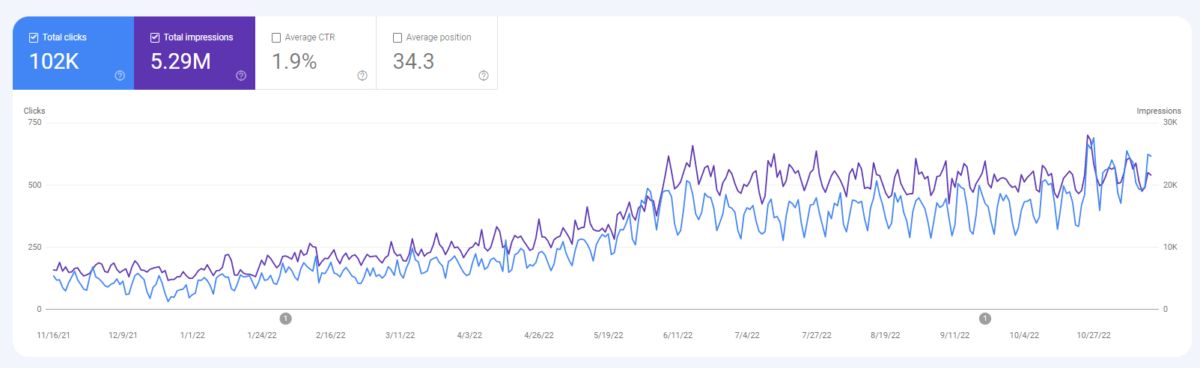
The performance change from Ahrefs graph for the same website is below.
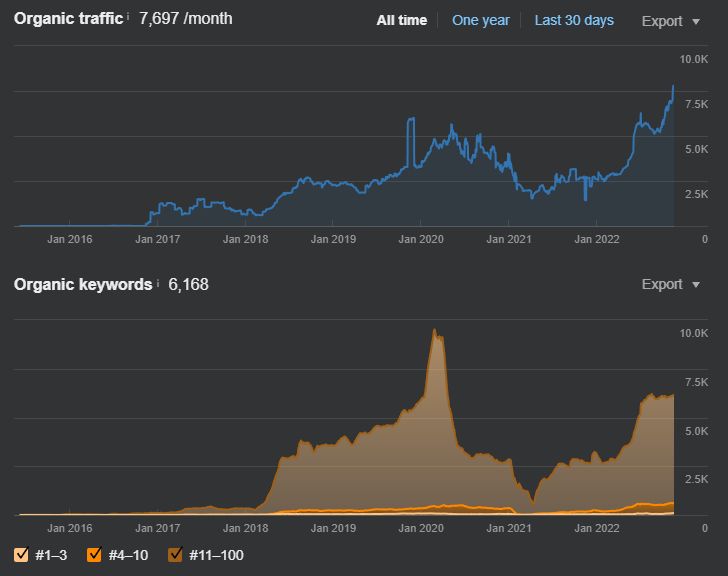
After the launch of the first semantic content network, the project will be opened to be public.
Twenty-ninth Websites with Lexical Relations and Semantic Relevance and Similarity for SEO
The details will be written later.
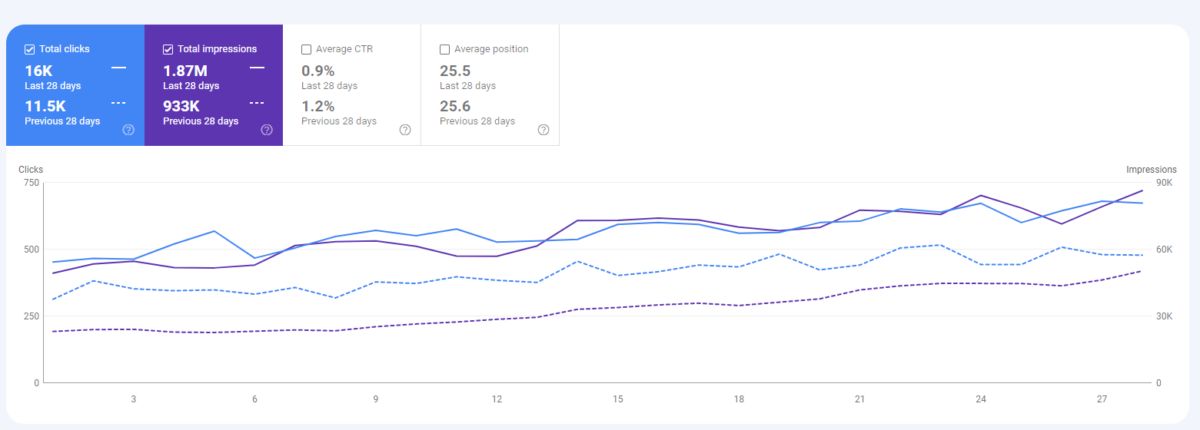
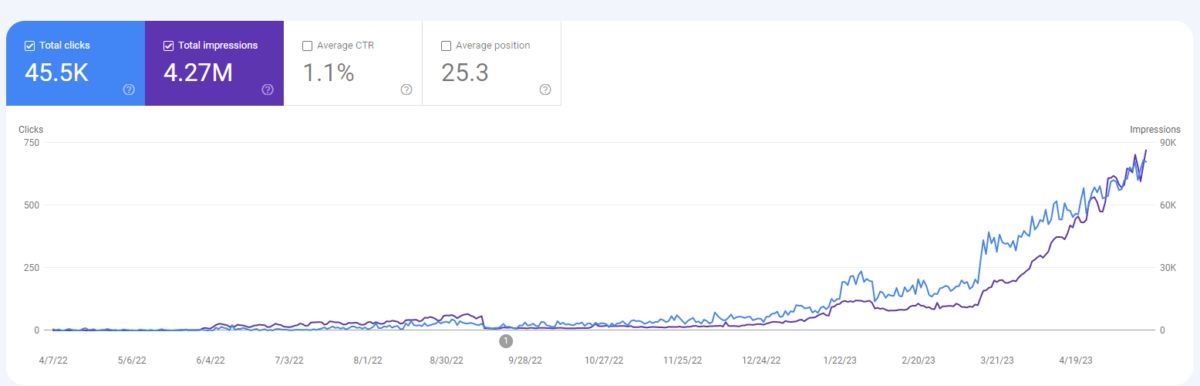
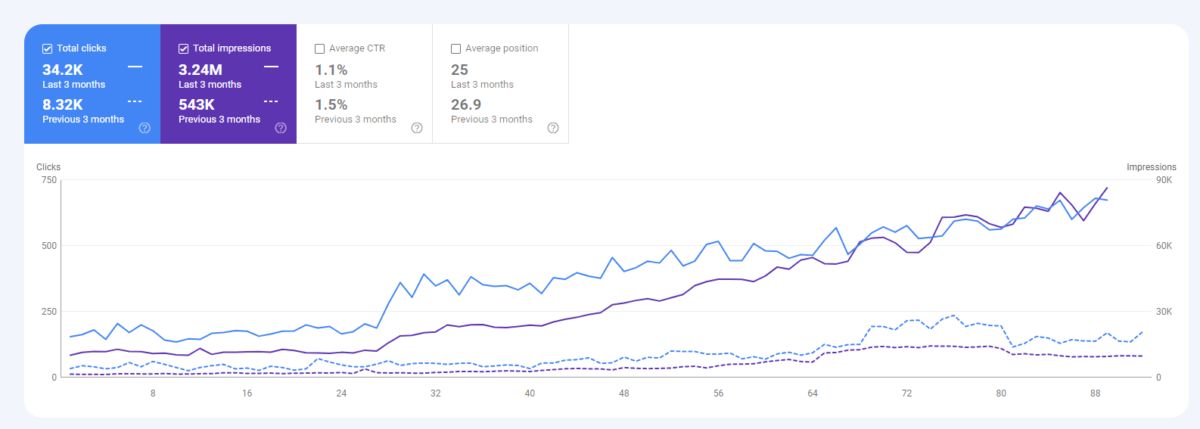
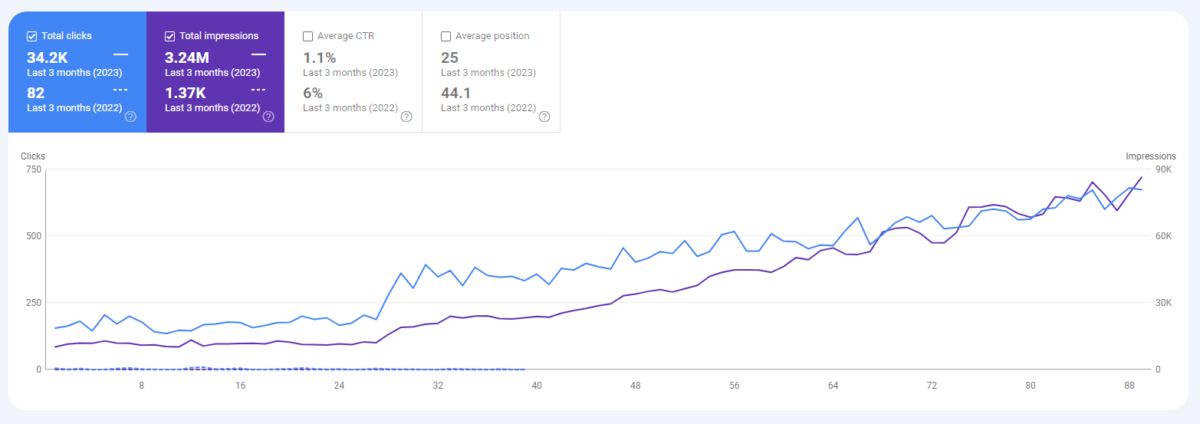
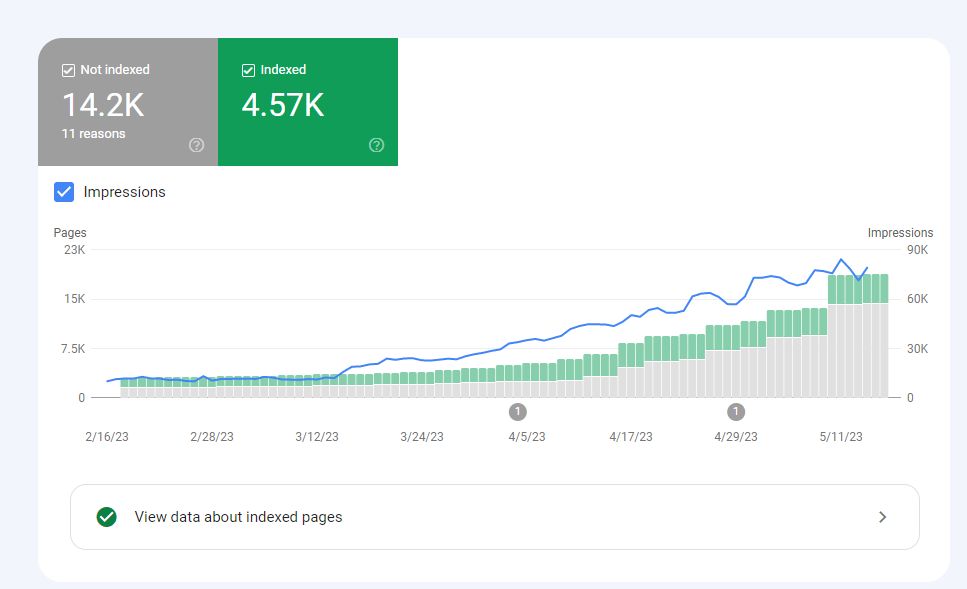
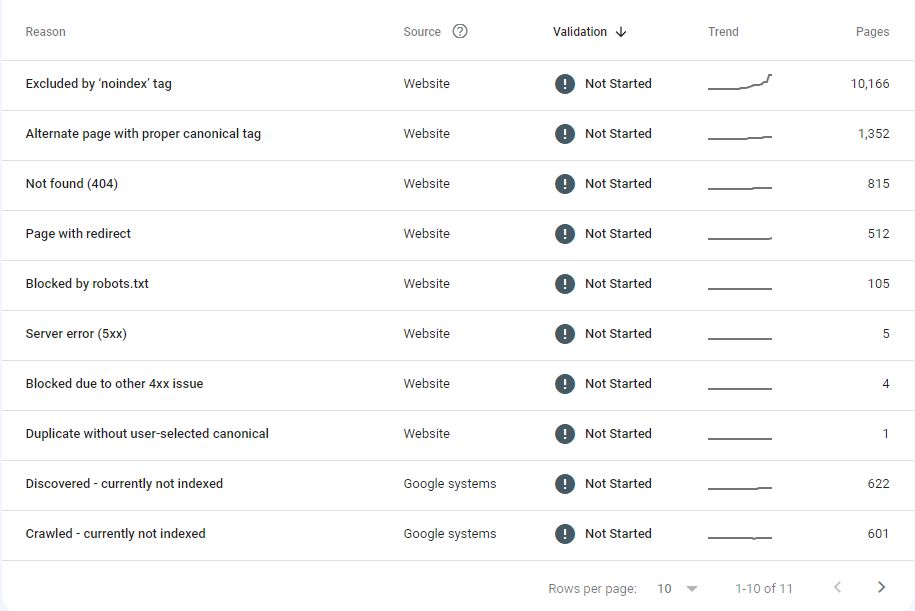
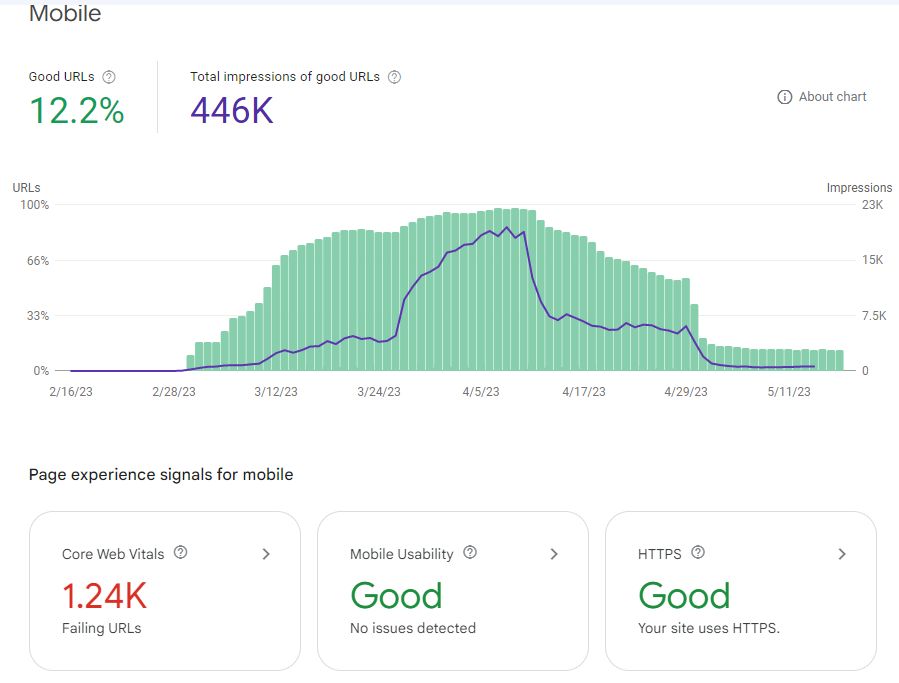

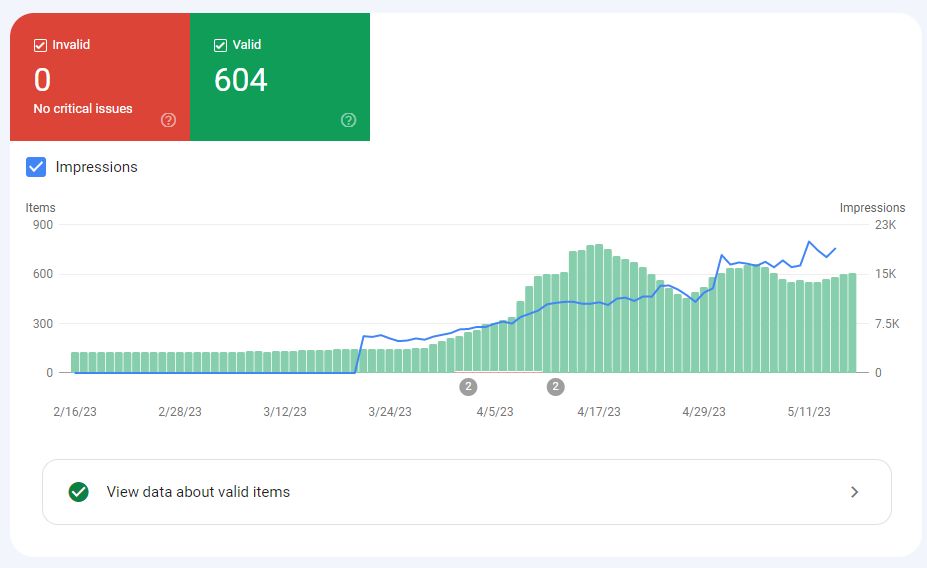
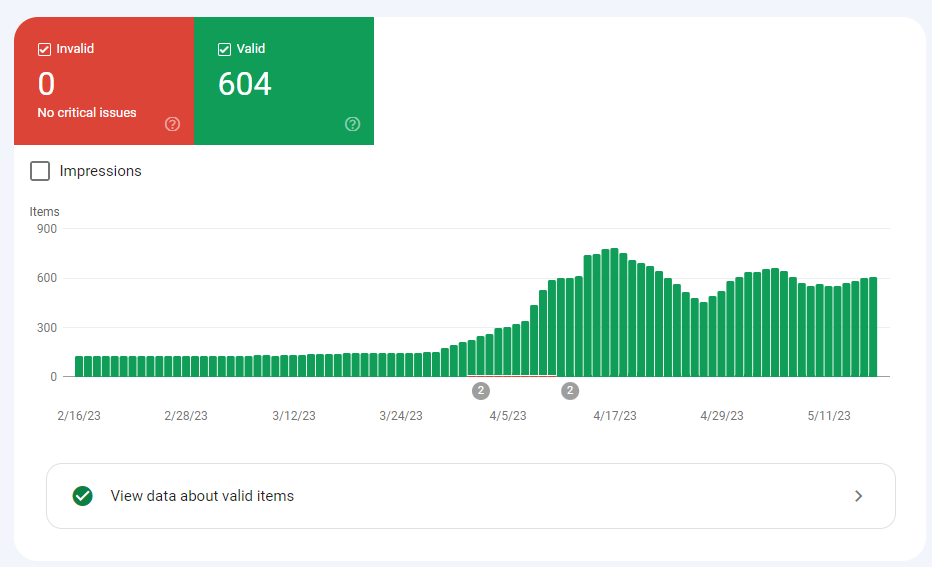
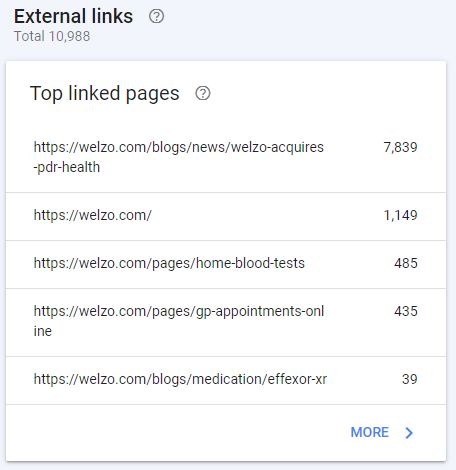
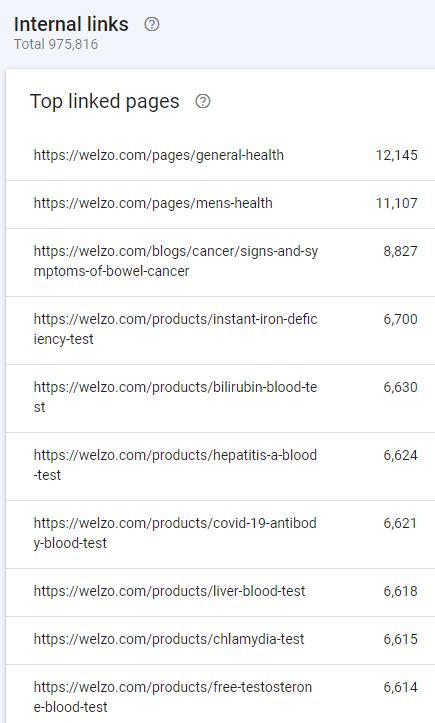
Thirteenth Website with Lexical Relations and Semantic Relevance and Similarity for SEO
The details will be written later.
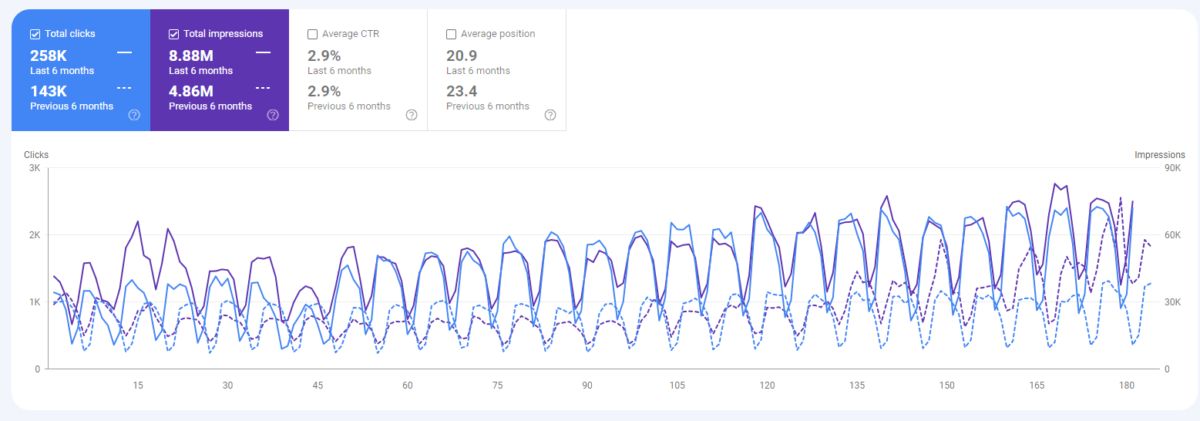
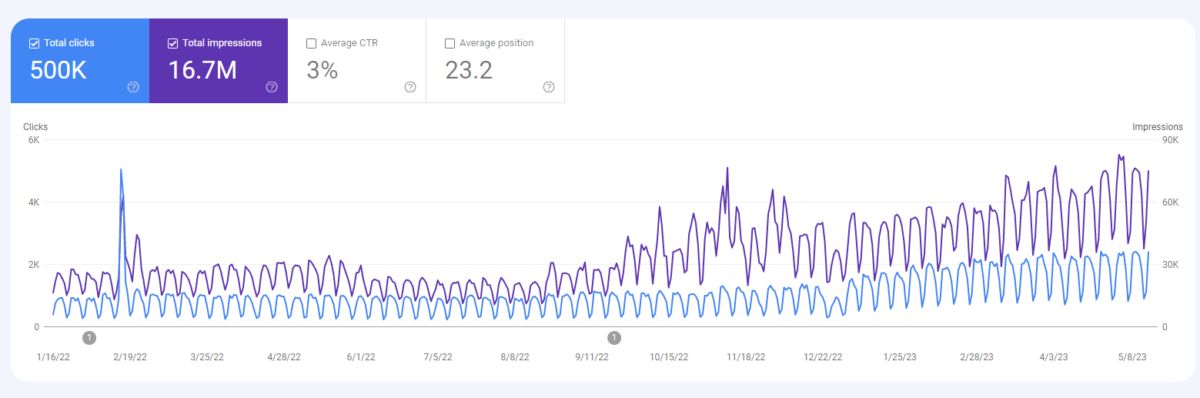

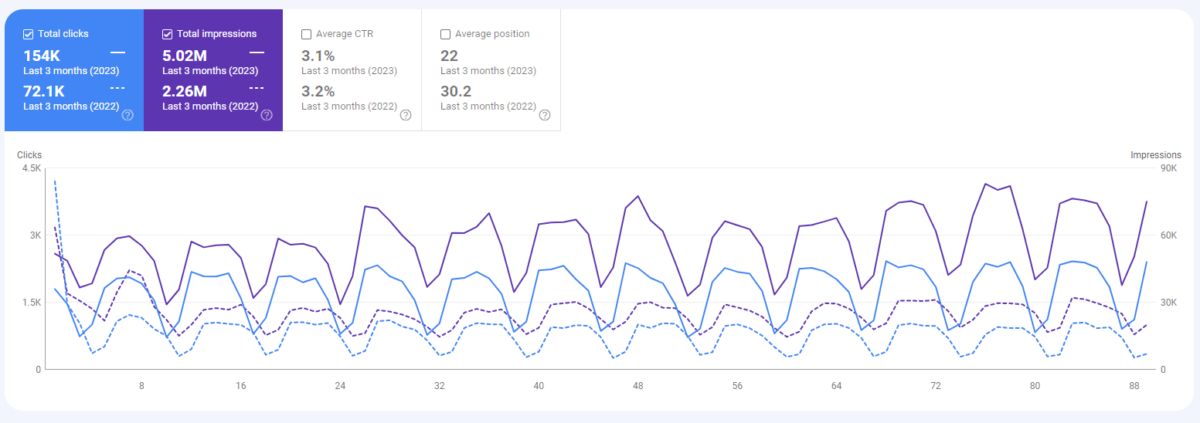
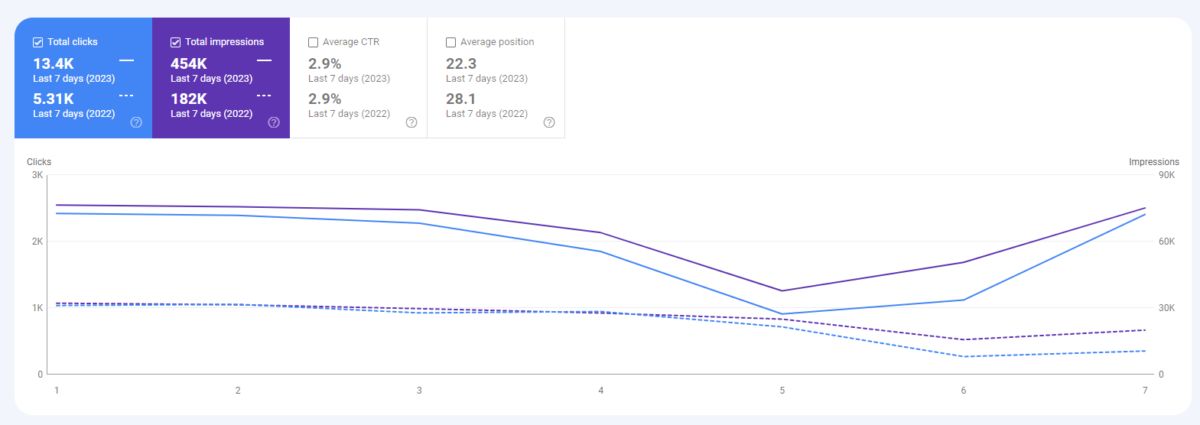
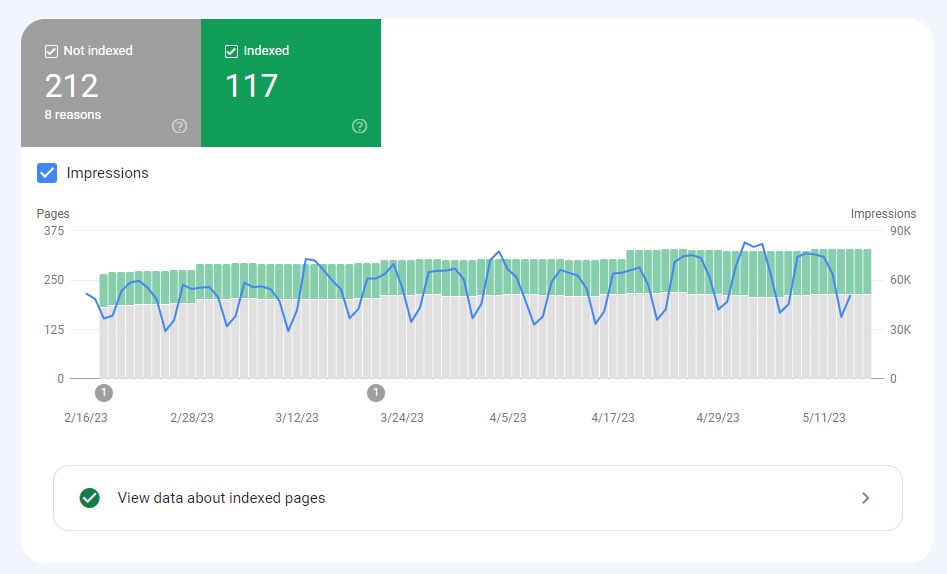
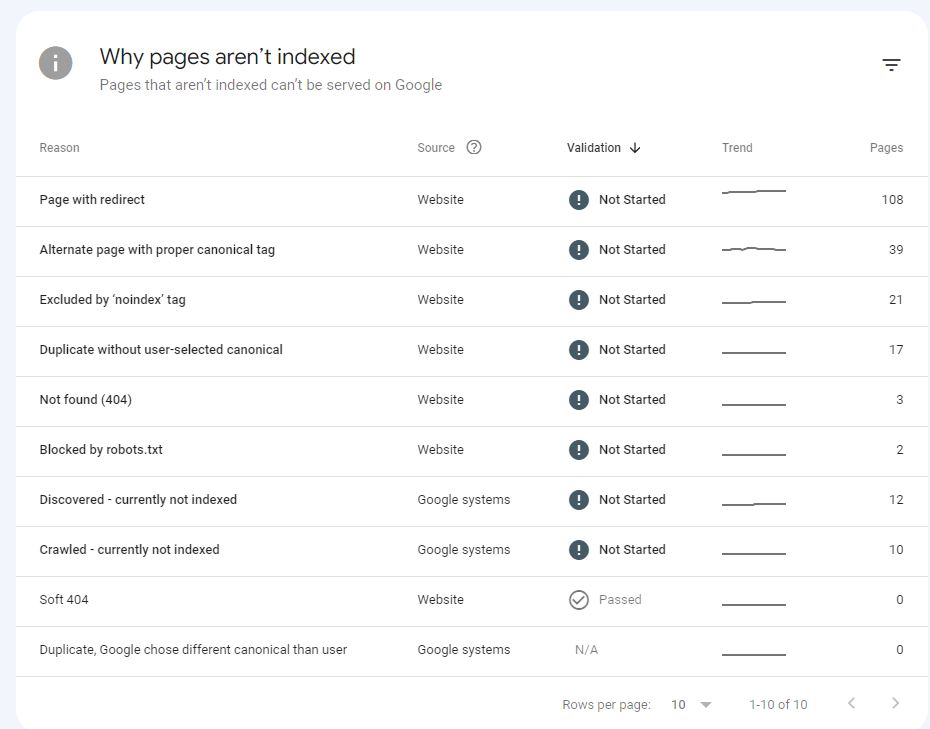
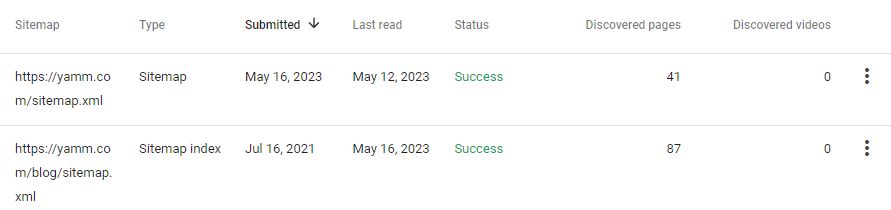
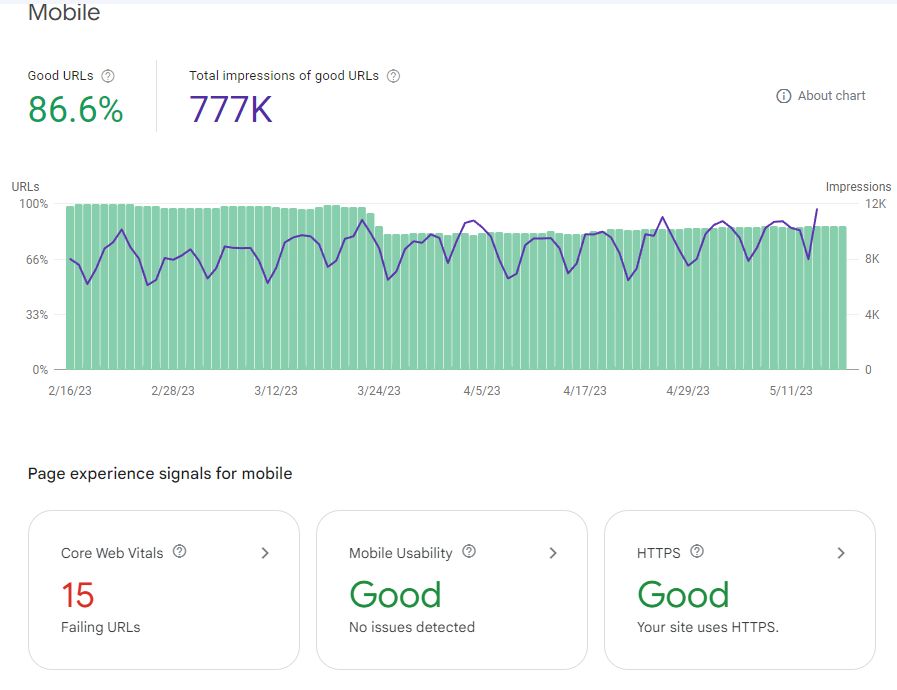
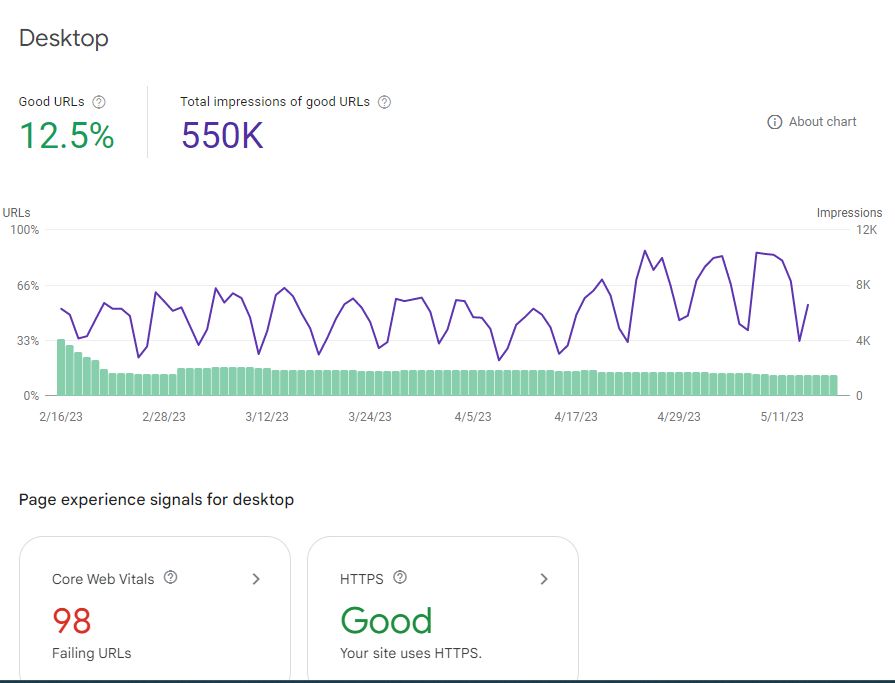
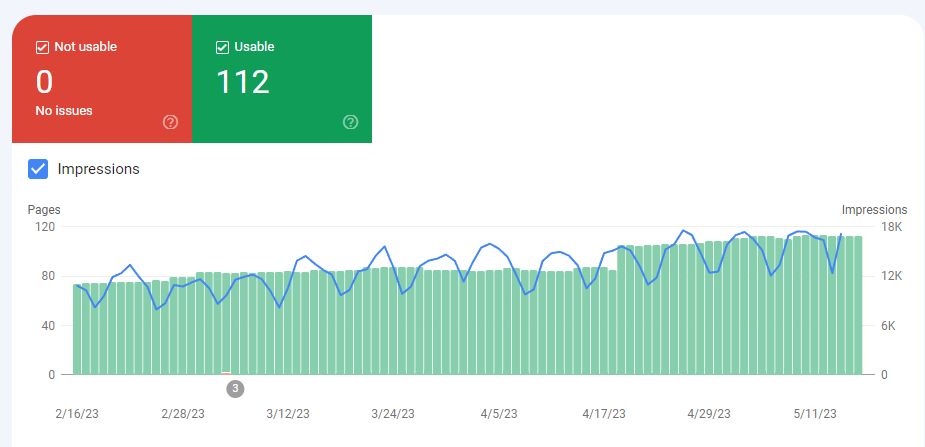
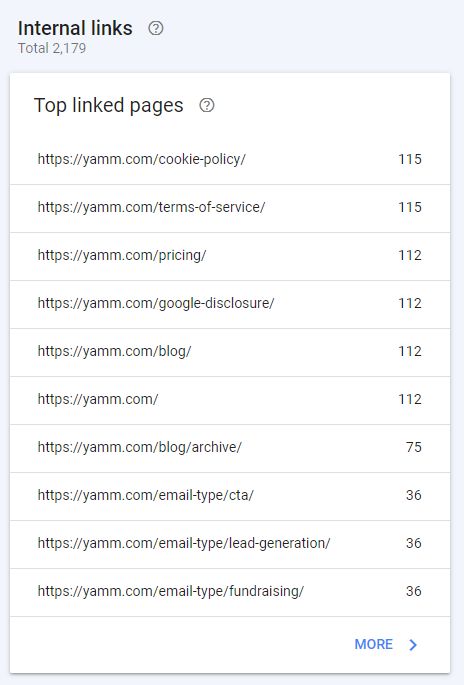
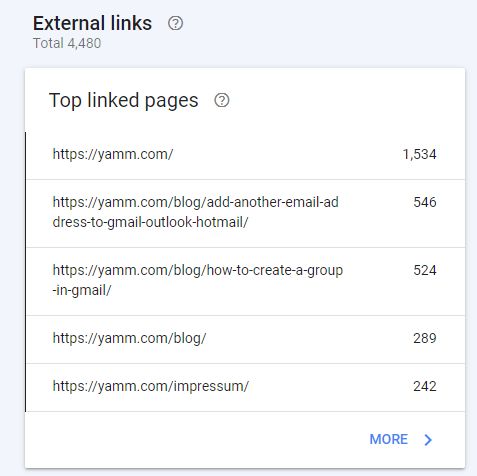
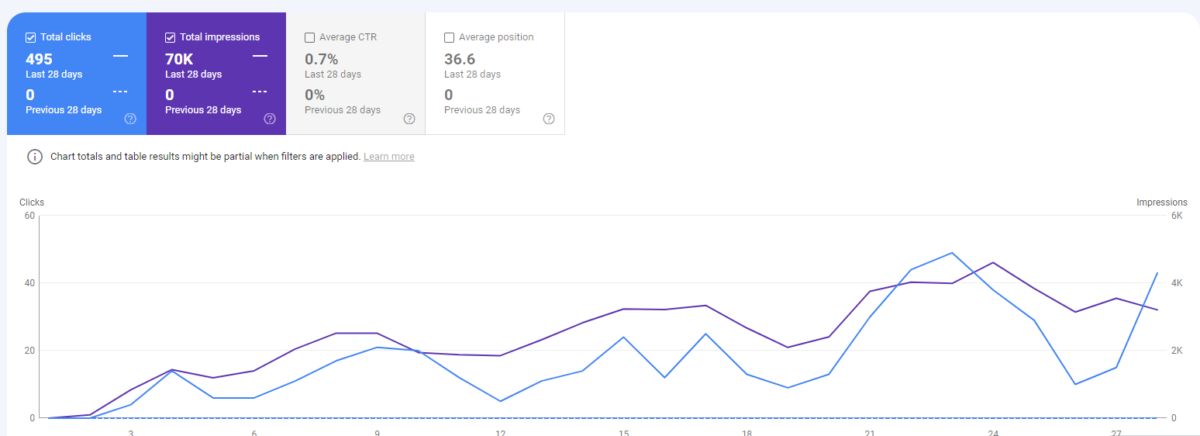
The Key Points of Semantic Similarity for SEO are given below.
- Semantic Similarity helps for improving the context by sharpening, specifying, and deepening to improve overall relevance.
- Semantic Similarity is connected to Lexical Semantics because similar existing things are closely related within hypernym, hyponym, or meronymy types of relations.
- Semantic Similarity is different from relevance. But, semantically relevant things are usually too similar, or exact opposites to each other.
- Semantic Similarity is used to understand the semantic distance between the concepts in topical maps.
- The title or root of the document is written based on semantic similarity, mainly so that the document can have a proper contextual vector (end-to-end contextual consistency).
- Semantically Similar entities and concepts are included in the websites, or web documents, to increase the overall ranking possibility.
- The new sub-folders that are created in the website leveraged semantic similarity to use “relevance attribution”.
- Relevance attribution attributes the relevance of a document to a concept, to another document for the same topic.
- Thus, a new quality sub-folder helps websites to close the gap, and have higher semantic closeness between different web subfolders.
The Key Points for Semantic Relevance are given below.
- The semantic relevance is different from the semantic similarity. A relevant thing to another doesn’t have to be similar but has to be in a complementary relation.
- The complementary relations between semantically relevant things are used in lexical relations and semantics.
- The lexical semantics help improve the semantic relevance for not leaving a gap in the topical map and the subfolders of the websites.
- Semantic relevance is used to measure the distance from one concept to another.
- The hypernym path or hyponym path types of concepts are used to measure semantic relevance.
- Multi-word phrases, stemming, lemmatization, semantic units inside the words, or word groups help search engines to combine different possible contexts inside the queries.
- Semantic relevance has different directions inside the same contextual domain.
- The semantic relevance direction should focus on the title, and the root of the document so that the contextual vector stays consistent.
- The irrelevant concepts to the contextual domain would decrease the relevance, instead of making the document more relevant to the specific context.
- Use the most relevant concepts, even if there is no search demand or volume, or quality thresholds are too high to improve the semantic relevance.
The Key Points for Lexical Semantics are listed below.
- Lexical semantics and relations are different from query semantics.
- Lexical semantics focus on the word-sense disambiguation, and word theme with connected other words inside a WordNet.
- WordNet involves the word definitions for different contexts and word roles inside different word sequences.
- A verb might change its context based on the noun, or the subject. But, the connections are patterned in semantic role labels and FrameNet.
- Lexical Relations help SEO to improve the overall relevance, and contextual coverage. The context direction within an article, web page, or any kind of rankable document for an Information Retrieval system involves the conceptual graph from one angle to another.
- Lexical relations and semantics for topical maps help to close the gaps while choosing the macro-context, and main entity for the specific web documents.
- Lexical semantics directly affect the internal links, anchor texts, and internal link order, or distance between the internal links.
What are the Lexical Relations between Words?
Lexical relations between words include relations of superiority, inferiority, part, whole, opposition, and sameness between the meanings of words. The same word can be a meronymy, hyponym, or antonym of another word, depending on the word before or after it. The lexical relation value of the first word can affect the structure of the next word, affecting the context of the sentence and the Information Retrieval Score. Information Retrieval Score is the score that determines how much content is related to a query, how close the different variants of the related query are, and the structure processed by the search engine’s query processor to the relevant document. A higher information retrieval score represents better relevance and possible click satisfaction.
The problem with a semi-structured and distracting context for Information Retrieval Score is that, if a document is not configured for a single topic, the IR Score can be diluted by the two different contexts resulting in a relative rank lost to another textual document.
IR Score Dilution involves badly structured lexical relations, along with bad word proximity. The relevant words that complete each other within the meaning map should be used closely, within a paragraph or section of the document, to signal the context in a more clear way to increase the IR Score. A search engine can check whether the document contains the hyponym of the words within the query or not. A possible query prediction can be generated from the hypernyms of the query. A search engine can check only the anchor texts to see whether there is a word within the “hyponym distance” which represents the hyponym depth between two different words.
Lexical Relations can represent the semantic annotations for a document. A semantic annotation is a word that describes the document overall in terms of category and main context that carries the purpose of the document. A semantic annotation can contain the main entity of the document or a general concept for covering a broader meaning area (knowledge domain). Semantic Annotations can be generated with the lexical relations between words. A semantic annotation can be used to match the document to the query. Semantic annotations are factors for a better IR Score.
A search engine can generate phrase patterns from the lexical relationships between words within the queries or the documents. A phrase pattern contains sections that define a concept with qualifiers. Phrase patterns can contain a hyponym just after an adjective, or a hypernym with the antonym of the same adjective. Most of these connections and patterns are used within the Recurrent Neural Network (RNN) for the next word prediction. A phrase pattern helps a search engine to increase its confidence score for relating the document to the specific query, or the meaning of the query.
The importance of Lexical Relations is related to Semantic Annotations, Phrase Patterns, Knowledge Domains, Word Proximity, Structure of the Context, Click Satisfaction, Query Predictions, and Categorical Queries from a topic.
To understand Lexical Relations, the types of lexical semantics between words should be seen.
- Hypernym: The general word of another word. For example, the word color is the hypernym of red, blue, and yellow.
- Hyponym: The specific word of another general word. For example, crimson, violet, and lavender are hyponyms for purple. And, purple is the hyponym for the color.
- Antonym: The opposite of another word. For example, the big is the antonym of the small, and the early is the antonym of the late.
- Synonym: The replacement of another word without changing the meaning. For example, huge is the synonym for big, and initial is the synonym for early.
- Holonym: The whole of a part. For example, the table is the holonym of the table leg.
- Meronym: The part of an entire. For example, a feather is the meronym of a bird.
- Polysemy: The word with different meanings such as love, as a verb, and as a noun.
- Homonymy: The word with different meanings accidentally, such as bear as an animal and verb, or bank as a river or financial organization.
Lexical Semantics is used for generating WordNets to see the meaning of a word within a document as a part of linguistics and NLP. Lexical Semantics and Semantic Networks are related to each other for creating a semantic net with the relevant word relations. A lexical unit refers to two or more words that are related to each other. Lexical units create the lexical semantics within a semantic network that includes a WordNet that is used to understand textual data. A WordNet can be used to find Python, and NLTK to read the documents and see the lexical semantics within them. Synonym or Antonym finding or word similarity measurement can be used by a search engine to match the documents to the queries or cluster them around the centroids of the cluster.
How can Lexical Semantics Help with Semantic Role Labeling?
Semantic Role Labeling is labeling words in a sentence according to their semantic function. The role of the word in the sentence in the semantic context refers to its “label” element in Semantic Role Labeling. It is also called Semantic Role Labeling, Shallow Semantic Parsing, or Slot-filling.
Semantic Role Labeling and Lexical Semantics are interconnected. When one word is juxtaposed with another, it produces a word vector. When a word vector is juxtaposed with another word vector, it produces a context vector. Each context vector in the Knowledge Domain is linked to another. The context in which a word is used, and the context of the surrounding words, affect the context vectors. Hypernym, Hyponym, Polysemy, or Homonym relationships in Lexical Semantics impact the Semantic Role Labeling process in a content snippet.
Semantic Role Labeling and Lexical Semantics show how related words are related to each other, as well as determine what role a word is used in a sentence. For example, the words “door” and “close” are used in different forms and with phrase patterns. “Door is closed”, “George closed the door”, and “Closed doors are opened by the order” sentences change the SRL (Semantic Role Labeling) values for the same words. From these sentences, a Natural Language Processor can see that the “door” is relevant to the “close”, and “door” can be an object, or subject for the “close” verb.
The same verb, “close” can be connected to another noun, such as “eyes”. In this phase, a search engine can create a co-occurring matrix to see the different contextual domains of the “door” and the “eye” with the verb “close”. “Closing eyes” and “Closing doors” are two and two different contexts, even if the word “close” is relevant to both. Thus, generating word vectors and context vectors from them is useful. From next-word prediction or query prediction (next string prediction) to the mid-page query refinements (related search terms), Semantic Role Labeling and Lexical Semantics are used together.
The algorithm, Word2Vec, has been used for many years by Google to see the distance between words within a vector space. Word2Vec is to check whether the word is used within a similar or same context or not with a neural network. Similarly, GloVe is used to check co-occurrence probability within a text span.
A search engine can decrease or increase its confidence score to the relevance score that is determined based on the Semantic Role Labels of the words within the sentence and lexical-semantic relations of the words to each other within the text.
Why Does Word Proximity Matter for Lexical Semantics?
Word Proximity is relevant to phrase-based indexing, and neural network algorithms such as Recurring Neural Networks (RNN). In RNN, the “attention span” can go from left to right, or right to left to see the contextual connections of the words to each other with their Part of Speech Tags and contexts. Word Proximity can have a threshold to relate a document to a query. If a scientist’s name is used far away from another word, the document might not be relevant to the meaning of that word and its relevance to the specific named entity. The specific word can represent an attribute or a dimension of the scientist, but the word proximity and “reference disambiguation” can lead a search engine to see that a document is not relevant to the specific context.
Based on Word Proximity, the impressions the source has from specific queries, the source’s topical relevance, and the documents’ from the source to a specific query category can be seen as relevant, or less relevant.
Especially, the Lexical Semantics and the Word Proximity can signal the relevance of the document to a query, and also a context in a clear way. A document can use different specific words from close contexts in lexically connected ways. A feeling can be seen as an abstract concept, and other abstract concepts can be used close to feelings to explain a macro context. A macro context can have multiple micro contexts within it, in the same document, or within a connected document based on Semantic Annotations.
What is the Semantic Similarity (Closeness)?
Semantic Similarity is the similarity between two nodes within a semantic network based on the connected nodes with similar edges. Semantic Similarity represents the changeability and replaceability of the words and meanings with each other without changing the context, and the meaning of the textual data. Semantic Similarity is a measurable metric for the set of documents or words and concepts from these documents. The distance between two different words is the measurement of the semantic similarity between them. Semantic Similarity can be measured with the help of topological similarity and ontology along with taxonomy. Topical Coverage and Semantic Similarity are connected to each other in the context of semantic similarity. Because atopic borders can be drawn based on the semantic similarity and their own content with the ontology and the taxonomy.
Semantic similarity uses the directed acyclic graph, and the path distance shows the similarity between the two nodes. A lexical unit and the concept can have a proximity location within the vector space model in a correlative way. The vector space model represents the vector space with specific co-occurrence metrics and scores for a text corpus.
Semantic similarity is confused with Semantic Relevance. As Topical Authority and Topical Relevance are not the same as each other, their relevance and similarity are not the same.
What is Semantic Relevance?
Semantic Relevance (Semantic Relatedness) is the metric used to determine the relevance between things. Semantic Relevance can be measured based on the Semantic Role Labels, and word proximity between the words. The semantic role label of the word within the sentence will signal the semantic relevance of different concepts to each other. For instance, the sentences “I will climb a mountain with my shoes”, and “I will run to the forest with my shoes” have different predicates, and themes, but the same tool which is “shoes”. It means that even if “climbing” and “running” are not close to each other, they have semantic relevance because they are both things that can be done with shoes. A question such as “What is necessary to climb a mountain, or run in a forest” can include shoes, easily.
What is the Difference between Semantic Similarity and Relevance?
The semantic relevance and the semantic similarity are different because a similar thing to another might not be relevant. For example, the sentences “I have watched a movie with my glasses”, and “I have watched a bird with my binoculars” have the word “watch” with different “tools” such as a “glass” and the “binoculars”. The “bird” and the “movie” are not semantically similar, but they are both things that can be watched with glasses, or binoculars. The fact is that extraction algorithms can use the “glasses” and the “binoculars” intersections in the context of watching and the nouns. A search engine can store related verbs, nouns, adjectives, and phrase patterns with facts and contexts for different query groups. Thus, factual language with consistent propositions with different contextual domains and knowledge domain possibilities is important to provide a better understanding of a topic overall.
How does Semantic Distance affect Semantic Similarity and Semantic Relevance?
Semantic Distance represents the distance between two concepts or words within the vector space model, or in a WordNet. The semantic distance can be high, but still, the semantic similarity or relevance can be below. Thus, saying that there is a correlation between semantic distance, semantic similarity, and semantic relevance is not correct. For instance, the “antonyms” within a text corpus can be semantically not similar, but still, they can be relevant. And, the semantic distance between the antonyms can be high because their connections to each other won’t be so frequent, but the word’s proximity will be higher.
Thus, when you try to process a document like a search engine, from one angle to another, natural language understanding will have different layers and obstacles. In Semantic SEO, understanding lexical semantics and their connections to each other is helpful to understand the possible relevance factors, and context-qualifying methodologies within a web document for query-document matching.
What is the relationship between Semantic Closeness and Lexical Semantics?
The relationship between Semantic Closeness (Relevance) and Lexical Semantics involves the hypernym, hyponym, synonym, antonym, meronymy, holonym, homonym, and polysemy paths to find relevant semantic meanings within the same context. The path distance represents the Semantic Distance. From the word “love” as a noun to the word “hate” as a noun (hatred), there is a path as “holonym” because they are both hyponyms of “emotion”. Since they are connected in the lexical semantics, the Semantic Closeness will possibly be higher if they are used as the same lexical semantics. If a person uses “love” as a verb while using “hate” as a “noun” within the same text without enough word proximity, the context might not be the emotions. Thus, semantic Closeness (Relevance) Lexical Semantics, and the related concepts from Natural Language Processing such as Semantic Role Labeling, or Semantic Distance are relevant to each other.
In the context of Semantic Search and Semantic SEO, Lexical Semantics and Semantic Closeness can be used to improve topical relevance and topical authority.
How to use Lexical Semantics and Semantic Closeness for SEO?
To use the Lexical Semantics and the Semantic Closeness for SEO, the phrase-based indexing, and the Search Engine’s query generation, and processing methods should be understood.
The usage of the Lexical Semantics for SEO shouldn’t be confused with the old traditions of SEO, such as keyword stuffing. Lexical Semantics is not even related to the “keyword” concept. The queries and meanings from queries can have a place within the perception map based on lexical semantics, and semantic closeness.
A search engine generates queries based on probabilities, and “Incomplete Phrase” understanding. An incomplete phrase involves a phrase without proper grammar rules in a query form. The search engine can complete the query by rewriting it for document matching. An incomplete phrase can be used within the documents to improve the confidence score of the search engine’s query-document matcher. To use the lexical semantics and semantic closeness for SEO, the summative methods below can be used.
- Use the neighborhood words to another word to describe a thing in more detail.
- Order the relevance of lexically relevant words to the targeted word within the query context.
- Rewrite the query within your mind to expand the query for augmented contextual coverage.
- Use the hypernyms with hyponyms and semantic annotations for your internal link creation process.
- Use the synonyms for a better Phrase Rank.
- Give new information with high accuracy for all the sentences to avoid keyword stuffing-related algorithms.
- Take the lexical semantics from software such as TheFreeSaurus or NLTK Corpus.
- Use Stemming and Lemmatization to make the evidence within the web page richer.
- Use phrase patterns and question patterns to make the search engine follow your Semantic Content Network and Topical Map for its own Document-Query Cluster Creation process.
- Generate questions and answers for specifying and sharpening the context.
- Determine the detailed amount for each question with the attributes of the main entity within the question.
- Create Vectors from Word Pairs. Variate the Vectors with different Attention Spans.
- Create a Context Vector from one concept to another to generate questions.
- Order the Anchor Texts with Lexical Hierarchy.
- Align the Lexical Hierarchy with the Anchor Text Hierarchy.
- Use the Different Phrase Patterns from Adjectives, nouns, Cardinals, and more.
What are the main Google Designs that mention Lexical Relations?
From Query Processing to Document Understanding, and entity clustering, lexical relations are mentioned and used in many Google Designs. To see the main Google designs and patents that mention lexical relations, use the list below.
- Dynamic update of an application in compilation and deployment with hot-swapping
- Search queries improved based on query semantic information
- Using geographic data to identify correlated geographic synonyms
- Identifying common co-occurring elements in lists
- Information extraction from a database
- Identifying a synonym with N-gram agreement for a query phrase
- Meaning-based advertising and document relevance determination
- Sub-lexical language models with word-level pronunciation lexicons
- Touch keyboard using language and spatial models
- Contextual dynamic advertising based upon captured rendered text
“The WordNet™ project, for example, is a lexical reference system whose design is inspired by current psycholinguistic theories of human lexical memory. In WordNet™, English nouns, verbs, adjectives, and adverbs are organized into synonym sets, each representing one underlying lexical concept. When using WordNet™, hypernyms of a word to a query (e.g., alcohol is a hypernym of wine) and/or hyponyms (e.g., burgundy is a hyponym of wine) can be used to generate terms related to the initial query. WordNet™ is developed by the Cognitive Science Laboratory of Princeton University… In addition to determining the possible alternate terms to the user query, query processing component 121 may also generate a set of documents that define a “query context” for the initial search query (Act 302). Act 302 may be performed simultaneously with, before, or after Act 301. In one implementation, the query context may be a set of documents returned by ranking component 122 based on the initial user search query. The set of documents may be limited to the most relevant N documents returned by ranking component 122. In one implementation, N is set between 30 and 50 documents, although in other implementations, other values of N may be used.”
Search queries improved based on query semantic information
“The preceding discussion described a generalized technique for discovering and generating context-sensitive synonyms. This technique is very useful, but it fails to take into account an important signal—lexical relationships between words. Consequently, the above-described technique misses many useful stems and other lexically similar words.
The following discussion remedies this problem by considering special classes of highly-trusted synonyms which are lexically related to the original word. We treat these synonyms differently and try to be more inclusive of them in our data. These lexically related words can be categorized in various ways, but all share the trait of their being a lexical connection between the original word and the synonym.”
A simple approach is to use pre-constructed synonym information, for example, from a thesaurus or a structured lexical database. However, thesaurus-based systems have various problems, such as being costly to construct and being restricted to one language… The preceding discussion described a generalized technique for discovering and generating context-sensitive synonyms. This technique is very useful, but it fails to take into account an important signal—lexical relationships between words. Consequently, the above-described technique misses many useful stems and other lexically similar words.
The following discussion remedies this problem by considering special classes of highly-trusted synonyms which are lexically related to the original word. We treat these synonyms differently and try to be more inclusive of them in our data. These lexically related words can be categorized in various ways, but all share the trait of their being a lexical connection between the original word and the synonym:
(1) synonyms that share the same stem as the original word, according to an industry-standard lexical stemming technique (e.g. car→cars).”
Identifying common co-occurring elements in lists
“A pattern is a template for finding additional tuples of information that may satisfy the desired information. A pattern typically needs to be lexically defined because it will be utilized to find the desired information in the books. The lexical definition of a pattern can largely determine how successful the information extraction will be. However, even a very simple lexical definition of a pattern that will be used for this example can generate excellent results. More sophisticated lexical definitions of patterns may provide better results. An occurrence can be lexically defined in correspondence to the definition of a pattern. An occurrence of an (author, title) pair can consist of a seven-tuple: (author, title, order, URL, prefix, middle, suffix). The order corresponds to the order in the author and title that occurred in the text. The URL is the URL of the document in which they occurred. The prefix includes the m characters (e.g., 10) preceding the author (or title if the title was first).”
Information extraction from a database
“Then, for each term in the query phrase, the system determines whether the term is a lexical synonym of a corresponding term in the candidate synonym or the term shares meaning with the corresponding term in the candidate synonym… In a variation on this embodiment, the system determines whether a query term and a synonym term are lexical synonyms or share meaning by lexically comparing the two terms.
In a further variation, the system determines whether a query term and a synonym term are lexical synonyms or share meaning by checking if the query term and the synonym term share a common stem…”
Identifying a synonym with N-gram agreement for a query phrase
“The quality of the results obtained varies, but by doing essentially sub-string matches or category browsing, the engines are unable to properly discern what the user actually intends or means when a particular keyword is entered. Thus, the response to search terms entered is a list of documents/sites that may bear little relation to the intended meaning/usage of the term(s) entered… One corollary response to search terms input into the search engine is the retrieval and display of advertising icons, often referred to as “banner ads.” One ad-buying model for companies and websites desiring to advertise on a search engine is to purchase one or more search terms. When the search term(s) is input by a user of the search engine, the corresponding banner ad is displayed…”
Meaning-based advertising and document relevance determination
“The process includes accessing a word level pronunciation lexicon and a word level training text corpus for a natural language; segmenting, using a word decomposition system, the word level training text corpus into sub-lexical units; training an n-gram language model over the sub-lexical units to produce a sub-lexical language model; constructing, using the word decomposition system, a word to sub-lexical unit mapping transducer; constructing a word level language model by composing the mapping transducer with the sub-lexical language model; and constructing a speech decoding network by composing at least the word level pronunciation lexicon, and the word level language model…”
Sub-lexical language models with word-level pronunciation lexicons
“lexical cost values may be based on language model 111. For instance, the respective lexical cost values may be based on the probability that a second key will be selected after a first key (e.g., the probability that the “o” key will be selected after the “c” key). In other examples, the respective lexical cost values may be based on the probability that a second candidate word will follow a first candidate word, or on the frequency values of language model 111. In certain examples, the keys for which respective lexical cost values are determined are selected based at least in part on language model 111. In some examples, the lexical cost values are lower where there is a greater likelihood that a tap gesture indicates a key. In other examples, the lexical cost values are higher where there is a greater likelihood that the tap gesture indicates a key.”
Touch keyboard using language and spatial models
“The system may generate keywords for an identified document in a number of ways. For example, the system may analyze the text of the document and perform iterative processes to determine one or more keywords for the document. The frequency (or lack of frequency) of words may be considered. The system may also look at the metadata associated with the document. For example, the document may already provide keywords within the metadata or may provide references related to the document. Additionally, the system may perform inverse document frequency analysis, lexical and semantic analysis, and/or document summarization techniques when analyzing the content of a document…”
Contextual dynamic advertising based upon captured rendered text
From guessing the next letter on your keyboard touch, or showing the related advertisement documents, synonym finding, query context extraction, pattern extraction, tuple creation, and relational database understanding, the lexical relations are used. Thus, a search engine algorithm and technology are used for multiple purposes as long as it helps search engines to improve their products.
What is the History of the Lexical Semantics?
Many theories of lexical semantics make use of lexical decomposition, which has its historical origins partly in French, and English linguistics (Algirdas Julien Greimas, Bernard Pottier), and partly in generative semantics. Word meanings are derived from an arrangement of primitive meaning modules (semantic features, semes). For example, the lexical structure of the verb “kill” is more complex than that of the verb “read”, because “kill” is a causative verb made up of the constants which are “CAUSE (x)” and “BECOME (DEAD (y))”, “read” on the other hand only consists of an activity constant “DO (x, y)”. So the variables “x” and “y” represent the arguments of the verb. In more recent approaches, decomposition structures are also reflected syntactically, so that the combinatorial behavior of lexical units systematically results from the interplay of syntactic and lexical principles.
Lexical semantics (including word semantics ) is a portion of linguistics. It deals with the meaning of the individual lexical elements (words, morphemes, lexemes). Assuming that the meanings of words are recorded, the question is asked how the meaning of a word relates to the meaning of the sentence (Frege’s principle of compositionality ). For this purpose, the combinatorial properties of lexical units are examined, which enable them to form more complex units—such as compound words, phrases, or sentences. An essential component of a meaningful theory of lexical semantics must therefore be the mapping of lexical-semantic to syntactic structures—the so-called linking. So there is a competition between syntax and lexical semantics as to which of the two systems produces or generates certain complexes. For example, it has not been clarified whether complex verbs of the type lean are to be regarded as a product of syntactic structure formation (for example, the separability of the particles speaks for this) or of lexical structure formation (this is suggested by the word status of such particle verbs). The lexical semantics assumes a similar interface status with regard to the morphological module of the grammar. This raises whether morphological operations can in principle be understood as mechanisms of the system of grammar—this would mean that morphological and lexical-semantic operations would coincide—or whether a strict distinction should be made between the two types of grammatical structure formation.
What are the Reference and Sense for Lexical Semantics?
Traditionally, lexical semantics distinguishes between reference and sense, as articulated most influentially by Frege and Saussure. An object, event, or state that a word points to is its reference. Essentially, it is what the word implies, and it exists outside the mind. Therefore, if someone uses the word chair to refer to a particular piece of wooden furniture with four legs and a back, the reference is to that object in the world. By contrast to the sense of a word, the sense of a word is its meaning in relation to its place in a linguistic system, and this meaning lies within the mind. A chair’s exact meaning in English relates to the meanings of other words such as beanbag, bench, pew, seat, sofa, stool, and so on. Translation equivalents are rarely 100% accurate due to differences in the meaning of words across languages, in part because of the relational aspect of meaning. An English term chair might be translated to mean both chairs and stools in a given language, or there may be two terms referring to chairs of different types. Furthermore, different words with different senses can refer to the same person in the world, such as Bill, Shakespeare, Ann’s husband, Hamlet, or the Bard of Avon. All of these terms may have different implications for the same person. It’s quite different from the repetition of terms when two senses signify the same thing. Even though those who are unaware that the terms ‘morning star’ and ‘evening star’ refer to the same object refer to the planet Venus as the morning or evening star, example conveys knowledge and example does not.
Below, there are two example propositions for the comparison between sense and reference.
- The morning star is the evening star.
- Venus is Venus.
As a result of the distinction between sense and reference, two distinct semantics research traditions have emerged. Reference (denotational) theories of meaning consider how words determine what they refer to. In formal semantics (e.g., Chierchia & McConnell-Ginet, 2000), and in Montague Grammar (Montague, 1974), the legitimacy of expressions is determined by whether they express an externally meaningful logical and true expression. Truth-conditional semantics, for example, sees nouns as denoting actual entities and verbs as denoting situations. True or false, they can either describe the way reality is or how it isn’t. Using this traditional view, meaning is limited to an idealistic conception of language independent of differences in speakers’ minds, such that languages are viewed as abstract and ideal examples instead of representations of psychological or sociological characteristics (Lewis, 1972, p. 170). The second is representational theories of lexical semantics, which are in contrast to denotational theories that do not consider any aspect of meaning as external to the mind. Accordingly, one cannot measure the truth of a linguistic representation against reality, but only against other mental representations of the world. As a function of shape, texture, color, perspective, etc., we perceive a chair and categorizing it is similarly a cognitive activity. The lexicon, which serves as the link between phonological, syntactic, and conceptual representations of words, lies at the center of lexical-semantic research. Jackendoff (1989, p.74) draws on Chomsky’s (1986) concepts of e-language (external, language as a social abstraction out there in the world) and i-language (internal, language as a cognitive construct). Representational theories are connected to E semantics, while denotational theories are related to semantics. In that sense, this chapter examines I-semantics, as well as lexical-semantic theories that have emerged from that tradition.
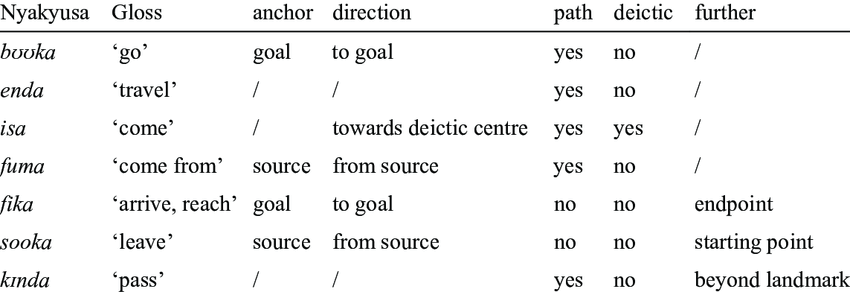
Lexical semantics deals with the meaning of words. Within lexical semantics, the main topics studied involve either the internal semantic structure of words or the semantic relationships within the vocabulary. The first set of phenomena includes polysemy (as opposed to vagueness), metaphor, metonymy, and prototypicality. A substantial part of the second set is devoted to lexical fields, lexical relations, conceptual metaphors, and frames. In the history of lexical semantics, three main theoretical approaches have succeeded each other: structuralist historical semantics, structuralist semantics, and cognitive semantics. They differ in taking a usage-oriented rather than a system-oriented approach to word-meaning research, but they have both contributed significantly to the description and conceptual apparatus in lexical semantics dating back to the historical development of the field.
Lexical semantics deals with the meaning of words. Within lexical semantics, the main topics studied involve either the internal semantic structure of words or the semantic relationships within the vocabulary. The first set of phenomena includes polysemy (as opposed to vagueness), metaphor, metonymy, and prototypicality. A substantial part of the second set is devoted to lexical fields, lexical relations, conceptual metaphors, and frames. In the history of lexical semantics, three main theoretical approaches have succeeded each other: structuralist historical semantics, structuralist semantics, and cognitive semantics. They differ in taking a usage-oriented rather than a system-oriented approach to word-meaning research, but they have both contributed significantly to the description and conceptual apparatus in lexical semantics dating back to the historical development of the field.
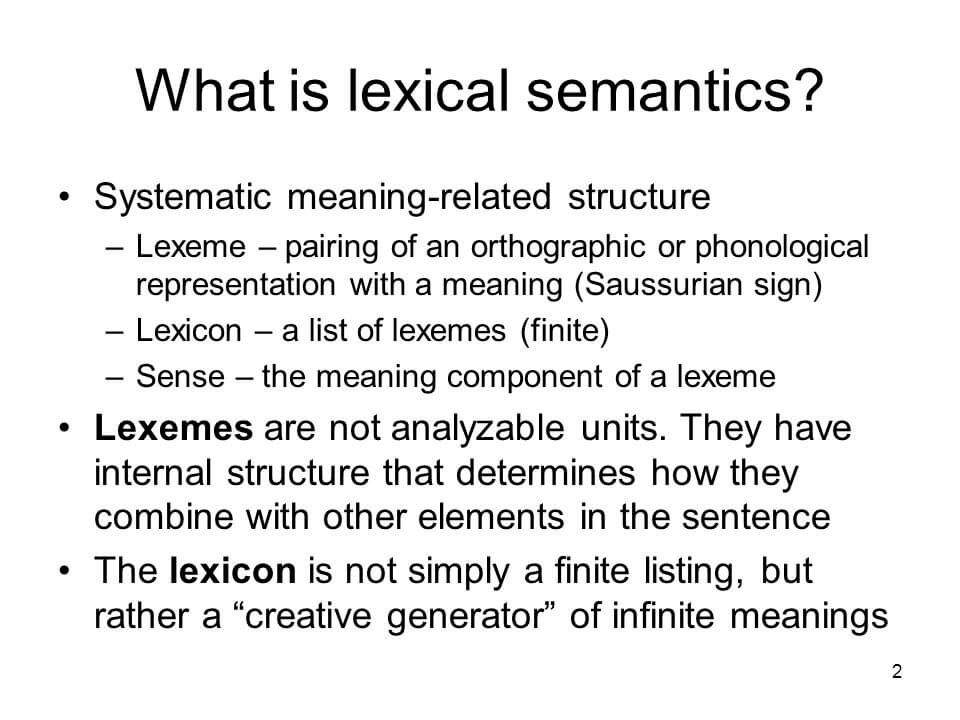
What are the Areas of Application of Lexical Semantics?
Areas of application of Lexical Semantics are listed below.
- Different methods of describing the meanings of words
- The traditional description of the meaning
- Word fields
- Component analysis
- Semanalysis
- Lexical ambiguity ( ambiguity )
- Fuzziness (language)
- Change of meaning
- Argument structure ( thematic role )
- Semantic relations (meaning relations ) between concepts and words such as hyperonymy; Hyponymy; Synonymy or antonymy.
What are the Related Terms for Lexical Semantics?
Lexical Semantics is used for Natural Language Processing and Natural Language Understanding. Below, there are related terms for lexical semantics in the context of NLP and NLU.
- Named Entity Recognition
- Semantic Role Labeling
- Semantic Parsing
- WordNet
- FrameNet
- Tokenization
- Lemmatization
- Stemming
- Semantic Network
- Syntax Tree
What is the Relation Between Lexical Semantics and Semantic Role Labeling?
Semantic role labeling is connected to Lexical Semantics because lexical semantics can change the semantic role label of a word within a sentence. Semantic Role Labeling is the process of giving a role to a word in a sentence, according to its role for the predicate of the sentence. Since all the words change their meaning according to the lexical semantics, the roles will be determined differently. For Semantic Role Labeling, arguments are used in the scope of lexical semantics. Arguments are participants who are integral to the event or state expressed by the predicate, and argument structure describes the relationship between the verb and the participants it selects. In several influential approaches to understanding argument structure, participants are characterized through their roles rather than by features. Thematic roles are determined by the lexical semantics of the verb and mapped to syntax by means of a thematic hierarchy, whereby the highest role is associated with the subject position, and the next highest is associated with the object position.
As an example of lexical semantics, the word “bachelor” refers to a male, human, animate, and unmarried person. In place of using word hierarchies to represent such relationships, the word itself contained these elements as interpretable features. In terms of lexical relations, semantic features provide a way to reconceptualize lexical items. Below, there are some lexical-semantic examples of the words “man”, “bachelor”, and “boy”.
- man [+MALE] [+ADULT] [+HUMAN]
- bachelor [+MALE] [+ADULT] [+HUMAN] [+UNMARRIED]
- boy [+MALE] [-ADULT] [+HUMAN]
According to the sentence, and predicate of the sentence, these words will have different semantic role labels and meanings. Below, there are some semantic role-label examples based on lexical semantics.
According to this approach, thematic roles are determined by the lexical semantics of verbs, and the mapping to syntax occurs via a thematic hierarchy where the highest role relates to the subject, the next highest relates to the object, and the lowest relates to the indirect object positions.
Last Thoughts on Lexical Relations and Holistic SEO
Last Thoughts on Lexical Relations and Holistic SEO involves the benefits of the lexical semantics for search engines, and search engine optimization. Holistic SEO involves Semantic SEO efforts as part of the multistaged and perspective SEO strategy and mindset. In semantics, lexical relations are used to understand the topic, sub-topics, entity-predicate-attribute patterns, query rewriting, information retrieval, and extraction. Understanding Lexical Relations is useful for creating topical maps, or semantic content networks. While designing a content network with semantics, sentence optimizations with micro-semantics and overall document optimizations with macro-semantics are used. Lexical relations are related to the micro and macro semantics from sentence optimization to entire document, and document network optimization.
Lexical semantics helps Search Engines understand the content better through Natural Language Processing. At the same time, a search engine that better understands the concepts in queries with lexical semantics improves the quality of search results by understanding the query better. Lexical Semantics are basic concepts within the scope of Semantic Search, Semantic Search Engines, and Semantic SEO. A holistic SEO needs to understand Lexical Semantics, evaluate content in this context, make word choices, and create the necessary word and meaning bonds for semantic role labeling.
In this SEO Case Study, I have provided three main things. Practical SEO Projects with positive success stories and testimonials that praise the semantic SEO methodology and Holistic SEO mindset, Google search engine designs, and patents to show the prominence of lexical relations for multiple angles, and definitions of the lexical relations along with how they are helpful for search engines are these three main things.
In future SEO Case Studies, some of these websites’ end results will be published.
- Sliding Window - August 12, 2024
- B2P Marketing: How it Works, Benefits, and Strategies - April 26, 2024
- SEO for Casino Websites: A SEO Case Study for the Bet and Gamble Industry - February 5, 2024
Amazing Work Brother
Thank you so much, Fahad!
nice
Thank you so much, Jaqesh!
These are valuable insights, Koray, and I am grateful you share so much free of charge. You are very good at presenting information while remaining focused on the facts. This is true both in your writing and in your videos.
I have a question regarding the scope of your topical map for Serapool. I see that recent posts in the blog are titled “The Benefits of Swimming” and “What Are Swimming Styles?”. Being that this is a website selling pool construction materials, it would seem that these posts fall far outside what a typical SEO would consider topics related to pool construction materials.
My question is: Aren’t there many other intermediary topics closer to “pool construction materials” that could be written about to create a sufficiently broad topical map?
Thank you for sharing your knowledge,
James
Hello James Ulysse,
First of all, thank you for your kind and supportive words for our SEO Case Study. It means a lot to us.
Second of all, the Serapool is a B2B Business project, and you are right that it focuses on pool construction, and pool construction materials, but at the same time, it focuses on pool types, pool designs, pool sizes for hotels, rooftops, or party organizations. Sometimes, even party organizations re-construct or re-design their pools based on light changes, or the community that they are having as guests. Thus, to provide further topical relevance and contextual coverage, the “swimming in X type of pool with P amount of people” context is united with the construction of an “Olympic pool”, or “Olympic pool” material.
This is about how to expand a topical map based on correlative search queries and sessions. And, one of the incoming SEO Case Studies will be about this, I hope you like it as well. Lexical relations, and lexicosemantics help to see these context bridges between different knowledge verticals.
The emphasis on Semantics over Links is because it is Google’s business model to increase Ads, whereas Quality would actually be better served if Links were given a higher priority, ( – as you said in a video, (https://youtu.be/XL2aL7P7ZUI?t=4192I – correct me if I’m wrong?!). Could you say any other consequences that might follow from this fundamental factor, such as whether ultimately it may prove to be unsatisfactory for Google and subject to updating or something? Btw, Amazing work, and proper science; speaking of quality, it’s so hard to find reliable information in the whole Google (i.e. the whole web experience) mystery!!
Hello Lawrence Mcdonnel,
Thank you for your comment, and creatively unique, and valuable question about Semantics.
Google uses semantics on content, and also links the graph’s sub-parts. If a link is semantically connected to another one, or if it makes sense between the link target and the link source, it means that the navigation between the source and target pages makes sense. Google has to understand semantics to serve the most relevant and satisfactory ads on the pages with natural placement. And, with the bloating web, and increased use of similar language models, Google has to re-balance the importance of PageRank for prioritizing the web sources over time. I hope this answer helps you.
Great work as always. Thank You.
When do you think your course will be coming out?
Great work as always Koray. Thank You.
Your case studies are very insightful and your results speak for themselves.
When do you think your course will be coming out?
Can you show a before and after example of a piece using lexical semantics? or a basic process for creating content using lexical semantics?
Hi koray, thanks for sharing this valuable information and those amazing results.
I still confused about the definition of “semantic closeness”.
As far as I understood throughout the article, closeness is another word for similarity, but I got confused when I read the section under the title
“What is the relationship between Semantic Closeness and Lexical Semantics?”
because you are putting it like
“Semantic Closeness (Relevance)”
Which seems like semantic closeness means semantic relevance, not similarity
awesome
Thank you!