Explainable AI (XAI) is the creation of artificial intelligence systems that provide explicit and understandable explanations for their decision-making processes. addresses AI algorithms’ demand for transparency and interpretability by bridging the complexity-comprehensibility gap.
The value of explainable AI resides in its capacity to improve regulatory compliance, trust, and accountability in AI systems. Users, stakeholders, and decision-makers better comprehend the thinking behind AI decisions due to XAI’s explanations for such decisions. Transparency is essential in vital industries that include healthcare, finance, and autonomous vehicles, where trust and accountability are necessary.
There are multiple benefits to explainable AI. Firstly, explainable AI encourages openness by demystifying AI model decision-making and making the technology more comprehensible. It increases user trust and acceptability. The ability to clearly explain AI-driven conclusions when needed by law or ethical principles is another way explainable AI helps enterprises adhere to rules. XAI makes bias reduction and detection easier, promoting equality and reducing discrimination in AI systems. It offers interpretability and insights into model performance, which aid data scientists in debugging and enhancing models. Lastly, explainable AI gives users more control by helping them to comprehend the variables taken into account when making decisions based on AI suggestions.
There are numerous case studies of explainable AI in use across various industries. XAI assists doctors in interpreting medical image analysis data, providing insights into the AI algorithms’ diagnoses. Explainable AI is capable of identifying suspicious activity and identifying fraudulent transactions in the financial sector. XAI clarifies the decision-making process of self-driving automobiles, assisting users in understanding the justification for key decisions with regard to autonomous vehicles. Explainable AI adds to fairness and accountability in legal and compliance environments by providing open justifications for AI-generated evidence or predictions.
The capabilities of explainable AI differ based on the approaches and procedures used. Techniques for model interpretation offer insights into how models function, such as the significance of features and decision-making processes. Local explanations justify specific forecasts or choices, illuminating the variables affecting particular results. Global illustrations examine trends and connections in the data to understand better how a model works.
Rule extraction techniques try to extract decision trees or rules that are easily understood by humans from complicated AI models, improving interpretability. Explainable AI frequently uses visualization techniques to represent complex data and model behavior visually. Remember that explainable AI’s capabilities and methodologies are constantly changing as researchers create new strategies.
What is Explainable AI?
Explainable AI (XAI), otherwise referred to as interpretable AI, is the creation of artificial intelligence systems that provide explicit and understandable explanations for their decision-making processes. XAI tries to overcome conventional AI systems’ “black box” characters, which frequently make intricate judgments without disclosing their reasoning. XAI aims to close the gap between the need for decision-making that requires transparency, interpretability, and human understanding and the intrinsic complexity of AI models.
Explainability in the context of XAI refers to the capacity of AI systems to offer meaningful and understandable justifications for their results. The justification includes outlining the rationale behind a particular choice or prediction, the elements or circumstances that shaped the choice, and the steps the AI model takes to reach its results. The explanations make it easier for users, stakeholders, and decision-makers to comprehend, believe, and validate AI system results.
There are several reasons why explainable AI is necessary. Understanding the thinking behind AI judgments is essential as AI spreads and is integrated into necessary fields, including healthcare, finance, and autonomous cars. Interpretability is crucial for fostering acceptance and trust in AI systems, ensuring fairness, preventing biases, and abiding by moral and legal obligations. XAI allows users to understand the logic, underlying patterns, and decision rules employed by AI models by giving explanations, empowering them to make educated decisions and take appropriate actions based on AI-generated insights.
What is the Purpose of Explainable AI?
The purpose of Explainable AI (XAI) is to address traditional AI algorithms’ “black box” character by offering clear and intelligible explanations for their decision-making processes. The main goal is to close the knowledge gap between the complexity of AI models and the demand for openness, interpretability, and human comprehension.
The main purpose of XAI is to improve AI system trust, accountability, and regulatory compliance. XAI helps consumers, stakeholders, and decision-makers comprehend the justification for AI decisions by offering explanations. Transparency is essential in healthcare, banking, and autonomous vehicles, where AI judgments have far-reaching effects.
The need for XAI is mainly fueled by trust. XAI contributes to demystifying the decision-making process and the growth of AI system confidence by offering explanations. The acceptance and usage of AI technology have risen as a result.
Accountability is another goal of XAI. Regulatory requirements exist in a number of industries, including finance and healthcare, for the justification of AI system judgments. XAI offers a method for outlining and defending actions to regulatory bodies and stakeholders while assisting enterprises in adhering to their requirements.
XAI is essential in locating and eliminating bias in AI systems. XAI facilitates the detection of biased behavior and aids in comprehending the variables that contribute to it by offering explanations. It allows enterprises to identify and correct biases, assuring justice and averting prejudice in AI-driven decision-making.
XAI is an excellent tool for troubleshooting and enhancing AI models. Data scientists discover and fix any problems or biases by using XAI approaches’ interpretability, which makes it easier for them to grasp the advantages and disadvantages of their models. Enhancing the performance and dependability of AI systems requires the iterative model refinement process.
XAI empowers people by offering insights into AI suggestions and decision-making processes. Users who understand the aspects that AI models take into account make better judgments and take the necessary actions based on that knowledge.
Why Does Explainable AI Exists?
Explainable AI (XAI) exists to solve the inherent complexity and lack of transparency in traditional AI systems. The requirement to offer concise and accessible justifications for AI decision-making processes led to the creation of XAI.
XAI was developed to get over the “black box” character of AI systems, where the algorithms’ inner workings are frequently complicated and challenging to understand. Traditional AI models perform well and reach high accuracy, but they sometimes need more transparency, making it difficult for consumers to comprehend how and why particular decisions are made. The lack of interpretability makes AI systems less trustworthy and less accepted, make it more difficult to comply with regulations, and raises questions about prejudice and discrimination.
The uses of Explainable AI (XAI) are diverse and have broad applications across various domains. XAI promotes trust in AI systems by explaining their decisions and improving acceptance and adoption. It aids regulatory compliance in industries such as banking and healthcare by justifying and explaining AI-driven judgments to regulatory agencies. XAI is essential for identifying bias in AI systems, resolving it, fostering fairness, and eliminating prejudice. It aids in debugging and improving AI models by enabling interpretability to discover strengths and flaws. XAI empowers end users by providing insights into AI recommendations, allowing for informed decision-making. XAI encourages accountability by allowing users and stakeholders to examine and confirm the justifications underlying AI actions.
What is the Value of Explainable AI?
Explainable AI (XAI) is valuable because it solves key problems with conventional AI systems, namely their need for more transparency and interpretability. XAI offers several advantages by providing concise and intelligible justifications for AI actions.
Trust is one of XAI’s core values. XAI contributes to increasing confidence in the technology by explaining AI decisions. Users and other interested parties comprehend the decision-making process, increasing trust in AI systems’ accuracy and fairness. The adoption and acceptance of AI technologies across various fields are encouraged by increased trust.
Other fundamental XAI ideals are responsibility and transparency. XAI enables enterprises to comply with regulatory standards in industries with regulatory constraints, such as finance and healthcare, by explaining AI-driven judgments. It guarantees decision-making procedures are transparent and enables appropriate justifications and auditing. XAI aids in the identification and mitigation of biases in AI systems, increasing justice and reducing prejudice.
XAI provides value by empowering users. Users obtain insight into the decision-making process by understanding the aspects that AI models take into account through the explanations supplied by XAI. They are better equipped to make wise choices, act appropriately, and have faith in the insights produced by AI.
XAI supports model development. Data scientists better comprehend the advantages and disadvantages of their models because of the interpretability offered by XAI approaches. It allows them to improve performance, pinpoint problems, and optimize AI algorithms.
What are the Benefits of Explainable AI?
Listed below are the benefits of Explainable AI.
- Transparency: XAI promotes transparency by providing concise and explicable justifications for AI choices. Users learn more about the decision-making process, comprehend the considerations taken into account, and see how the AI model arrived at a specific result.
- Trust and Acceptance: XAI fosters trust and acceptance in AI systems by demystifying decision-making. Users are more inclined to trust the technology and feel secure in relying on AI-generated insights when they understand and analyze the outputs of the technology.
- Regulatory Compliance: XAI assists businesses in adhering to laws that demand justifications for decisions made using AI. XAI improves regulatory compliance and ensures accountability in sectors with legal and ethical standards by offering clear justifications.
- Bias Detection and Mitigation: XAI helps identify and correct biases in AI systems. XAI enables companies to correct biases and advance fairness in decision-making by identifying the variables causing biased behavior through explanations.
- Model Debugging and Improvement: XAI approaches offer interpretability, enabling data scientists to debug and enhance AI models. Data scientists find flaws, improve performance, and increase the dependability of AI systems by understanding the inner workings of the models and the elements that affect their judgments.
- User Empowerment: XAI gives people more control by justifying AI suggestions and judgments. Users better employ AI technology to support their decision-making processes when they have clear insights into the reasons behind the outputs that the technology produces.
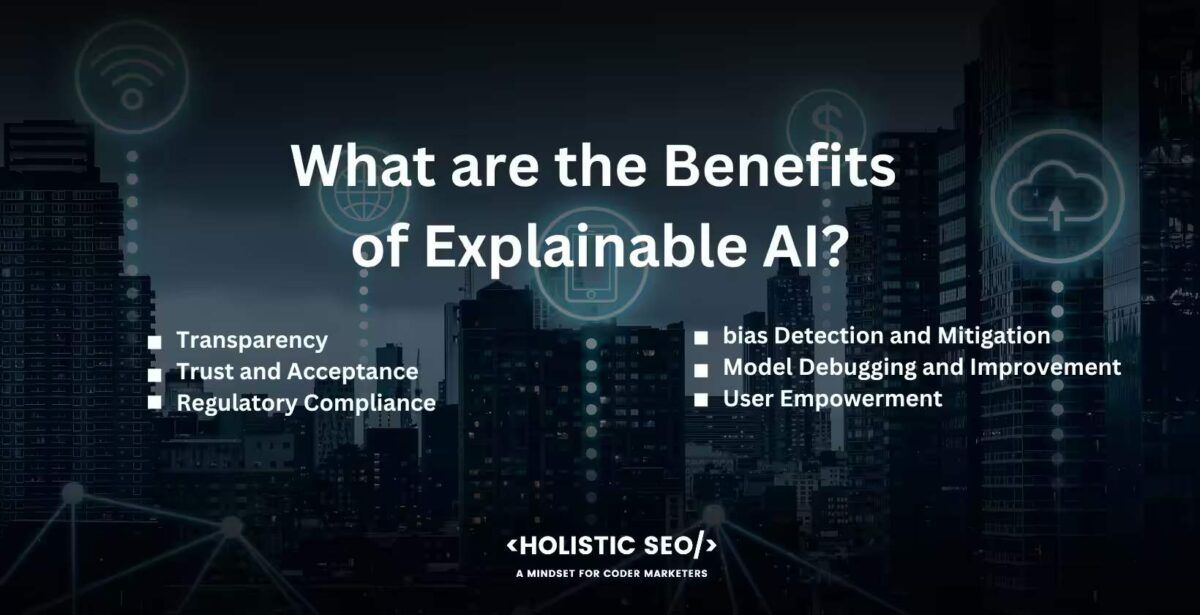
What are Examples of Explainable AI Cases Studies?
Listed below are the Examples of Explainable AI Cases Studies.
- Healthcare: XAI is applicable to medical picture analysis, where AI tools help clinicians identify disorders. Increased confidence and improved collaboration between AI and medical professionals are made achievable by the explanations supplied by XAI approaches. It assists doctors in understanding the traits and trends that influence the AI system’s diagnosis.
- Finance: XAI is applied to the financial sector to detect fraud. Financial institutions make informed decisions and lower false positives by understanding the reasoning behind AI-driven fraud warnings with the aid of XAI’s explanations for the variables that go into the identification of suspicious actions.
- Autonomous Vehicles: The development of self-driving cars is greatly aided by XAI. XAI ensures that consumers understand why specific actions were done, fostering trust and safety in autonomous driving technology by explaining the decision-making process of AI algorithms in real-time scenarios.
- Legal and compliance: XAI offers support in court proceedings involving forecasts or evidence produced by artificial intelligence. XAI approaches’ explanations make it easier for judges and attorneys to comprehend the thinking behind AI-driven decisions, promoting justice, accountability, and transparency in courtroom procedures.
- Service to customers: XAI is used in chatbots for customer service to explain the responses provided by the AI system. XAI improves the user experience and aids users in comprehending the reasoning behind the chatbot’s recommendations by offering justifications for the suggested responses or activities.
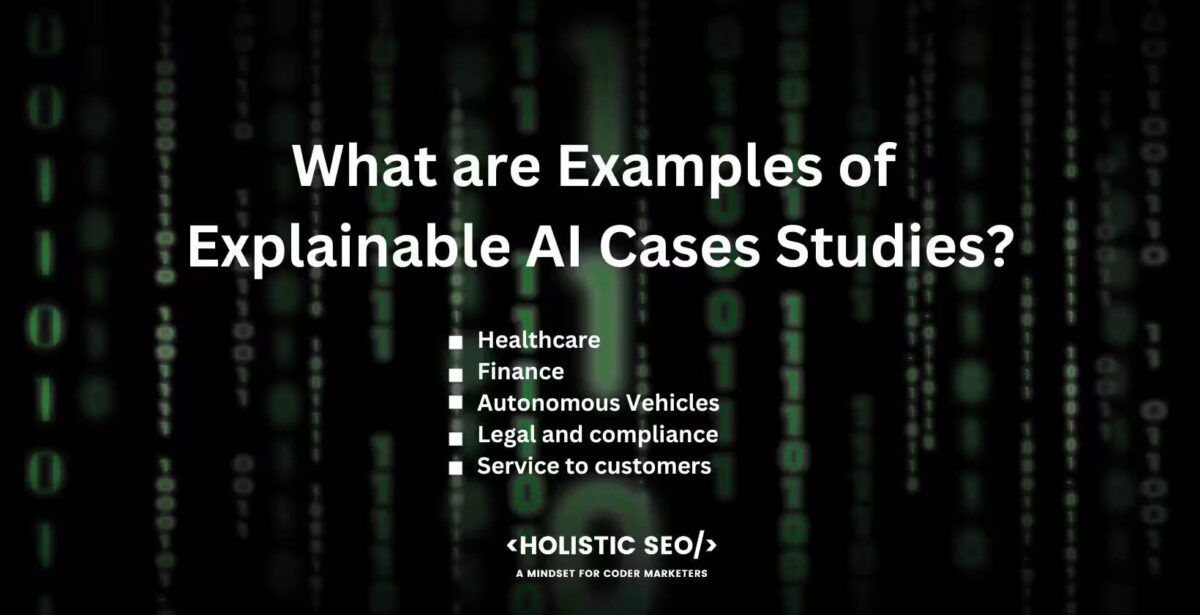
What are the Capabilities of Explainable AI?
Listed below are the Capabilities of Explainable AI.
- Model interpretation: XAI approaches to shed light on the inner workings of AI models. It entails determining the significance of a feature, comprehending its contribution, and disclosing the model’s decision-making processes or patterns.
- Local Explanations: XAI offers justifications for specific judgments or predictions. It aids users in comprehending the variables that affected a given result, giving them insights into the AI model’s logic in that unique situation.
- Global Explanations: XAI offers a comprehensive explanation of a model’s behavior. XAI approaches to uncover the overall decision-making processes and tendencies of the AI model by examining patterns and relationships in the data.
- Rule Extraction: A few XAI techniques are designed to take complicated AI models and turn them into human-readable rules or decision trees. It makes the models more interpretable and intelligible because they are expressed in a format similar to classic rule-based systems.
- Visualization: XAI frequently uses graphics to describe complex data and model behavior intuitively. Visualization techniques aid users in understanding and interpreting AI outputs by providing graphical representations of the decision-making process or feature significance.
- Counterfactual Explanations: XAI generates counterfactual explanations, which include presenting alternative hypotheses that have produced certain results. Users better comprehend the decisions made by the AI model by describing the influencing elements.
- Estimating Uncertainty: XAI sheds light on the degree of uncertainty in AI predictions. It entails quantifying the degree of assurance or probability distribution of predictions, assisting users in evaluating the dependability of the outputs of the AI system.
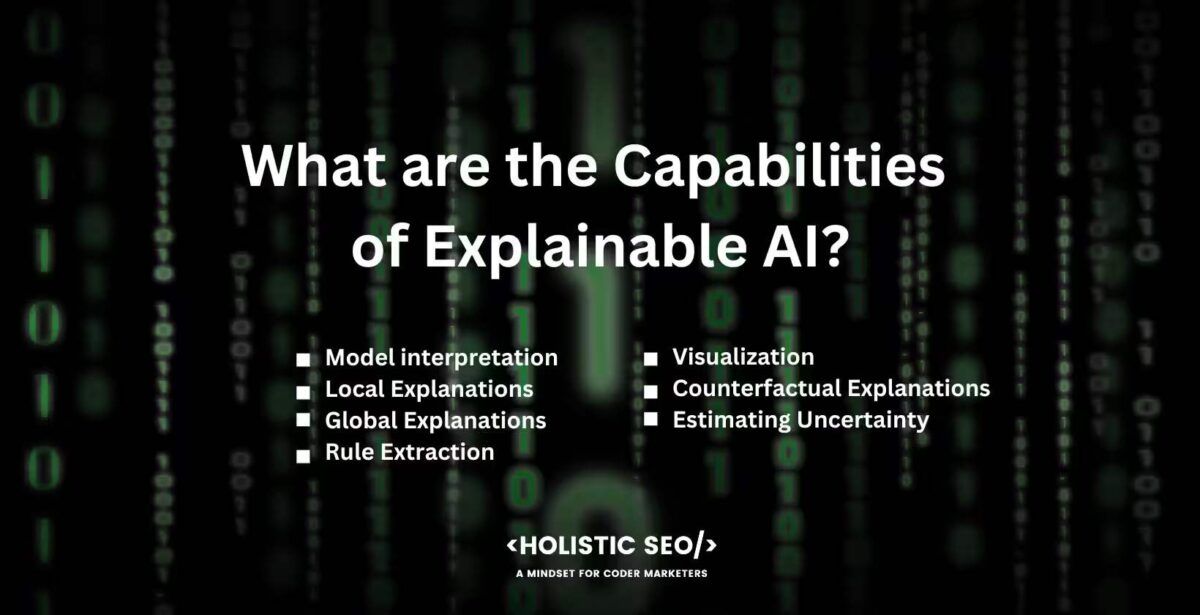
What are Factors to Consider Explainable AI?
Listed below are the factors to consider in Explainable AI.
- Interpretability Techniques: It’s essential to comprehend the various XAI approaches and procedures. Consider the interpretability methods that apply to the AI models they are developing, such as model interpretation, local and global explanations, rule extraction, visualization, counterfactual explanations, and uncertainty estimates.
- Domain Relevance: Transparency and interpretability standards and criteria vary across different domains. Determine the level of explainability required to achieve regulatory compliance and stakeholder expectations by taking into account the specific domain in which XAI is deployed.
- Performance-Interpretability Trade-off: It’s critical to take model performance and interpretability into account. Some XAI methods make AI models more complex or less accurate. Assess the balance between model performance and interpretability depending on the particular environment and use case.
- User Needs and Understanding: Recognize the requirements and hopes of the people utilizing the AI system. Take into account their level of technical knowledge and comprehension of AI ideas. Ensure that the XAI explanations are appropriate for the users’ understanding and that they are clear and meaningful to them.
- Ethical Considerations: Consider the ethical implications of AI decisions and their influence on various stakeholders. Fairness is ensured, biases are reduced, and prejudice is avoided using XAI. Consider ethical rules and principles when creating and deploying XAI systems.
- Scalability and Performance: Assess the XAI approaches used for scalability and performance. Consider the computational resources required to generate real-time explanations or handle enormous datasets. Ensure the XAI technique one chooses manages the requirements of their particular application.
- Integration and Adoption: Consider integrating XAI into current AI workflows or systems. Analyze how simple it is to integrate XAI approaches into the current systems and workflows. Ensure that XAI is compatible with organizational objectives and is successfully incorporated into the decision-making process.
- Validation and Verification: Create procedures for approving and confirming the justifications offered by XAI. Examine the XAI approaches’ dependability and robustness to make sure the explanations are reliable and correct. It entails running studies, interviewing users, or contrasting explanations with facts.
How Does Explainable AI Work?
Explainable AI (XAI) works with many strategies and methodologies to provide explicit and understandable explanations for the decision-making processes of AI systems. The chosen approach and the complexity of the discussed AI model determine how exactly XAI functions.
Model interpretation is a popular strategy in XAI, where approaches, including feature importance analysis, gradient-based methodologies, or sensitivity analysis, are utilized to comprehend the variables influencing the model’s judgments. Users learn more about the model’s behavior using the strategies, which assign relevance scores to features or pinpoint the aspects that influence the decision-making process most.
The creation of local explanations is another XAI strategy. Local explanation entails justifying specific forecasts or judgments made by the AI system. Pinpoint the specific components or pieces of information that contribute to a particular result using strategies, including rule-based explanations, example-based reasoning, or attention techniques. Users better comprehend the rationale behind each choice due to the regional justifications.
Global explanations in XAI aim to convey a comprehensive grasp of how the AI model functions. The methods examine the decision rules, linkages, and patterns the model has discovered from a wider angle. They consist of techniques that include decision trees, rule extraction, or visualizations that show the AI system’s high-level decision-making process.
Visualization tools in XAI are essential because they make complex data and model behavior more understandable and intuitive. The visualizations are heatmaps, charts, or graphs emphasizing key model components, connections, or decision-making processes.
XAI entails creating counterfactual explanations, which give alternative scenarios to show how altering specific inputs or attributes produce different outcomes. Another feature of XAI is uncertainty estimation, which measures the degree of assurance or probability distribution connected to the predictions made by the AI system.
How Can Explainable AI be Utilized for Healthcare?
Explainable AI (XAI) is utilized in the healthcare industry to improve decision-making, patient outcomes, and trust and transparency in AI-driven systems. Applications in healthcare, such as medical picture analysis, diagnosis, therapy prescription, and patient monitoring, all benefit from the rich insights and explanations that XAI approaches offer.
XAI assists radiologists and clinicians in understanding the characteristics and patterns that AI algorithms utilize to make diagnoses in medical image analysis. Healthcare professionals’ confidence in the precision and dependability of the AI model is increased by XAI’s capacity to validate and understand the results by explaining the AI system’s findings. The collaboration between AI and healthcare professionals results in more precise and effective diagnoses, lowering human error and enhancing patient outcomes.
XAI supports the rationale behind AI-generated treatment regimens or drug recommendations. The system facilitates shared decision-making with patients, supports healthcare providers’ understanding of the variables taken into account by the AI model, and guarantees that therapies align with clinical recommendations and each patient’s unique needs. Healthcare practitioners trust and defend AI-driven treatment suggestions due to the justifications supplied by XAI, which increases the adoption and acceptability of AI technology in clinical practice.
XAI helps with risk assessment and patient monitoring. Healthcare workers better grasp the underlying elements and characteristics that affect patient outcomes by using XAI to explain AI-generated risk scores or predictions of adverse events. Better patient risk classification, early intervention, and individualized care strategies are available. Healthcare practitioners explain and comprehend the rationale behind patient risk assessments because of the explanations offered by XAI. AI in healthcare promotes openness and trust in the decision-making process.
How Can Explainable AI be Utilized for Financial Services?
Explainable AI (XAI) is utilized in financial services to improve decision-making, better fraud detection, guarantee regulatory compliance, encourage openness and trust in AI-driven systems, and more. Financial institutions comprehend and confirm the reasoning behind AI-driven predictions, risk assessments, and fraud detection with the help of XAI approaches, which offer unambiguous explanations.
Financial institutions use XAI to spot suspicious activity and explain the factors in identifying fraudulent transactions. Investigators better grasp the patterns, characteristics, or abnormalities that led to the AI system’s fraud alerts due to the explanations provided by XAI. It helps to reduce false positives, increase the effectiveness of fraud protection initiatives, and confirm the validity of fraud detection results.
Another area where XAI is useful is regulatory compliance. Financial services are governed by a number of laws, including those about know-your-customer (KYC) and anti-money laundering (AML). Financial institutions justify and record reasons for particular decisions by using XAI, which offers explanations for AI-driven compliance decisions. It helps fulfill legal requirements, assures accountability, and makes audits and regulatory reporting easier.
XAI aids the processes of credit scoring and loan approval. Financial institutions better communicate to clients the rationale behind credit decisions by using XAI to explain the features and aspects the AI models consider when assessing credit. Individuals understand why they were accepted or refused loans, which fosters justice and builds trust between financial institutions and their clients.
XAI helps with investment advice and portfolio management. Financial experts and investors grasp the elements driving the recommendations due to XAI’s explanations for AI-driven investment predictions and strategies. AI in finance promotes informed decision-making, increases the transparency of investment decisions, and aids in matching investment strategies to client preferences and risk profiles.
How Can Explainable AI be Utilized for Retail Sector?
Explainable AI (XAI) is used in retail to improve customer experience, personalization, inventory management, and price decisions. Retailers are expected to comprehend and explain the thinking behind AI-driven recommendations, product suggestions, and pricing strategies with the help of XAI approaches.
Retailers benefit from XAI by better understanding the elements affecting AI-driven recommendations and suggestions in customer experience and personalization. Retailers confirm and decipher the logic behind personalized offers, product recommendations, and targeted marketing campaigns with the help of XAI by offering explanations. It increases customer trust and happiness by allowing them to understand why specific products or offers are given to them, resulting in a more engaging and personalized purchasing experience.
XAI helps retailers manage their inventories more effectively. Retailers better understand what drives demand for particular products by using XAI’s explanations for AI-generated demand forecasts. It allows businesses to decide on inventory levels, restocking plans, and supply chain management with knowledge, preventing stockouts or having too much inventory while ensuring the proper products are available when needed.
XAI assists with retail pricing choices. Retailers comprehend the logic behind dynamic pricing, promotional offers, and discounts by outlining the aspects AI models consider while developing pricing strategies. Retailers explain to customers and other stakeholders the reasons behind pricing decisions due to transparency, which promotes fair pricing practices and fosters customer trust.
XAI helps with fraud prevention and detection in the retail sector. Retailers better comprehend the characteristics and patterns that led to fraud warnings and suspicious activity detection using XAI’s explanations for the alerts. AI in retail allows them to verify and take the proper steps, lowering false positives and increasing the accuracy of efforts to detect fraud.
How Can Explainable AI be Utilized for Cyber Security?
Explainable AI (XAI) is utilized in cyber security to improve threat detection and incident response and give transparency and accountability in AI-driven security systems. Cybersecurity experts comprehend and verify the logic behind AI-generated alerts, predictions, and risk assessments with the help of XAI approaches, which offer unambiguous explanations for the outcomes.
XAI assists cybersecurity teams in comprehending the variables and signs that AI models employ to spot risks and assaults in threat detection. XAI allows analysts to evaluate and confirm the AI-generated alerts by offering explanations and assisting in the detection of false positives and false negatives. The accuracy and efficiency of threat detection systems are improved by transparency, allowing for quick reaction and mitigation.
The underlying causes and characteristics of an AI-generated incident or anomaly detection are explained by XAI, which assists with incident response. XAI assists cybersecurity experts in understanding the context and severity of identified incidents, supporting effective reaction and repair measures. It makes incident triage more effective, shortens response times, and lessens the effects of security breaches.
XAI helps with vulnerability management and risk assessment. XAI enables cybersecurity professionals to comprehend the foundation of risk assessments and prioritize remediation activities by outlining the parameters taken into account by AI models in risk score and vulnerability detection. Informed decision-making and resource allocation are achievable by transparently addressing the most severe security risks and vulnerabilities.
XAI helps with explainable threat intelligence by revealing the underlying linkages, patterns, and indicators that AI models employ to recognize new and existing threats. Cybersecurity experts are given the ability to interpret and authenticate threat intelligence outputs by XAI, which explains the rationale behind it. AI in cyber security empowers them to take preventative measures to safeguard their systems and networks.
What are Ethical Considerations in with AI?
Ethical considerations in AI encompass a range of essential factors that must be addressed when working with artificial intelligence technologies. Fairness and prejudice reduction in AI systems are two essential ethical considerations. Preventing unfair results and addressing prejudices related to protected characteristics, including race, gender, and age, are essential. Privacy and data protection are significant problems, as AI frequently relies on personal information. Upholding privacy rights requires taking strong security precautions, protecting sensitive data, and getting informed consent.
Transparency and explainability are critical ethical considerations because AI algorithms are sophisticated and opaque. They build confidence, improve accountability, and allow people to comprehend how AI systems arrive at their decisions when decision-making procedures are transparent and explained. Additional ethical issues include responsibility and blame because it’s critical to create frameworks for assigning blame when AI systems go wrong or hurt people. Providing human autonomy and control over AI systems is essential, underscoring the idea that AI must support rather than replace human decision-making.
Ethics must consider how AI affects society, including job losses and economic repercussions. Developing measures for job transition, retraining, and reskilling programs is essential to ensure a fair opportunity. AI technologies are used for good and bad, raising ethical issues that call for rules and governance systems to stop improper use. Another factor to consider is algorithmic accountability, which involves routinely auditing and assessing AI systems to guarantee fairness, accuracy, and compliance with ethical norms.
Experts in ethics, law, technology, and various stakeholders must work together across disciplines to address ethical issues. Society benefits from AI while reducing risks and guaranteeing AI technology’s ethical and responsible use. Exploring Ethical Considerations in AI builds AI systems with ethical principles in mind, encouraging transparency, protecting privacy, assuring fairness, and fostering accountability and human control.
Does Explainable AI and AI not the Same?
Yes, Explainable AI (XAI) and AI are not the same. The term “AI” stands for the broader field of creating intelligent machines that are capable of carrying out tasks that normally need human intelligence. It includes a broad range of methods and algorithms that allow computers to analyze data, reason, and make choices. XAI is a branch of AI that specializes in creating approaches and strategies to make AI systems easier to understand, interpret, and justify.
XAI tackles the problem of comprehending and elucidating the decisions made by AI algorithms, while AI attempts to develop intelligent systems that carry out activities independently. Humans understand the decision-making process and the reasons impacting those conclusions due to using XAI approaches, which are designed to provide clear and intelligible explanations for AI-driven results.
The “black box” issue with AI, where it is challenging to understand or interpret the inner workings of sophisticated algorithms, is addressed by XAI. AI systems become more transparent, interpretable, and accountable by adopting XAI approaches. XAI facilitates regulatory compliance, enables users to validate AI outputs, fosters trust, and assists in locating biases or flaws in Artificial Intelligence models.
What is the Difference Between Explainable AI from Responsible AI?
Responsible AI and Explainable AI (XAI) are related ideas but have different objectives. Explainable AI focuses on the transparency and interpretability of AI systems to provide concise justifications for the choices made by AI algorithms. It tackles the “black box” issue by allowing humans to comprehend the rationale and elements that influence AI-driven outcomes. XAI’s benefits are increased trust, easier regulatory compliance, and assistance in spotting biases or flaws in AI models.
The Responsible AI movement has a broader viewpoint and considers various ethical issues while developing and using AI technologies. It focuses on the ethical and responsible usage of AI systems, considering issues including justice, privacy, responsibility, bias reduction, and the social implications of AI.
Responsible AI entails taking into account the risks and effects of AI technologies and making sure that AI is created and used in a way that is consistent with moral standards and societal norms. It entails dealing with concerns, including algorithmic bias, privacy protection, human control, autonomy, accountability for AI judgments, and the effect of AI on employment and socioeconomic dynamics.
XAI is a component of Responsible AI. The system examines artificial intelligence technology’s more significant ramifications and societal impact, focusing on guaranteeing fairness, transparency, accountability, privacy, and human well-being. Responsihttps://www.holisticseo.digital/ai/machine-learning/responsibleble AI extends beyond interpretability to encompass a holistic approach to the ethical and responsible development, deployment, and use of AI systems.
- 48 Online Shopping and Consumer Behavior Statistics, Facts and Trends - August 22, 2023
- B2B Marketing Statistics - August 22, 2023
- 38 Podcast Statistics, Facts, and Trends - August 22, 2023